A Comprehensive Overview of Seeman Analysis Techniques
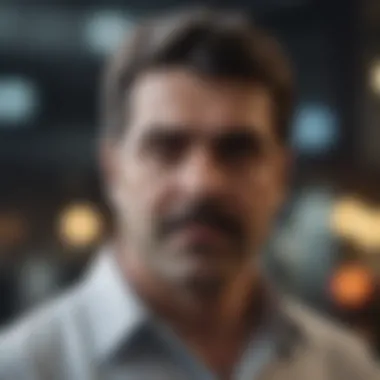
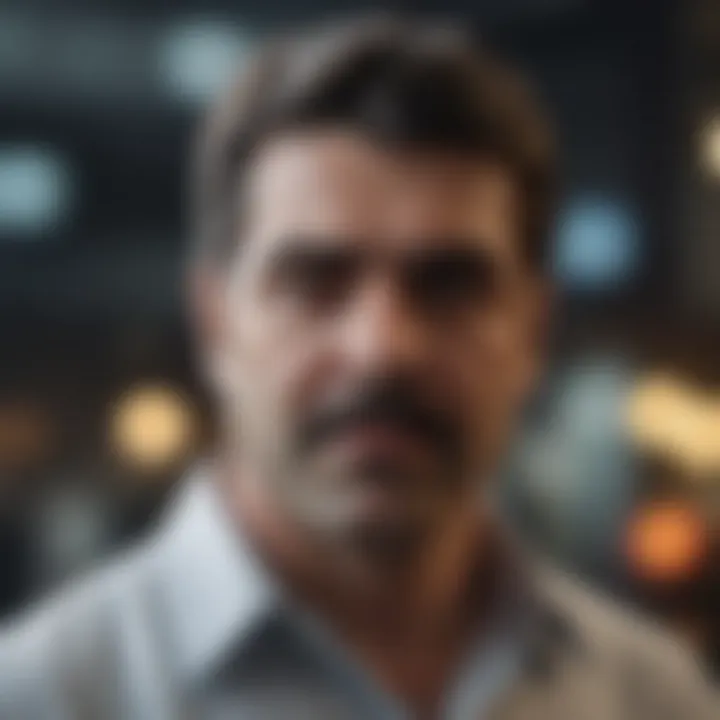
Intro
Seeman analysis is a methodological approach that stands out in the analysis of complex data sets across various scientific fields. Its significance is not merely confined to a single discipline; rather, it resonates throughout several domains, including sociology, psychology, economics, and natural sciences. This article systematically unpacks the main features of Seeman analysis, delving into its techniques, applications, and insights, serving as a resource for students, researchers, educators, and professionals alike.
In this discourse, a comprehensive understanding of Seeman analysis will be laid out, focusing on its foundational concepts, typologies, and practical applications. The relevance of this analysis in contemporary research is also examined, emphasizing its implications for effective data interpretation and sound decision-making. By navigating through this content, the reader will be equipped with the knowledge necessary to effectively leverage Seeman analysis in their work.
Research Context
Understanding the broader backdrop of Seeman analysis is crucial for appreciating its value in research. This section delves into the historical and contextual factors that underpin the methodology.
Background Information
Seeman analysis emerged as a response to the growing need for structured approaches to understand multifaceted data. Named after the influential sociologist, it incorporates various statistical and analytical techniques to dissect intricate relationships and patterns. The genesis of this analysis roots back to the exploration of social structures and individual behaviors, leading to its application in diverse research scenarios today.
Importance of the Study
The study of Seeman analysis is vital for multiple reasons:
- It enriches the methodological repertoire available to researchers.
- It enhances the precision of data analysis in social sciences and beyond.
- It accommodates interdisciplinary approaches, making it applicable in various fields.
- It supports informed decision-making by providing clear interpretations of data.
Discussion
The analysis of Seeman techniques offers insights that drive further research and practical applications. Engaging with the results of Seeman analysis allows for meaningful interpretations that influence future studies.
Interpretation of Results
Interpreting the results involves careful examination of the data through the lens of Seeman analysis. Researchers must identify key patterns and trends that emerge from the data. The effectiveness of Seeman analysis lies in its ability to reveal insights that may not be immediately apparent.
Comparison with Previous Research
Comparing Seeman analysis with other methodologies yields a deeper understanding of its strengths. Many traditional statistical methods overlook the complexity of data relationships, while Seeman analysis accounts for these intricate interdependencies. Previous studies utilizing alternative approaches often miss potential insights that Seeman analysis can uncover.
"Seeman analysis opens new avenues for understanding complex data and supports smarter decision-making processes."
Prologue to Seeman Analysis
Understanding Seeman analysis is critical in today's research landscape. This methodological approach plays a vital role across various disciplines, influencing how data is interpreted and decisions are made. The importance of this topic lies in its capacity to disentangle complex relationships within data sets.
Seeman analysis offers researchers sophisticated tools for both qualitative and quantitative examination. It provides insight into not only existing data but also guides future research directions based on evidence. As academic and professional realms increasingly rely on intricate data, comprehending Seeman analysis can enhance analytical skills significantly.
Defining Seeman Analysis
Seeman analysis refers to a methodological framework utilized for examining complex data sets. It involves both qualitative and quantitative approaches, allowing researchers to derive meanings and patterns from the information they analyze. With its roots in empirical research methods, Seeman analysis has emerged as an essential tool for making sense of multifaceted data in a systematic way.
At its core, Seeman analysis is characterized by its focus on logical reasoning and evidence-based conclusions. This analysis often includes various statistical techniques and qualitative assessments, enabling a comprehensive understanding of the subjects under study. The nuances in methods make it adaptable across numerous domains such as social sciences, health sciences, and engineering.
Historical Context
The evolution of Seeman analysis can be traced back to early research techniques that prioritized systematic observation and data organization. With the advent of advanced statistical methods in the 20th century, this analysis began to incorporate quantitative approaches, enhancing its applicability. Initial methodologies laid the groundwork for contemporary practices, reflecting a gradual transition to more complex analytical frameworks.
As interdisciplinary collaboration increased, the relevance of Seeman analysis expanded, becoming a robust tool for various research environments. Over the past few decades, an array of software tools developed, further streamlining the analysis process and enriching data interpretation.
Relevance Across Disciplines
Seeman analysis is not confined to a single field; rather, it demonstrates meta-disciplinarity, serving scholars and professionals in many areas, including:
- Social Sciences: Here, Seeman analysis aids in behavioral studies and policy evaluations, providing insights into societal patterns.
- Health Sciences: In medicine, it is crucial for epidemiological research and clinical trials, contributing to evidence-based practices.
- Engineering and Physical Sciences: It enhances quality control processes and predictive modeling applications.
"The versatility of Seeman analysis enables its application across diverse fields, underscoring its significance in interpreting complex phenomena."
By facilitating interdisciplinary research, Seeman analysis allows for a comprehensive exchange of ideas and methods, driving innovative solutions to pressing issues. Its adaptability assures that researchers can approach questions from various perspectives, maximizing the impact of their studies.
Methodological Framework
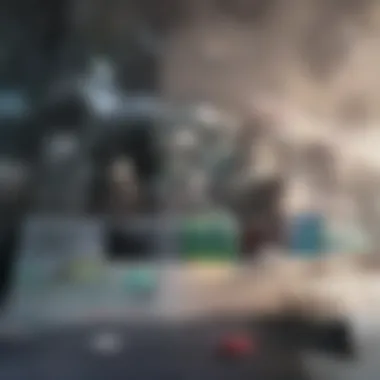
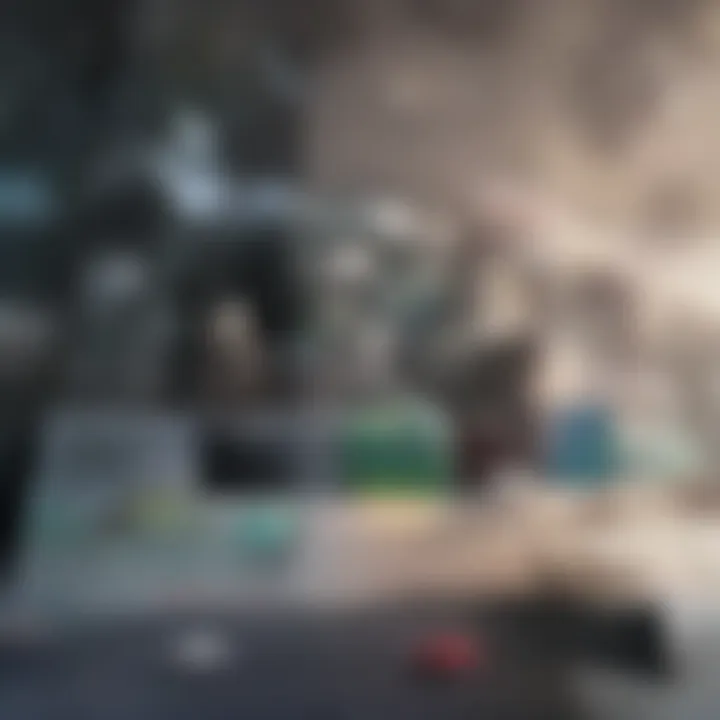
The methodological framework is fundamental in guiding the application of Seeman analysis. It establishes a structured approach for conducting analysis, ensuring the process remains systematic and replicable. This framework aids researchers in navigating the complexities of data analysis, promoting consistency in methods and interpretation. Key components of this framework include the selection of appropriate techniques, preparation of data, execution of analysis, and interpretation of results. Each step must be well-defined to ensure that conclusions drawn from the data are valid and meaningful.
Key Techniques
Data Collection Methods
Data collection methods are crucial for gathering accurate and relevant information necessary for Seeman analysis. These methods encompass a range of strategies such as surveys, interviews, and observational techniques. Choosing effective data collection methods can significantly enhance the quality of the analysis. A notable benefit of robust data collection is the reliability of the findings. Poorly conducted data collection may lead to skewed results and misinterpretations. Thus, ensuring thorough and precise collection processes underpins the effectiveness of the entire analysis task.
Among the unique features of data collection methods in the context of Seeman analysis is their adaptability. Depending on the research context, different methods can be utilized, allowing for a tailored approach. However, one disadvantage is that some methods can be resource-intensive, potentially limiting their practical application in certain scenarios.
Statistical Tools
Statistical tools play a pivotal role in facilitating the analysis of data. These tools enable the examination, interpretation, and presentation of data, making them indispensable in Seeman analysis. Statistics help in identifying patterns, testing hypotheses, and deriving insights that may not be immediately visible.
A significant characteristic of statistical tools is their capacity for complex calculations and modeling. The use of software such as SPSS or R is common in this realm. They are popular choices because they can handle large data sets efficiently, providing rigorous analytical outcomes. However, reliance on statistical tools can have drawbacks. If interpreted inappropriately, the results can lead to misconceptions about the data.
Steps in Conducting Seeman Analysis
Data Preparation
Data preparation is a critical phase in Seeman analysis. This step involves cleaning, organizing, and transforming data to make it suitable for analysis. Proper data preparation ensures that researchers can accurately assess the data's validity and reliability, which contributes significantly to the overall goal of deriving meaningful insights.
A key aspect of data preparation is the identification of outliers or errors within the data set. Addressing these issues early helps in enhancing the integrity of the analytical results. Although data preparation is vital, it can be time-consuming and require considerable effort, particularly with large and complex data sets.
Analysis Execution
Analysis execution involves implementing the chosen methods and statistical tools to explore the data. This step translates theoretical methodologies into practical applications, allowing researchers to process and analyze the collected data effectively. The execution phase is where the analysis comes to life, transforming raw information into actionable insights.
A fundamental characteristic of analysis execution is its iterative nature. Analysts may need to revisit previous steps based on findings, adjusting methods or approaches to enhance accuracy. This flexibility is beneficial, although it can also lead to extended timelines and increased complexity in the research process.
Result Interpretation
Result interpretation is the final step in the Seeman analysis process, and it holds considerable weight in deriving conclusions. This phase involves explaining the findings in context, linking them back to the research objectives, and determining their implications. Effective interpretation is essential for stakeholders to make informed decisions based on the analysis.
A noteworthy characteristic of result interpretation is its requirement for critical thinking and contextual understanding. Analysts must not only present data but also articulate what the findings mean for the intended audience. Potential challenges include bias or misinterpretation, which can distort the conclusions drawn from the analysis. By appreciating these nuances, researchers can deliver more reliable and impactful insights.
Types of Seeman Analysis
Understanding the different types of Seeman Analysis is crucial. Each type offers distinct methodologies and perspectives, which serve various academic and practical purposes. This section addresses three main types: Qualitative, Quantitative, and Comparative Seeman Analysis. Each type has unique characteristics, application areas, and advantages that enhance the breadth of Seeman Analysis.
Qualitative Seeman Analysis
Characteristics
Qualitative Seeman Analysis focuses on non-numerical data. It examines verbal or textual information, aiming to uncover patterns and themes. One key characteristic is its emphasis on context, making it particularly valuable in understanding complex human behaviors. Qualitative analysis enables researchers to capture nuances that quantitative methods might overlook.
Its benefit lies in providing deep insights that are often not available through numeric data alone. This method allows for a rich exploration of subjective experiences, offering a qualitative depth that is essential in social sciences.
Applications
Qualitative Seeman Analysis is widely used in various fields. It finds applications in behavioral studies, exploring how individuals or groups think and act. Researchers can conduct in-depth interviews or focus groups to gather rich data. Another important application is in policy evaluation, where it helps to assess the qualitative impact of policies on different populations.
The unique feature of this application is its ability to address the why behind trends, not just the what. However, a limitation could be the potential for researcher bias in interpretation.
Quantitative Seeman Analysis
Characteristics
Quantitative Seeman Analysis involves numerical data. It aims to quantify and analyze data trends statistically. A prominent characteristic of this type is the ability to generalize findings. It helps researchers make predictions about broader populations based on sample data.
This methodology is beneficial because it provides a robust framework for statistical analysis. Researchers utilize various statistical tools to derive meaningful insights from numerical data, which can lead to sound conclusions drawn from large datasets.
Applications
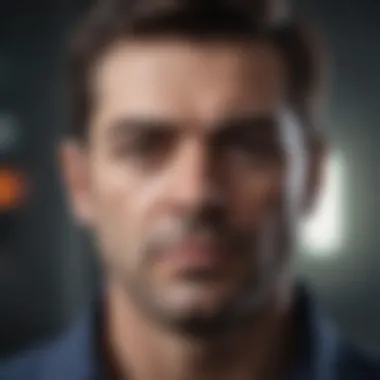
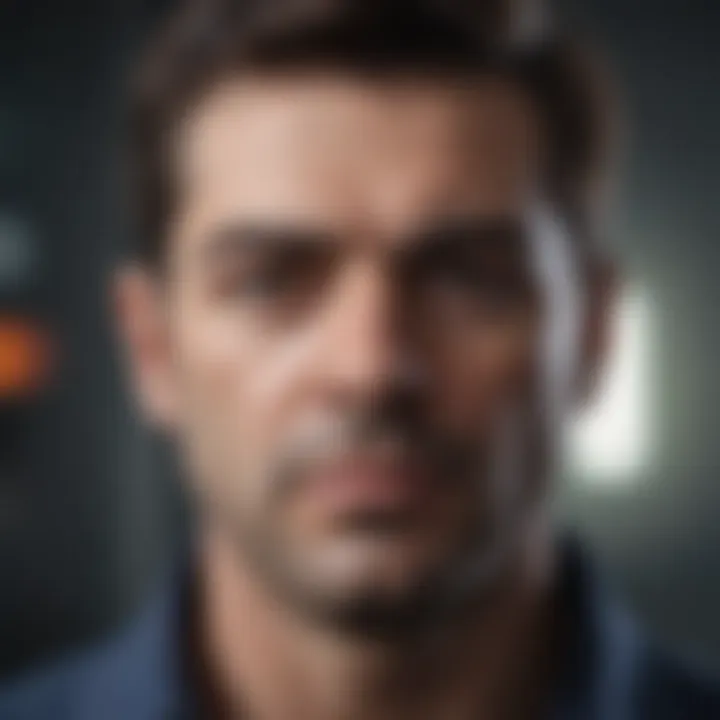
Applications of Quantitative Seeman Analysis extend across disciplines. In health sciences, it is particularly effective in epidemiological research, allowing for the study of disease patterns across populations. Clinical trials also benefit from quantitative methods, providing measurable outcomes that are critical for evaluating treatment effectiveness.
The strength of this type lies in its capacity for reproducibility and accuracy, as it relies on quantifiable metrics. However, it may fail to capture the depth of information that qualitative analyses provide.
Comparative Seeman Analysis
Strategies
Comparative Seeman Analysis seeks to evaluate two or more datasets or scenarios. This type of analysis is pivotal in identifying differences and similarities across analyses. A key strategy involves establishing a framework for comparison that includes defined criteria, ensuring a systematic approach.
This method is popular because it enhances understanding by contextualizing results from different studies. It allows researchers to visualize patterns or discrepancies that could influence decision-making processes.
Outcomes
The outcomes of Comparative Seeman Analysis can be profound. By evaluating various approaches or datasets, researchers can derive conclusively about effectiveness, efficiency, and applicability in real-world situations. One notable characteristic of the outcomes is their reliance on evidence-based assessments that guide future research and application strategies.
While widely beneficial, the comparative methodology may require extensive data management and resources, which can present challenges. However, the insights gained often justify the effort.
Applications of Seeman Analysis
Seeman analysis plays a crucial role in various fields, where it provides insights that inform both research and practical applications. Its methods facilitate a better understanding of complex datasets, enabling researchers to draw meaningful conclusions. The applications are numerous and extend across disciplines, with benefits that enhance decision-making and contribute to knowledge advancement. In this section, we shall explore how Seeman analysis finds its place in different areas, emphasizing its significance in these domains.
In the Social Sciences
Behavioral Studies
Behavioral studies rely on Seeman analysis to examine patterns of human behavior. This approach allows for the exploration of underlying motivations, attitudes, and influences on behavior. One of the key characteristics of behavioral studies is the integration of qualitative and quantitative data. This blend provides a more nuanced understanding of behavioral phenomena, making it a popular choice for researchers.
A unique feature of behavioral studies is their capability to analyze large datasets using various statistical tools. This characteristic enhances the robustness of findings but may also introduce challenges related to data quality. Researchers must ensure that their data sources are reliable to draw valid conclusions.
Policy Evaluation
In the realm of policy evaluation, Seeman analysis serves to assess the impact and effectiveness of policies. Policymakers benefit from this analytical approach as it reveals how policies affect populations and highlight areas that require adjustment. A crucial aspect of policy evaluation is its focus on evidence-based outcomes, making it a favorable choice for social scientists.
One unique feature of policy evaluation is the ability to use real-world data to measure success indicators. However, challenges may arise in interpreting data accurately. Contextual factors often influence policy impacts, and researchers must navigate these complexities to provide actionable insights.
In Health Sciences
Epidemiological Research
Epidemiological research utilizes Seeman analysis to identify relationships between health outcomes and various risk factors. This method is essential for understanding disease patterns and informing public health initiatives. A significant characteristic of epidemiological research is its reliance on large sample sizes, which enhances the statistical power of the findings.
The unique aspect of this type of research lies in the ability to link environmental and genetic factors to health outcomes. However, epidemiological studies can face challenges such as confounding variables that may skew results. Researchers need to carefully design studies to mitigate these issues and ensure accurate interpretations.
Clinical Trials
Clinical trials are another application area where Seeman analysis is invaluable. They involve evaluating the effectiveness of medical interventions under controlled conditions. A key characteristic of clinical trials is their structured approach, involving clearly defined protocols and endpoints. This makes them a beneficial choice for researchers seeking to test hypotheses systematically.
The unique feature of clinical trials is their focus on direct patient outcomes, which can drive advancements in medical science. However, ethical considerations related to participant welfare can pose challenges. Balancing thorough analysis while maintaining ethical standards is critical for successful clinical research.
In Engineering and Physical Sciences
Quality Control
In engineering and the physical sciences, Seeman analysis contributes significantly to quality control processes. This application ensures that products meet established standards and specifications. A fundamental characteristic of quality control is its systematic approach to data analysis, allowing for continuous improvement in manufacturing processes.
A unique feature of quality control is the real-time feedback mechanism it provides. This allows engineers to identify defects early in the production process. However, challenges can arise from the need for extensive training on analytical tools. Maintaining consistent quality necessitates ongoing education for personnel.
Predictive Modelling
Predictive modelling applies Seeman analysis to forecast outcomes based on historical data. It is a vital tool in both engineering and physical sciences. The main characteristic of predictive modelling is its reliance on complex algorithms to make educated predictions. This makes it a favored option for researchers in these fields.
A unique aspect of predictive modelling is its ability to simulate potential future scenarios. However, the effectiveness of these models can depend heavily on the quality and quantity of input data. Researchers must be diligent in data selection to ensure valid predictions.
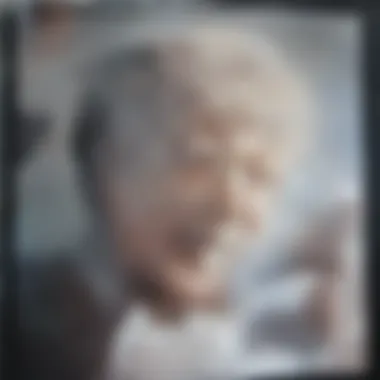
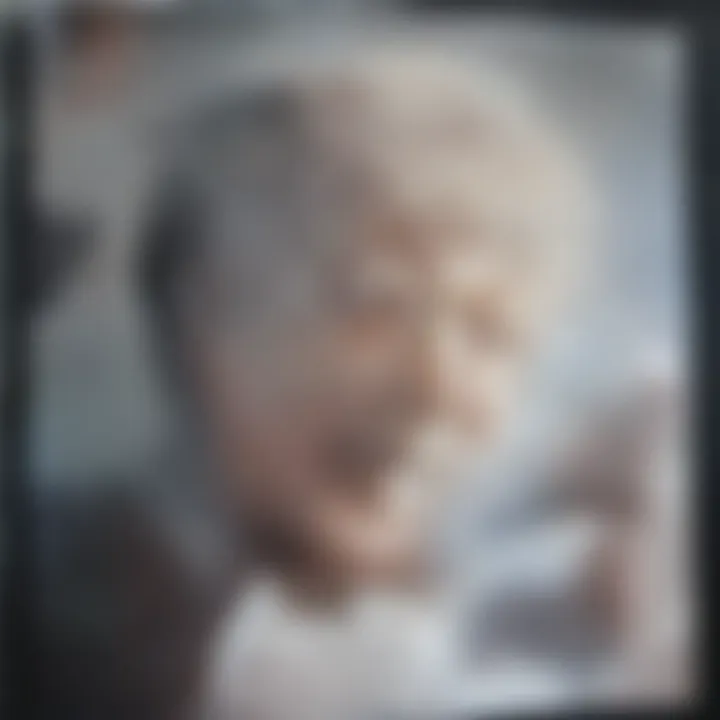
In summary, the applications of Seeman analysis span multiple disciplines, each leveraging its techniques to uncover crucial insights and improve outcomes. By understanding these applications, researchers can better utilize Seeman analysis for their specific fields.
Challenges in Seeman Analysis
The realm of Seeman analysis, despite its extensive applications and benefits, is fraught with various challenges that can undermine the reliability of outcomes. Addressing these challenges is not merely optional but essential for enhancing the robustness of the analysis. Understanding the implications of data quality issues, interpretation difficulties, and resource constraints provides a roadmap to navigate these hurdles effectively. By recognizing these obstacles, researchers can mitigate risks associated with incorrect conclusions, fostering credibility in their findings.
Data Quality Issues
Data quality stands as a cornerstone of effective Seeman analysis. Flaws in the data can lead to significant biases in results. Common problems include missing values, anomalies, and inconsistencies in data collection processes. Poorly collected data may not accurately represent reality, which can distort the conclusions drawn from the analysis. Moreover, the choice of data collection methods impacts quality significantly. For instance, survey-based data may suffer from respondent bias or misinterpretation of questions.
To combat these issues, it is critical to implement rigorous data management practices. This includes:
- Standardized data collection protocols. Establishing clear guidelines helps in maintaining consistency.
- Regular data audits. Periodic evaluations assist in identifying and correcting errors in datasets.
- Utilizing technology solutions. Software tools can help validate and clean data before analysis.
"Good data is foundational. Ensuring quality can mean the difference between insight and confusion."
Interpretation Difficulties
The interpretation of data results is another area where challenges often arise. Even with high-quality data, the analysis may yield misleading conclusions if interpreted improperly. This reflects a complex interplay between statistical skills, domain knowledge, and the analytical techniques employed. For instance, failing to account for confounding variables can lead to erroneous inferences about causality.
Moreover, the complexity of the data may require sophisticated statistical knowledge that not all practitioners possess. Misinterpretations can propagate throughout the research process, leading to flawed recommendations or policy decisions.
Mitigating interpretation difficulties involves:
- Training in statistical analysis. Enhancing skills in data interpretation can reduce errors.
- Peer reviews. Sharing findings with colleagues can provide alternative perspectives that illuminate weaknesses in the analysis.
- Clear communication of results. Utilizing accessible language and visualizations can help engage a broader audience, aiding in the understanding of complex data.
Resource Constraints
Finally, resource constraints can severely impact Seeman analysis. This includes limitations in time, funding, and access to analytical tools. Many researchers work within tight budgets, which can restrict the methods chosen for data collection and analysis. As a result, they may resort to less rigorous techniques that compromise the integrity of the research.
Addressing resource constraints requires innovative thinking. Strategies include:
- Leveraging open-source tools. Utilizing free statistical software can minimize costs.
- Collaborative projects. Partnering with other institutions may provide additional resources and expertise.
- Efficient project management. Prioritizing tasks and employing time management techniques can optimize limited time.
In sum, recognizing and addressing these challenges is crucial. By understanding the pitfalls associated with data quality, interpretation, and resource limitations, practitioners of Seeman analysis can enhance the validity and influence of their research outcomes.
Future Directions for Seeman Analysis
The future of Seeman analysis is rich with potential, offering avenues for innovation and application across various fields. Understanding the future directions of this analytical method is vital for several reasons. First, it allows researchers to stay abreast of technological advancements that can enhance the efficacy and accuracy of their analyses. Second, it serves to highlight integration possibilities with other methodologies. Lastly, the interdisciplinary nature of future Seeman analysis promise innovation by bringing diverse perspectives together.
Emerging Technologies
Emerging technologies are significantly reshaping the landscape of Seeman analysis. Advanced data processing tools such as machine learning algorithms and artificial intelligence can handle vast datasets with greater efficiency. These tools facilitate more complex analyses while reducing human error. An example is using AI to detect patterns that traditional methods may overlook. Furthermore, cloud computing offers flexible and scalable solutions for data storage and processing. Researchers can, therefore, access powerful computational resources on demand.
"The integration of AI in Seeman analysis is not just a trend; it is a transformative leap that enhances data interpretation capabilities."
As these technologies evolve, they will likely enable deeper insights and broader applications. Researchers must remain adaptable and willing to explore these tools to maintain relevant in an increasingly competitive environment.
Integration with Other Analytical Methods
The integration of Seeman analysis with other analytical methods can substantially enhance research outcomes. These collaborations allow for a more comprehensive approach, combining qualitative, quantitative, and comparative techniques. For instance, merging Seeman analysis with traditional statistical methods can provide a richer context for understanding relationships within data.
Moreover, connected analytical frameworks, such as system dynamics and network analysis, could be employed alongside Seeman methodologies. This might lead to the development of hybrid models that offer nuanced insights into complex phenomena, ultimately advancing theoretical and practical understanding in multiple disciplines. Researchers are encouraged to actively seek partnerships with experts in other methodologies to enrich their analyses.
Potential for Interdisciplinary Research
Interdisciplinary research holds significant potential for advancing Seeman analysis. Combining knowledge from various fields can foster innovative approaches that improve analysis quality and applicability. For instance, collaboration between social sciences and health sciences can yield rich data on behavioral health issues, incorporating diverse perspectives that enhance understanding.
In addition, the cross-pollination of ideas can spark creativity and lead to the development of new models or frameworks tailored to address specific research questions. This approach challenges traditional boundaries between disciplines, encouraging researchers to explore problems from multiple angles.
Ultimately, investing in interdisciplinary research contributes not just to the advancement of Seeman analysis but also to the broader body of knowledge across various fields. Encouraging collaboration among different academic disciplines can lead to groundbreaking discoveries and methodologies.
End
The conclusion section of this article serves as a critical component in reinforcing the importance of Seeman Analysis within diverse fields of study. As we review the cumulative insights discussed throughout the article, the overarching value of Seeman Analysis emerges clearly. This method provides a structured approach to deciphering complex datasets, aiding researchers in drawing meaningful conclusions. Its application transcends disciplinary boundaries, impacting areas such as social sciences, health sciences, and engineering.
Summary of Key Points
This section revisited the essential elements covered in the article. Key points include:
- Definition and Historical Context: We clarified the origins of Seeman Analysis and its evolution over time.
- Methodological Framework: Various techniques and the necessary steps for conducting a Seeman Analysis were thoroughly explored.
- Types of Analysis: We discussed qualitative, quantitative, and comparative methods, outlining their respective characteristics and applications.
- Applications: The practical use of Seeman Analysis in social sciences, health sciences, and engineering highlights its versatility.
- Challenges: Addressing data quality, interpretation hurdles, and resource limitations is crucial for effective implementation.
- Future Directions: The integration of emerging technologies and interdisciplinary research potential signifies a progressive outlook for the discipline.