A Comprehensive Review of Econometrics Trends and Techniques
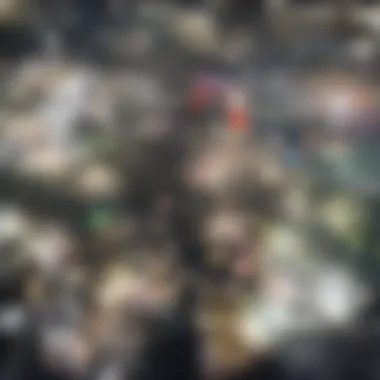
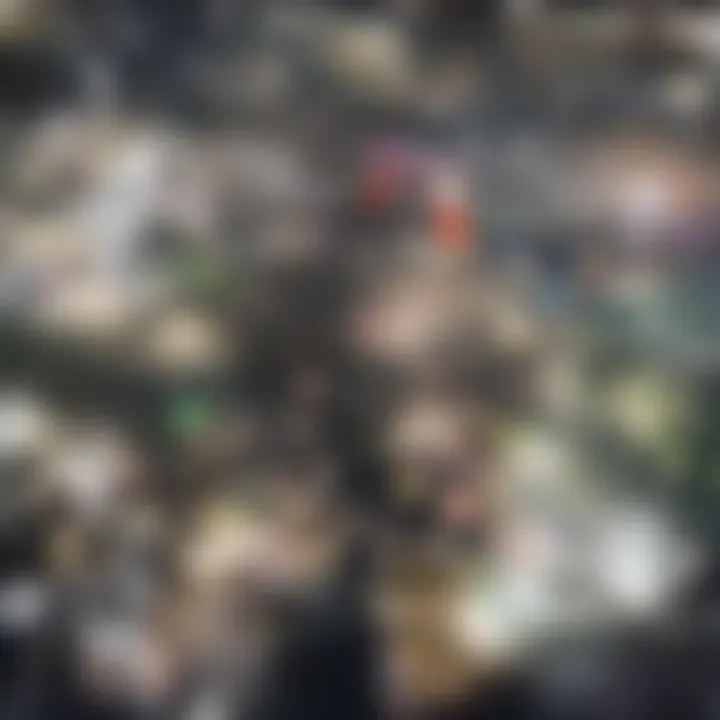
Research Context
Econometrics is a domain that sits at the crossroads of economics and statistics. It examines data to understand economic relationships, testing theories and quantifying economic phenomena. Given the intricate nature of economies, econometric analysis allows researchers to derive insights that are not readily apparent. This area has evolved significantly with advancements in computational power and data availability.
Background Information
The origins of econometrics can be traced back to the early 20th century, with the aim to establish a more rigorous approach to economic analysis. At its core, econometrics uses statistical methods to give empirical content to economic theories. This transformation is marked by key developments in methodologies, moving from simpler linear models towards more complex nonlinear frameworks. The rapid growth of data science and machine learning is also influencing the direction econometrics is heading.
Importance of the Study
Understanding econometrics is crucial for several reasons. Firstly, it provides fundamental tools for policymakers. By analyzing the impact of decisions, econometricians can model potential outcomes, enabling informed decision-making. Additionally, the techniques introduced in econometrics have applications beyond economics, influencing fields like finance, health, and public policy.
Moreover, this study highlights significant methodologies and their applications in various domains. By understanding current trends, practitioners can leverage innovative techniques for better economic modeling. Equally, discussing the future directions offers insights into how emerging technologies like big data and AI can redefine economic analysis.
Discussion
Interpretation of Results
Results from econometric analyses often yield valuable insights into relationships that traditional economic models might overlook. For example, regression analysis can elucidate factors influencing inflation or employment rates, allowing economists to draw connections that inform broader economic theories.
The use of advanced techniques such as time series analysis or causal inference adds layers of depth to these results. This ability to dissect past economic behaviors and predict future trends underscores the relevance of econometric methodologies in today's world.
Comparison with Previous Research
Comparing recent studies in econometrics with earlier works reveals significant shifts in both methods and focus areas. Earlier research often relied on basic models that assumed linear relationships. Today's research emphasizes complexity and the integration of various data sources.
For instance, modern studies often incorporate machine learning algorithms. These methods can enhance predictive accuracy and unveil patterns that simpler models might miss. By juxtaposing historical approaches with contemporary ones, one can appreciate how far econometrics has come and its trajectory for future development.
"Econometrics utilizes data not merely to affirm existing theories, but to challenge, refine, and redefine our understanding of economic behavior."
In summary, the study of modern econometrics provides a framework that is not only applicable but essential across diverse fields. As the discipline continues to grow, so too does its influence in shaping economic understanding and policy.
Prelims to Econometrics
Econometrics is a vital area within economics that bridges empirical data and theoretical models. By systemically applying statistical principles to economic data, econometricians can derive insights that facilitate informed decision-making. This section emphasizes the importance of understanding the fundamentals of econometrics, as it lays the groundwork for comprehending more complex analytical methods and applications.
Definition and Scope
Econometrics can be defined as the application of statistical and mathematical theories to the study of economic data. It aims to quantify relationships within economic phenomena, producing models that are both predictive and explanatory. The scope of econometrics is significant; it spans numerous fields, including macroeconomics, microeconomics, and finance. Econometrics is essential for analyzing economic trends, testing hypotheses, and evaluating economic theories. The integration of data into this sphere enhances its relevance, allowing for more accurate assessments of real-world economic issues.
Historical Development
The roots of econometrics trace back to the early 20th century. The field began to formalize with the works of pioneers like Ragnar Frisch and Jan Tinbergen, who developed early models using statistical methods. During the 1960s, the field gained traction with advances in computer technology, allowing for more sophisticated methods and larger datasets. The integration of these tools paved the way for modern econometrics. Over time, the focus shifted from purely theoretical models to empirical validation, emphasizing real-world applications and data-driven conclusions. Today, econometrics is an established discipline, critically analyzing problems ranging from inflation to labor market dynamics.
Key Terminologies
To engage effectively with econometrics, familiarity with key terminologies is crucial. Some fundamental terms include:
- Regression Analysis: A statistical method used to understand the relationship between variables.
- Endogeneity: A situation in which an explanatory variable is correlated with the error term, leading to biases in estimators.
- Time Series Analysis: A technique that analyzes data points collected or recorded at specific time intervals.
- Panel Data: Data that involves observations over time for the same subjects, allowing for more intricate analyses.
Each of these terms serves as a building block for deeper understanding and application within econometric studies.
"Understanding the foundations of econometrics is critical for anyone looking to analyze or interpret economic data effectively."
Through defining the discipline, tracing its historical evolution, and elucidating key terms, this section frames econometrics as essential for interpreting economic phenomena.
It prepares the reader for more complex models and applications explored in subsequent sections of the article.
Core Econometric Models
Core econometric models are fundamental to understanding complex economic relationships. These models provide practitioners and researchers tools to analyze data effectively and draw insightful conclusions. Their significance in econometrics cannot be overstated; they serve as the backbone of empirical economic research. This section will explore three primary econometric models: Linear Regression Models, Generalized Method of Moments, and Instrumental Variable Techniques, highlighting their components, uses, and the considerations to keep in mind while applying them.
Linear Regression Models
Linear regression models are widely used in econometrics for estimating relationships between variables. These models assume a linear relationship between dependent and independent variables. Their simplicity allows for easy interpretation of results. By estimating coefficients, researchers can assess how changes in independent variables impact the dependent variable.
Some benefits of linear regression include:
- Ease of use: These models can be easily constructed and understood.
- Interpretability: The coefficients indicate the direction and strength of relationships.
- Foundation for more complex models: Linear regression serves as a basic building block for various advanced econometric techniques.
However, careful consideration must be given to the assumptions that underlie these models, such as linearity, independence, and homoscedasticity.
Generalized Method of Moments
The Generalized Method of Moments (GMM) is another pivotal econometric technique. It extends the ordinary least squares method, allowing for more flexibility in handling various statistical issues. GMM focuses on estimating parameters by matching sample moments to their theoretical counterparts.
Key features of GMM include:
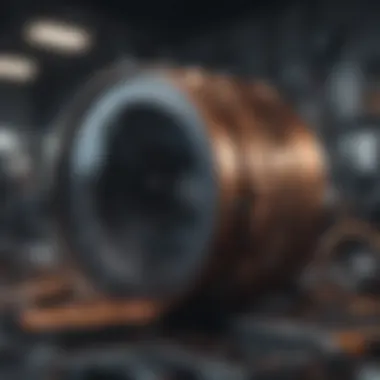
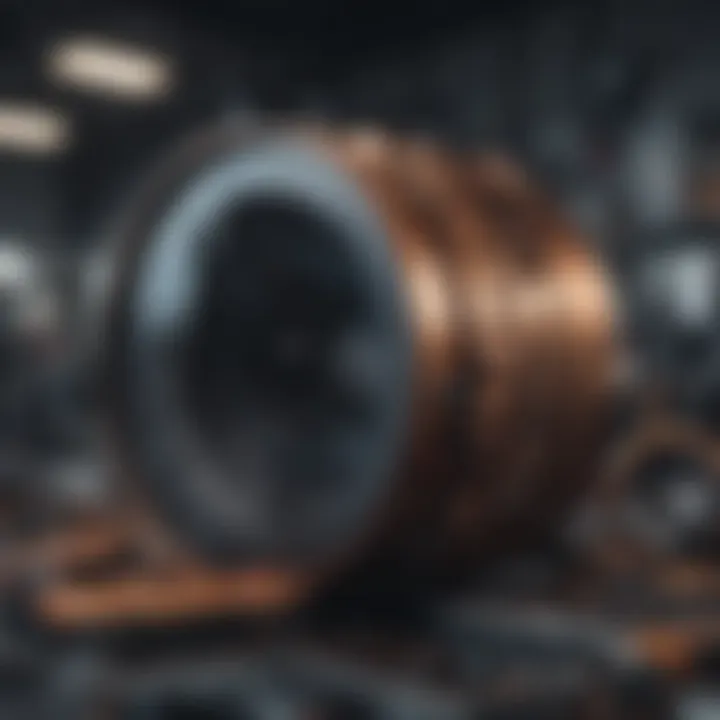
- Flexibility: GMM applies to more complex datasets and models, including those suffering from heteroskedasticity.
- Asymptotic efficiency: Under certain conditions, GMM estimators exhibit desirable properties.
- Zero moment conditions: This method can work with over-identifying restrictions, increasing robustness.
However, GMM may require robust instruments to ensure valid estimation.
Instrumental Variable Techniques
Instrumental Variable Techniques (IV) address the problem of endogeneity in econometric models. Endogeneity arises when an independent variable correlates with the error term, leading to biased results. IV methods introduce instruments—variables that are related to the endogenous predictors but uncorrelated with the error term—to obtain consistent estimates.
Benefits of using IV techniques include:
- Correction for bias: IV helps obtain unbiased estimators when direct variable relationships are problematic.
- Applications in various fields: This method is useful in fields such as health economics, education evaluation, and behavioral economics.
Maintaining the validity of the instrument is crucial. The instruments must be strong enough to provide reliable results.
Overall, core econometric models, like Linear Regression, Generalized Method of Moments, and Instrumental Variable Techniques, are essential in advancing the field of econometrics. They assist in making sense of precise economic questions and guide practical applications in policy and decision-making.
In summary, understanding these models enhances the ability to conduct robust economic analysis, which is crucial for students, researchers, and professionals in the field.
Evaluating Econometric Models
Evaluating econometric models is crucial in ensuring that the models provide reliable and accurate results. This evaluation process includes different techniques and considerations that assess how well a model fits the data it is supposed to represent. A robust evaluation helps in deriving meaningful interpretations and making informed decisions based on the findings.
Inadequate evaluations can mislead policymakers and researchers alike. Therefore, emphasizing the significance of this section in the review is essential.
Goodness of Fit Techniques
Goodness of fit techniques provide information on how well the selected econometric model describes the observed data. These techniques yield valuable metrics and indicators, which can be used to evaluate a model's predictive accuracy and validity.
Common methods include:
- R-squared: This coefficient indicates the proportion of the variance in the dependent variable that can be predicted from the independent variables. A higher value suggests a better fit.
- Adjusted R-squared: Unlike the standard R-squared, this number adjusts for the number of predictors in the model, which prevents misleadingly high values in models with many variables.
- Residual Analysis: By analyzing the residuals, one can assess the distribution and randomness of errors. A good model will show no patterns in the residuals.
- F-statistic: This statistic tests the null hypothesis that all regression coefficients are equal to zero, thus determining if the model is explanatory.
By employing a combination of these techniques, researchers can obtain a comprehensive picture of model performance.
Hypothesis Testing
Hypothesis testing in econometrics serves as a primary tool for validating models. It assesses the impact and significance of independent variables on the dependent variable. Generally, hypothesis tests can lead to conclusions about the relationships within the data.
The fundamental steps include:
- Formulating Hypotheses: Define the null hypothesis (H0) and the alternative hypothesis ().
- Choosing Significance Levels: Commonly, a 5% significance level is selected.
- Calculating Test Statistics: Depending on model type, tests like t-tests or z-tests are calculated.
- P-values: These values indicate the probability of observing the data under the null hypothesis. If the p-value is less than the chosen significance level, H0 can be rejected.
Employing robust hypothesis testing ensures that identified relationships within the model are statistically sound.
Model Selection Criteria
Model selection is an essential process in econometrics, involving the choice between competing models based on their performance and suitability to the data. Various criteria are employed to filter out models that do not meet empirical and theoretical standards.
Key criteria for model selection include:
- Akaike Information Criterion (AIC): This criterion estimates the relative quality of statistical models for a given set of data, where lower values indicate better fit.
- Bayesian Information Criterion (BIC): Similar to AIC, but introduces a penalty for the number of parameters, which is useful when dealing with a limited sample size.
- Cross-Validation: This technique involves partitioning data into subsets to validate model effectiveness, ensuring that the model’s findings generalize well to unseen data.
Effective model selection safeguards against overfitting and underfitting, which can compromise the validity of research conclusions.
Applications of Econometrics
Econometrics serves as a crucial framework for analyzing economic data and informing decisions in various fields. This section highlights the importance of applying econometric principles in practical scenarios, emphasizing how these techniques guide policy-making, enhance financial predictions, and inform health economics.
Public Policy Analysis
Public policy analysis relies extensively on econometric methods to evaluate the effectiveness of programs and policies. By employing regression analysis, researchers can ascertain the causal relationships between policies and their economic impacts.
- Impact Assessment: Econometrics assists in understanding whether a policy achieves its intended goals by measuring outcomes like employment rates or income distributions.
- Counterfactual Analysis: It allows analysts to create hypothetical scenarios about what would happen without a specific policy, differentiating actual outcomes from those that may have occurred under alternative regulations.
An example can be found in welfare programs, where econometric models can assess how changes in benefits influence poverty levels. As a result, these models provide valuable insights that shape governmental strategies to enhance societal welfare.
Financial Market Predictions
In finance, econometrics plays a pivotal role in predicting market trends and asset prices. Analysts utilize various models to forecast returns on investments and manage risks effectively.
- Time Series Analysis: This technique is vital for understanding patterns over time in financial data. It helps in forecasting stock prices or assessing the volatility of market trends.
- Risk Assessment Models: Econometric methods enable investors to quantify risks associated with different investment strategies, guiding capital allocation efficiently.
For instance, using econometric models, a hedge fund might predict the future movement of stock prices based on historical data, allowing for more informed trading decisions. Overall, the applications in financial markets enhance decision-making capabilities for both individual and institutional investors.
Health Economics
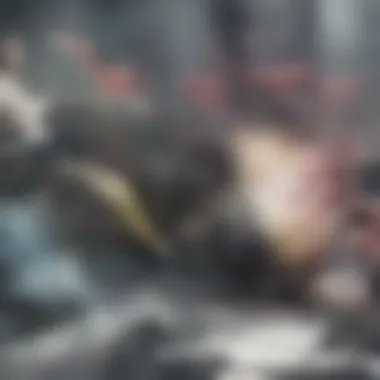
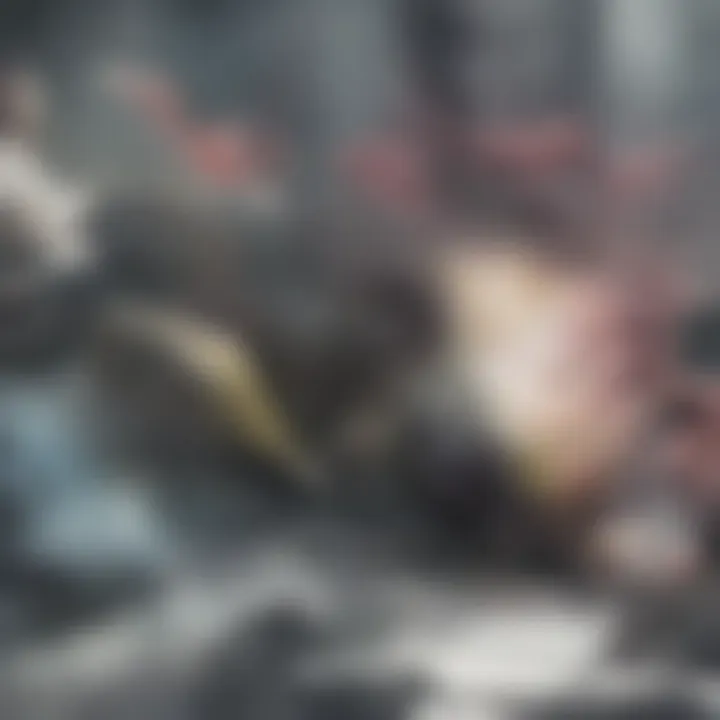
Health economics increasingly employs econometric techniques to study the efficiency and effectiveness of health care systems. By analyzing data, researchers can evaluate the cost-effectiveness of medical treatments and interventions.
- Cost-Benefit Analysis: Econometrics aids in comparing the costs of healthcare interventions with their expected health outcomes, making it easier to allocate limited resources.
- Program Evaluation: Health programs' impacts can be assessed using econometric methods to determine if they improve patient outcomes or lower costs.
A concrete example is examining the effects of vaccination programs on population health metrics, such as disease incidence and healthcare spending. The insights gained can directly influence health policy, ensuring that resources are used optimally.
Econometrics not only provides analytical tools but also shapes real-world applications, influencing policies and strategies across sectors.
Challenges in Modern Econometrics
Understanding the challenges in modern econometrics is crucial for anyone engaged in economic analysis. The field is evolving rapidly, and professionals must navigate various hurdles to produce robust and reliable models. These challenges not only affect the quality of results but also their utility in inform policy-making and business decisions. Each challenge discussed here offers significant insights into the complexities of econometric modeling.
Data Limitations
Data limitations present one of the foremost challenges in modern econometrics. Data quality and availability can significantly impact the outcomes of econometric analyses. Often, datasets may be incomplete, biased, or outdated. This issue is particularly acute in emerging economies where comprehensive data collection infrastructure may not be in place. The problem of missing data can lead to erroneous conclusions that affect economic policy.
Furthermore, various forms of data, such as big data, can introduce additional complications like noise or irrelevant information. It's essential to utilize methods like imputation or robust estimation techniques to address these limitations effectively. However, these solutions are not always feasible or may introduce new biases if not handled carefully.
Model Specification Issues
Model specification plays a pivotal role in econometrics. Choosing the correct model is critical to accurately representing economic theories or real-world phenomena. Issues arise when a model fails to include relevant variables or mistakenly includes unnecessary ones. These mis-specifications can skew results, leading to inaccurate predictions and potentially flawed policy recommendations.
Commom mistakes include ignoring non-linear relationships or neglecting interactions between variables. For example, a linear regression model might fail to capture the complexities of economic behavior if the relationship is inherently non-linear. Researchers must engage in rigorous diagnostic testing to ensure their model specifications are appropriate. This process often requires a deep understanding of both the economics at play and the technical aspects of model building.
Overfitting and Underfitting
Overfitting and underfitting are two complex issues that arise when fitting econometric models. Overfitting occurs when a model is too complex, capturing noise instead of the underlying pattern. This results in a model that performs well on training data but poorly on unseen data. Conversely, underfitting happens when a model is too simple to capture the underlying trend, often leading to significant predictive errors.
To mitigate these issues, researchers need to apply strategies like cross-validation or regularization techniques. These methods help select a model that balances complexity and generalizability, ensuring that it accurately predicts outcomes in real-world scenarios. Maintaining this balance is vital as the right choice can significantly influence economic analysis and policymaking.
"In econometrics, the path to accurate model predictions requires navigating through a landscape of data challenges, specification pitfalls, and model fitting issues."
By acknowledging and addressing these challenges, both researchers and policymakers can enhance the relevance and accuracy of econometric analyses.
Recent Trends in Econometric Research
The landscape of econometric research is evolving rapidly, influenced by advancements in technology and analytical techniques. This section delves into three pivotal trends that are reshaping the field. Understanding these trends is crucial for students, researchers, educators, and professionals who aim to integrate modern methodologies into their work, enhancing their understanding of economic phenomena.
Machine Learning Integration
The integration of machine learning into econometrics represents a significant shift in analysis methodologies. Machine learning algorithms, such as decision trees, random forests, and neural networks, provide powerful tools for dealing with non-linear relationships and complex interactions among variables. Unlike traditional econometric models that often assume linear relationships, machine learning techniques can adapt to more intricate patterns in data.
"Machine learning methods can unveil insights that classical models may not detect due to their rigid assumptions."
Moreover, the predictive power of machine learning models has been shown to outperform traditional econometric techniques in various applications, from financial forecasting to policy evaluation. The challenge, however, lies in the interpretability of these models. Econometricians must bridge the gap between sophisticated machine learning techniques and the necessity for clear explanations of results.
Big Data Applications
The incorporation of big data into econometric research has opened new avenues for empirical analysis. With the exponential growth of data availability, researchers can now leverage vast datasets that encompass diverse variables. This advancement allows for greater accuracy in modeling and insights into previously obscure relationships.
The use of big data enables:
- Enhanced predictive accuracy: More extensive datasets can lead to improved predictions as they capture a broader range of economic scenarios.
- Real-time analysis: Big data applications allow for a faster response to economic events, making it possible to adjust policies on the fly.
- Complex problem solving: Researchers can analyze intricate issues like climate change impacts on economic growth using data that includes weather patterns and socio-economic indicators.
However, working with big data also introduces challenges such as the need for robust data management and analytical skills. Additionally, concerns regarding data privacy remain a critical consideration.
Behavioral Econometrics
Behavioral econometrics combines insights from psychology with traditional econometric models to understand how human behavior influences economic outcomes. This trend recognizes that economic decisions are often not driven by rational calculations but by cognitive biases and heuristics.
Key points of this approach include:
- Richer models of human behavior: By incorporating psychological factors, these models can better predict spending habits, investment choices, and policy acceptance.
- Experimental methods: Conducting experiments allows researchers to observe actual behavior rather than relying solely on self-reported data, which can be unreliable.
- Policy design: Understanding behavioral tendencies enables policymakers to create more effective economic policies that align with how people truly behave rather than how they are expected to behave.
In summary, recent trends in econometric research reflect a shift towards incorporating innovative techniques and interdisciplinary approaches. These advancements not only enrich the field but also enhance its relevance in analyzing complex economic issues.
Future Directions in Econometrics
Future directions in econometrics represent a critical area of exploration for scholars and practitioners alike. As the discipline evolves, it is important to identify and analyze the emerging trends that will shape the future of econometric research. This section focuses on three key elements that are anticipated to drive change in the field: the influence of emerging technologies, the significance of cross-disciplinary approaches, and the implications for policy formulation.
Emerging Technologies
The integration of emerging technologies in econometrics is paving the way for more robust data analyses and model development. For instance, advancements in computational capabilities facilitate the handling of larger datasets, allowing for more refined empirical investigations. Technologies such as artificial intelligence and machine learning enhance the ability to uncover complex patterns within economic data. They allow econometricians to move beyond traditional models and explore innovative techniques that can yield greater accuracy.
Moreover, the application of natural language processing can revolutionize the way qualitative information is incorporated into econometric models. By analyzing unstructured data from sources like social media and news articles, researchers can gain insights into sentiment and public opinion, which are crucial for economic forecasting.
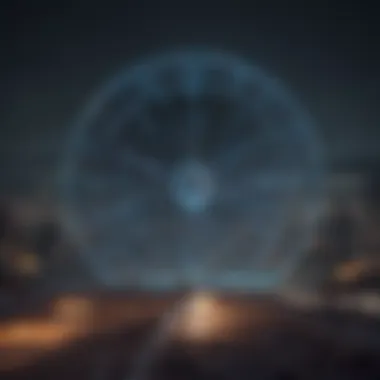
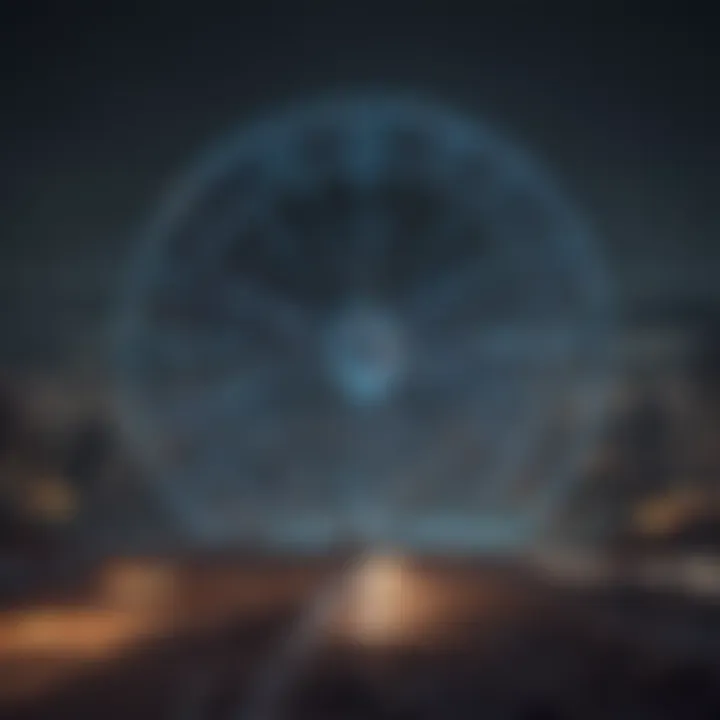
Cross-Disciplinary Approaches
The embrace of cross-disciplinary methodologies is another pivotal direction in the field of econometrics. Collaboration with domains such as psychology, data science, and environmental studies enriches econometric analysis. For example, behavioral econometrics leverages psychological insights to better understand economic decision-making processes. This integration helps in modeling human behavior with more realism.
Additionally, cross-disciplinary approaches provide a framework for tackling complex problems like climate change. Economic models can benefit from the quantitative approaches developed in ecology and geography. By combining expertise from different fields, econometricians can formulate comprehensive strategies that address multifaceted issues.
Policy Implications
Understanding future directions in econometrics also carries significant policy implications. The evolving nature of the field equips policymakers with better tools for decision-making. Accurate econometric models can inform fiscal policies, investment strategies, and trade agreements. As more refined statistical techniques emerge, enabling predictions with higher precision, the effectiveness of these policies can improve markedly.
Furthermore, the relationship between econometrics and public policy is increasingly pronounced. Insights derived from econometric analyses can illuminate the potential outcomes of policy changes, thus guiding legislators in their choices. In the age of data-driven decision-making, a rigorous econometric framework becomes indispensable for effective governance.
In summary, the future of econometrics is bright, powered by emerging technologies, cross-disciplinary collaborations, and enhanced policy insights. The continued adaptation and integration of these elements will drive the discipline forward, ensuring it remains relevant in addressing the challenges of the 21st century.
Case Studies in Econometrics
Case studies in econometrics serve as vital illustrations of theoretical principles and methodologies in action. They provide concrete examples that enhance the understanding of complex econometric techniques and their applications in real-world scenarios. By analyzing specific cases, researchers, students, and practitioners can gain insights into the mechanisms that drive economic phenomena. This section will explore three distinct case studies, each focusing on different aspects of econometric analysis. The benefits of case studies include:
- Practical applications of theoretical concepts
- Insights into the effectiveness of various econometric techniques
- An understanding of the challenges and limitations encountered in research
The analysis of these case studies also reflects the diversity of the field and its relevance across different socio-economic contexts.
Case Study One: Economic Growth
This case study focuses on the relationship between education and economic growth in developing countries. It uses panel data from multiple countries over several decades. By applying a linear regression model, researchers assess how educational attainment influences GDP growth rates. The findings show a strong positive correlation, indicating that higher education levels contribute significantly to economic development.
Some key considerations in this study include:
- Choice of variables: Other factors such as healthcare and infrastructure are also considered.
- Data limitations: Access to reliable data in developing countries can be challenging.
- Policy implications: The results underscore the importance of investing in education as a means to spur economic growth.
Case Study Two: Labor Market Dynamics
This case study examines the effects of minimum wage laws on employment levels in the fast-food sector. Utilizing a difference-in-differences approach helps to analyze the economic conditions of regions before and after the implementation of minimum wage increases. The analysis highlights varied impacts on employment, with some regions experiencing job losses while others saw job gains.
Important aspects include:
- Heterogeneity in responses: Different regions react differently to minimum wage changes.
- Long-term effects: The immediate impact can differ from long-term job market adjustments.
- Labor economics theories: The study engages with various labor market theories to explain findings.
Case Study Three: Environmental Economics
This case study assesses the effectiveness of carbon pricing mechanisms in reducing greenhouse gas emissions. It employs a panel data analysis spanning several countries with and without carbon pricing policies. Findings indicate that countries with carbon pricing show a more pronounced reduction in emissions compared to those without such policies.
Notable factors to consider include:
- Externalities: The case highlights the need to account for negative externalities in economic models.
- Economic efficiency: Carbon pricing mechanisms can create incentives for cleaner technologies.
- Policy recommendations: The results support the implementation of carbon pricing as an effective policy tool to combat climate change.
By examining these varied case studies, readers can appreciate how econometric methods are applied to address real-world issues, shaping not just academic discourse but also practical economic policy.
The End
The conclusion of this article serves a pivotal role in synthesizing the comprehensive review of econometrics that has been explored. Here, the significance of econometrics is underscored through its multifaceted applications across various domains, including public policy, financial markets, and healthcare. Econometrics equips researchers and policymakers with the tools necessary to analyze and interpret economic data, thereby facilitating informed decision-making.
Summary of Key Insights
In the preceding sections, numerous insights have been detailed:
- Core Models: Econometrics employs key models such as linear regression and instrumental variable techniques, which are critical for analyzing relationships between economic variables.
- Evaluation Techniques: Goodness of fit tests, hypothesis testing, and model selection criteria are essential for establishing the validity and robustness of econometric models.
- Emerging Trends: The integration of machine learning and big data signifies a shift toward more sophisticated analytical approaches, augmenting traditional econometric methods.
- Future Directions: Given the rapid evolution of technology, cross-disciplinary approaches will likely enhance the applicability of econometric analysis, offering new avenues for research and policy development.
This summary encapsulates the broader discussions within the article, highlighting how econometrics remains a relevant field capable of adapting to contemporary challenges.
Final Thoughts
Econometrics stands as an indispensable framework within the broader discipline of economics. As we face new economic uncertainties and complexities, the rigor of econometric analysis will be vital. The ongoing development of methodologies and the incorporation of novel data technology promise to enhance the functionality and reach of this discipline. The future of econometrics will not solely rest on refining existing techniques, but it will also profoundly influence policymaking and the understanding of economic phenomena.
Importance of Accurate Referencing
Accurate references ensure that readers can trace the origin of information. This process of tracing allows for verification and fosters trust in the findings discussed. In econometrics, where data is often complex and multifaceted, having solid references helps to anchor theoretical models in proven research. Furthermore, it highlights the interconnections within the field, showcasing how various studies build upon one another.
Benefits of Comprehensive References
The use of comprehensive references adds value to an article significantly. Here are some key benefits:
- Foundation for Further Research: References invite readers to explore deeper, enabling them to pursue topics that pique their interest.
- Support for Methodologies: Citing established methodologies reassures readers about the validity of the approaches used in econometric analysis.
- Clarification of Concepts: References can clarify ambiguous terminologies, making complex concepts more accessible.
Considerations for Incorporating References
However, there are important considerations to keep in mind while citing references:
- Relevance: Ensure that referenced works are directly applicable to the topic being discussed, enhancing the article's focus.
- Diversity of Sources: A well-rounded reference list should include a mix of academic journals, books, and credible online resources such as Wikipedia and Britannica.
- Up-to-date Literature: Given the evolving nature of econometrics, including recent publications can provide insight into current trends and methodologies.
"References not only substantiate the content but also enrich the reader's journey through the subject matter."