Mastering Data Analysis in Scientific Research
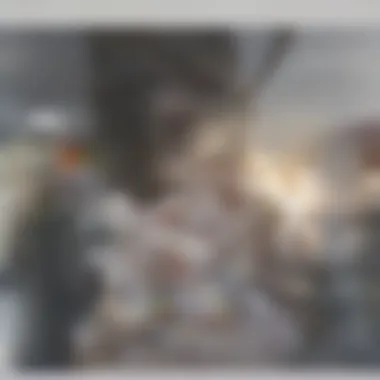
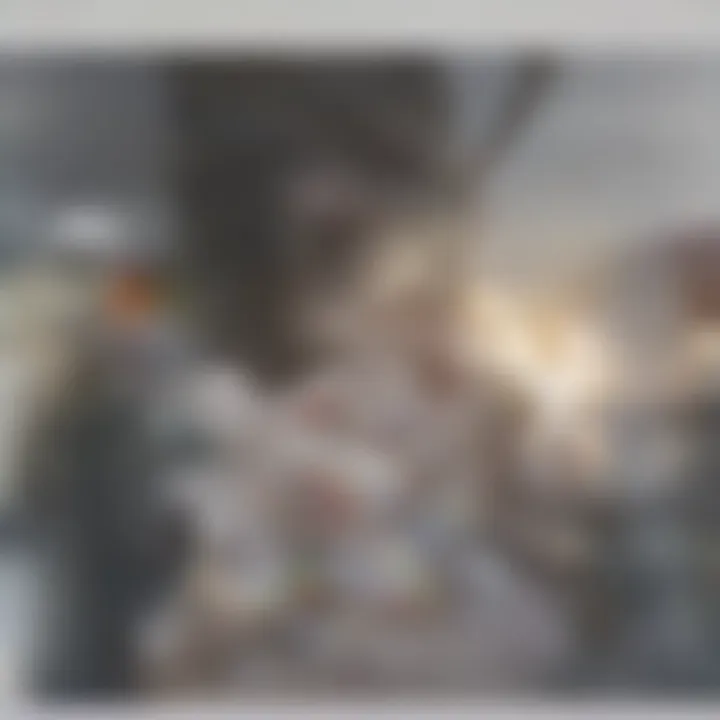
Intro
Data analysis is at the heart of scientific research. From biology to physics, the ability to interpret data accurately is essential for drawing valid conclusions. Understanding how to analyze data effectively can set apart a good researcher from a great one. This article delves into the methodologies, tools, and best practices in data analysis, aiming to empower students, researchers, educators, and professionals in their quest for clarity and rigor.
Research Context
Background Information
The process of data analysis in science encompasses several critical steps. It often begins with formulating a research question, which guides the study's design and data collection process. Researchers typically gather quantitative data, qualitative data, or both, depending on their field and research objectives. Once the data is collected, it undergoes various analysis techniques to extract meaningful insights.
Importance of the Study
In today's data-driven world, the significance of solid data analysis cannot be understated. Accurate data interpretation influences decision-making, policy crafting, and the advancement of knowledge across all domains. Researchers may use statistical methods, computational tools, and visualizations to understand patterns and trends in their data. For instance, the application of software like R or Python can enhance the efficiency and accuracy of data processing.
"Data doesn't just speak for itself; it needs careful interpretation and understanding."
Discussion
Interpretation of Results
The interpretation phase is often the culmination of the data analysis process. This is where researchers must critically assess the results obtained. It involves determining what the statistical outputs mean, and how they relate to the initial research questions. It is crucial to consider confounding variables and to understand the limitations of the data. A thorough interpretation will often highlight the relevance of findings in the broader context of existing research.
Comparison with Previous Research
Comparing new findings with previous research adds depth to the analysis and provides a framework for validating results. By examining how new data aligns or diverges from established literature, researchers can contribute to the ongoing conversation in their fields. This can also help in identifying gaps in knowledge that may warrant further investigation.
Closure
In summary, effective data analysis is fundamental in science. By grounding research in solid methodologies and best practices, researchers can ensure their findings are credible and impactful. The interaction between data collection, analysis techniques, and interpretation forms the backbone of a rigorous scientific inquiry. For those looking to enhance their data analysis skills, continuing education and practical application can significantly further their expertise.
The Importance of Data Analysis in Scientific Research
Data analysis is at the core of scientific research, serving as a fundamental process that translates raw data into meaningful insights. The significance of data analysis extends beyond mere number crunching; it embodies the methodical scrutiny of data that ultimately informs decision-making in various scientific domains. Engaging in data analysis allows researchers to discern patterns, test hypotheses, and validate their findings, thus enhancing the overall rigor of their studies. Without this critical step, the integrity and applicability of research findings can be severely compromised.
In recent years, the rapid advancements in technology and data collection techniques have further underscored the importance of efficient data analysis. The sheer volume of data generated in scientific inquiries can be overwhelming, making it imperative for researchers to adopt structured approaches to tackle their analytical challenges. This shift towards sophisticated data analysis is essential for achieving higher accuracy in scientific interpretations, leading to better conclusions that influence both academia and industry.
Understanding the Role of Data in Science
Data serves as the backbone of scientific inquiry. In essence, it is the tangible evidence that supports or refutes theories and observations. Scientists utilize various forms of dataโquantitative and qualitativeโto construct their arguments and draw conclusions. For example, quantitative data, which can be measured and expressed numerically, lends itself well to statistical analyses. Conversely, qualitative data encompasses descriptive information that can yield deeper insights into phenomena that numbers alone might not capture.
Moreover, data collection is not a haphazard process; it is carefully designed to ensure relevance and reliability. Researchers develop protocols for gathering data that are aligned with their research questions. This alignment is crucial because the right mix of data helps paint a more comprehensive picture of the studied phenomenon. The role of data in science thus becomes one of facilitating rigorous testing of hypotheses and ultimately contributing to the body of knowledge in a given field.
Impact of Data Analysis on Research Outcomes
The analysis of data significantly impacts the outcomes of scientific research. First and foremost, it shapes how results are interpreted. For instance, statistical methods allow researchers to determine the significance of their findings, enabling them to separate chance results from truly meaningful ones. When analyses are done correctly, the confidence in research conclusions improves.
Beyond interpretation, the analysis also influences the direction of future research. Findings can reveal gaps in understanding or highlight new areas worth exploring. For instance, if a data analysis suggests a correlation between two variables, it prompts further investigation into causality. The iterative nature of research is fueled by thoughtful data analysis, ensuring that the scientific community continuously evolves and improves.
"Effective data analysis bridges the gap between raw information and actionable insights, crafting a narrative that drives scientific understanding forward."
This impact is not limited to academia; industries also rely on data analysis to inform practices, policies, and innovations. In fields ranging from healthcare to environmental science, data-driven decisions have become the gold standard. Consequently, mastering data analysis is paramount for anyone looking to contribute meaningfully to scientific research.
Defining Research Questions and Objectives
Defining research questions and objectives is a critical step in the scientific process. This phase sets the foundation for the entire research project. When research questions are well-articulated, they guide the methodology, data collection, and analysis. A poorly defined question can lead to wasted resources and inconclusive results. Thus, clarity is essential. The objectives need to be measurable and achievable, making it easier to evaluate success after the research is complete.
One significant benefit of crafting specific objectives is that they allow researchers to narrow their focus. This focus can help avoid data overload and ensures that only relevant data is collected. Furthermore, clearly defined objectives make it easier for all team members to understand the purpose of the research. This collective understanding fosters collaboration and enhances productivity.
Crafting Clear and Testable Hypotheses
Crafting clear and testable hypotheses is an essential part of defining research questions. A hypothesis provides a statement that can be tested through research. It reduces ambiguity and focuses the investigation. A good hypothesis should be specific and based on prior knowledge or theory. For example, instead of stating that "exercise improves health," a more specific hypothesis could be "increased aerobic exercise reduces blood pressure in adults aged 40-60."
In addition to specificity, a testable hypothesis must also allow for empirical testing. It should predict a relationship between different variables. If a hypothesis is not testable, it cannot be scientifically evaluated, limiting the effectiveness of the research. Furthermore, strong hypotheses may result in advancements in knowledge in a particular field, making them invaluable.
Aligning Data Collection with Research Goals
Aligning data collection with research goals is vital for producing useful results. The data collection process must reflect the objectives set during the initial stages. A mismatch can lead to irrelevant data, complicating the analysis and potentially skewing results. For instance, if the goal is to understand a specific population, the data collected should represent that demographic accurately.
To effectively align data collection with research goals, researchers should prioritize the following steps:
- Identify Key Variables: Determine the independent and dependent variables relevant to the study.
- Select Appropriate Methods: Consider whether the research requires qualitative or quantitative methods, depending on the questions posed.
- Ensure Quality Control: Establish protocols for data collection to minimize errors. This includes training for data collectors and piloting data collection tools.
In summary, defining research questions and objectives is critical for guiding scientific inquiry. Crafting clear hypotheses and aligning data collection with research goals bolsters the quality of the research. Each step contributes to the validity and reliability of findings, ultimately enhancing the impact of the research.
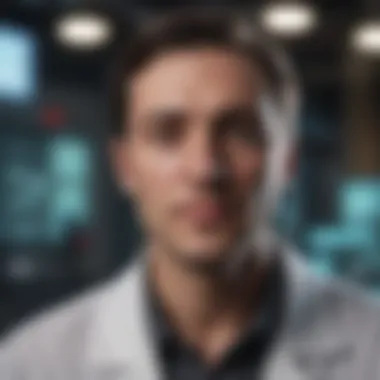
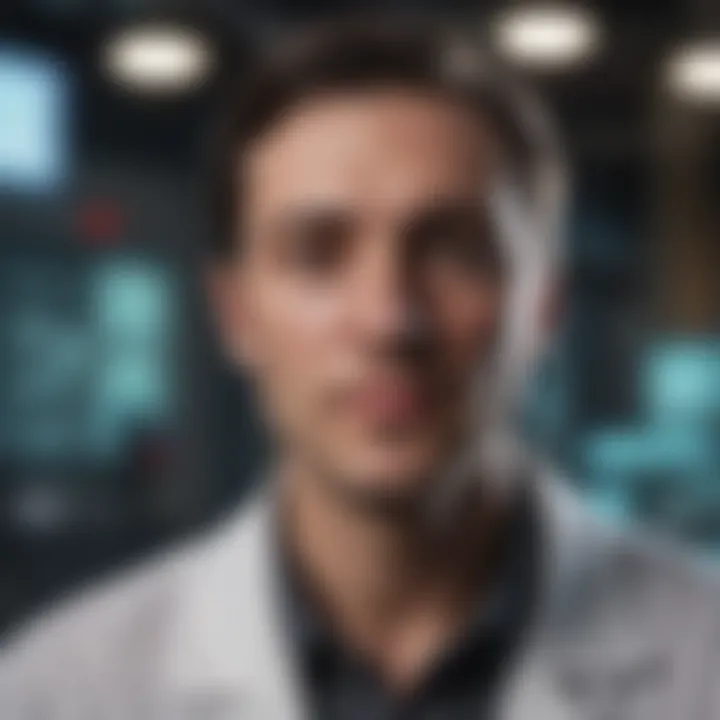
Data Collection Methods
Data collection methods form a core component of the overall data analysis process in scientific research. Understanding the appropriate methods to employ aids researchers in obtaining relevant and reliable data that directly aligns with their research objectives. Adequately collected data allows for insightful analysis and contributes to meaningful conclusions. The choice of data collection method influences not only the quality of data but also the interpretation of results.
Quantitative vs. Qualitative Data Collection
Quantitative data collection involves gathering numerical data that can be measured and analyzed statistically. This method is crucial for studies that require quantifiable evidence to support hypotheses. The strength of quantitative data lies in its ability to establish patterns, make comparisons, and facilitate the use of statistical analysis. Qualitative data collection focuses on gathering descriptive data that explores the nuances of a subject through subjective perspectives. This method is essential for understanding motivations, experiences, and beliefs. Researchers must consider their research questions and objectives to determine the most suitable data collection approach. Both methods have their unique benefits and limitations; hence, blending them can sometimes provide a more comprehensive insight.
Selecting Appropriate Data Collection Tools
Choosing the right data collection tools is a significant step that affects the potential for meaningful results. Tools can vary greatly depending on the method selected.
Surveys and Questionnaires
Surveys and questionnaires allow researchers to collect data from a broad audience efficiently. These tools can include closed-ended questions for quantitative analysis as well as open-ended questions for qualitative feedback. A key characteristic of surveys is their ability to gather data over a wide scope. This widespread reach makes them a popular choice in many studies. However, one downside may include the potential for low response rates, which can compromise the data reliability.
Interviews and Focus Groups
Interviews and focus groups involve direct interaction with participants, allowing for rich, qualitative insights. A significant characteristic of these tools is their depth; they provide a platform for participants to express their thoughts in detail. Their popularity stems from their ability to generate nuanced data not captured in surveys. This method, however, can be time-consuming and may introduce bias based on the interviewer's influence or group dynamics.
Observational Studies
Observational studies focus on recording behaviors and events as they occur in natural settings. This method captures real-world data devoid of interference. The primary advantage lies in its ability to provide context around behaviors. Observational studies are particularly beneficial when ethical concerns prevent experimental manipulations. One limitation is that they can be subject to observer bias which affects data integrity.
Experimental Designs
Experimental designs involve manipulation of variables to establish cause-and-effect relationships. This method is highly regarded for its scientific rigor, allowing for controlled conditions and repeatability. One unique feature is the ability to randomize subjects, reducing the risk of confounding variables. However, creating controlled experiments can be complex and may not always reflect natural conditions, potentially limiting the external validity of the findings.
"The choice of data collection method not only impacts the quality of data but also shapes the narrative of research findings."
Each method comes with its set of advantages and challenges, and researchers must carefully assess their requirements to select the most appropriate collection tool, ensuring alignment with their study's aims.
Data Management and Organization
Data management and organization is a vital aspect of scientific research. It pertains to the structured handling of data throughout its lifecycle, from collection to analysis and storage. The significance of effective data management cannot be overstated; it ensures data are accessible and retrievable, aids in maintaining data integrity, and ultimately supports more robust research outcomes. Researchers must realize that poorly managed data can lead to misleading results, wasted resources, and compromised credibility in their work. Hence, instituting systematic data management practices is essential.
Creating a Data Management Plan
A data management plan (DMP) is a comprehensive framework for managing data throughout the research process. It includes defining types of data to be collected, detailing how data will be organized, and specifying storage solutions. Establishing a DMP helps clarify the research process's structure, enabling researchers to anticipate challenges related to data handling.
Some key components of a robust DMP include:
- Data Description: Outline what kind of data will be collected, including formats, size, and nature.
- Storage and Backup: Specify how data will be stored during the research and what contingency plans are in place for data loss.
- Access and Sharing: Define who will have access to the data during and after the study, and how this data will be shared.
- Retention: Establish a timeline for how long data will be retained and the methods for eventual disposal or archiving.
Using tools such as DMPOnline can further streamline this process and ensure completeness. It allows researchers to create, store, and modify their plans in response to the evolving nature of their projects.
Ensuring Data Quality and Integrity
Maintaining data quality and integrity is crucial for producing reliable research findings. The concept of data quality encompasses accuracy, completeness, consistency, and reliability of the data collected. The reliability of research greatly hinges on these factors, which are fundamental for sound data analysis.
To ensure quality and integrity, consider the following:
- Regular Validation: Implement processes for periodic checks of data to identify errors or inconsistencies.
- Consistent Formats: Maintain uniform data formats and structures across datasets to facilitate analysis and comparison.
- Documentation: Keep thorough documentation about data collection methods, variables, and any changes made to the dataset over time. This documentation provides clarity and context for future analysis and helps other researchers replicate studies adequately.
- User Training: Ensure that all personnel involved in data collection and entry are well-trained in proper techniques to minimize human error.
"Well-managed data is not only about maintaining order but ensuring the accuracy and reliability of the research process."
Establishing a culture of appreciation for data integrity among the research team can greatly enhance overall research quality. Data management requires diligence and foresight, yet the payoff is invaluable in achieving credible research results.
Statistical Methods for Data Analysis
Statistical methods are critical in the context of data analysis in scientific research. They provide frameworks that assist researchers in making sense of raw data. Using these methods allows for systematic examination of data, enabling scientists to draw valid conclusions and support their hypotheses. Statistical analysis offers clarity by turning complex data into understandable formats, thus facilitating informed decision-making.
The choice of statistical methods can strongly influence research outcomes. Properly applied, they reduce ambiguities and illuminate patterns, trends, and relationships hidden in the data. Researchers must consider both the type of data they have and the specific research questions at hand when selecting appropriate analyses. An effective statistical approach not only aids in data interpretation but also enhances the rigor and reliability of research findings.
Descriptive Statistics: Summarizing Data
Descriptive statistics provide essential summaries of data sets, presenting them in a manageable form. They serve to condense large volumes of information into key metrics, helping researchers grasp the overall picture without getting lost in details.
Measures of Central Tendency
Measures of Central Tendency, such as mean, median, and mode, are fundamental traits of descriptive statistics. They reflect the most common or typical values within a data set. The mean is especially popular as it incorporates all data points, providing a comprehensive summary of the data set. However, it can be sensitive to outliers. The median, on the other hand, is often a better measure in skewed distributions since it is unaffected by extreme values. The mode highlights the most frequently occurring value, which offers insights into data concentration.
Measures of Variability
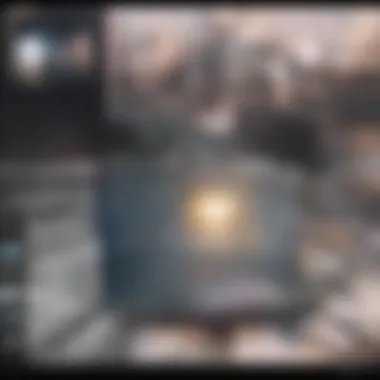
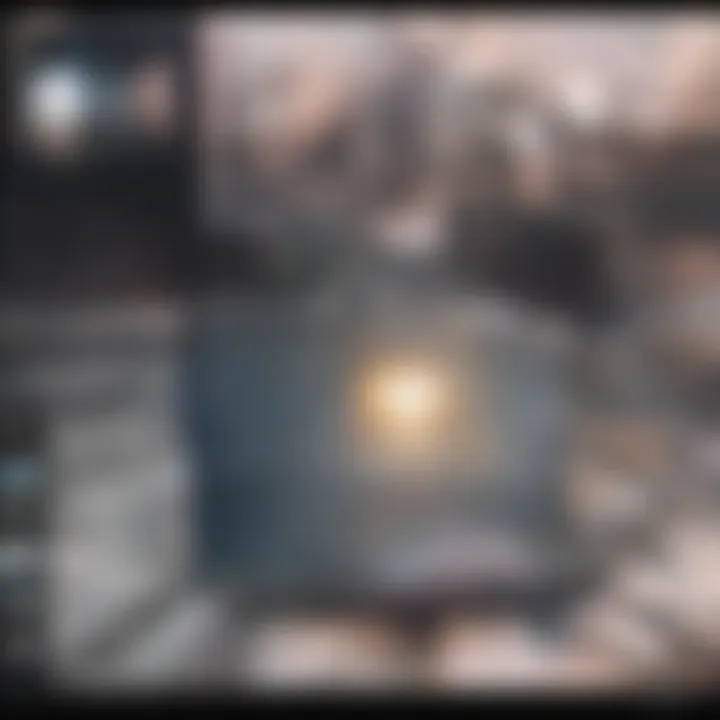
Measures of Variability, including range, variance, and standard deviation, inform us about the spread of data. While measures of central tendency indicate where most of the data points lie, variability shows the extent to which they diverge from these central points. A small standard deviation suggests that data points are close to the mean, whereas a large one indicates considerable divergence. This aspect is crucial for understanding the reliability of data and ensuring robust conclusions.
Inferential Statistics: Drawing Culminations
Inferential statistics extends the insights gained from sample data to larger populations. This allows researchers to make predictions or generalizations based on their collected data sets.
Hypothesis Testing
Hypothesis Testing is a critical feature of inferential statistics. It offers a structured method for validating or rejecting a hypothesis based on sample data. This process aids in determining if an observed effect is statistically significant or likely due to random chance. It enables researchers to draw reliable conclusions and direct future studies based on findings.
Confidence Intervals
Confidence Intervals provide a range within which researchers can expect the true population parameter to fall. They add an additional layer of interpretation to hypothesis testing. Instead of just concluding whether a hypothesis is accepted or rejected, confidence intervals indicate the level of precision and uncertainty associated with estimates. This characteristic enhances the understanding of the data, making it a valuable component in scientific research.
Regression Analysis
Regression Analysis is a powerful statistical method that helps in examining the relationship between variables. It allows researchers to identify trends and make predictions based on data. Unlike some other techniques, it provides insights into how much one variable may influence another. However, the effectiveness of regression can depend on the assumptions made, such as linearity and independence of errors. Careful consideration of these elements is essential for valid results.
Statistical methods in data analysis are not just tools; they are the backbone supporting scientific inquiry.
Data Visualization Techniques
Data visualization plays a crucial role in scientific research. It transforms complex datasets into understandable visual formats, aiding in the interpretation of data and facilitating clearer insights. The presentation of data visually can help researchers identify patterns, trends, and correlations that may not be evident in raw data. Moreover, effective visualization contributes to better communication of research findings, making it easier to convey the significance of data to both specialized and general audiences. In this section, we will explore how to choose the right visualization tools and how to interpret various visual representations of data.
Choosing the Right Visualization Tools
When selecting visualization tools, one must consider several factors. First, the specific nature of the data set is important. Some tools cater to large datasets, while others may best serve smaller, simpler datasets. Additionally, the audience for the data presentation must also be taken into account. Tools that are user-friendly and visually appealing can enhance the understanding of the information presented.
Commonly used visualization tools include Microsoft Excel, Tableau, and R with ggplot2. Each of these tools offers distinct advantages, allowing for diverse forms of data representation.
- Microsoft Excel: Accessible and ubiquitous, it is ideal for basic charts and graphs.
- Tableau: Known for its interactive dashboards, useful for exploring trends dynamically.
- R: A statistical programming language with extensive packages for data visualization, suited for advanced statistical representations.
Interpreting Visual Data Representations
The interpretation of visual data representations is critical in data analysis. Understanding the kind of graph or chart used helps communicate the dataset's story effectively.
Graphs and Charts
Graphs and charts are popular for summarizing data visually. They can take various forms, such as bar charts, line charts, and pie charts. These visual tools enable the viewer to quickly grasp key insights without delving into numbers alone.
The key characteristic of graphs and charts is their ability to showcase trends over time, comparisons, and proportions. For instance, bar charts allow easy comparison between different categories, while line charts can display how data changes over time.
While beneficial, there are disadvantages. Poorly designed graphs can mislead viewers. Therefore, it is crucial to ensure accuracy in scales, labels, and overall design. Avoid cluttering visuals, to maintain clarity and focus on the intended message.
Heat Maps and Infographics
Heat maps and infographics serve distinct purposes in data visualization. Heat maps are effective for presenting complex data through colors. They can show areas of intensity and help identify patterns across varying parameters.
One unique feature of heat maps is their ability to display a large amount of data visually compellingly. They are often used in fields like epidemiology or social sciences, where spatial trends are significant. However, the downside can be the potential for misinterpretation if color scales are not well explained or understood.
Infographics, on the other hand, combine visual data with narrative, making them very engaging. They are especially useful in conveying complex information succinctly. A well-designed infographic can summarize an entire study's findings in an accessible format. However, if overloaded with information, they risk losing clarity.
In summary, selecting appropriate visualization techniques is essential for effective data analysis and communication. Proper interpretation and design can enhance understanding and facilitate impactful sharing of research findings.
Interpreting Analysis Results
In scientific research, interpreting analysis results forms a pivotal part of the data analysis process. It is where the raw data transitions into meaningful insights and conclusions. This segment delves into understanding statistical significance and discussing the implications and limitations of findings, both of which enrich the research narrative.
Understanding Statistical Significance
Statistical significance is a foundational concept in data analysis. It helps researchers determine whether the observed effects in their data are likely due to chance or represent genuine phenomena. Commonly, researchers utilize a significance level (often set at 0.05) to assess this. If the p-value is lower than this threshold, researchers can confidently assert that the results are statistically significant.
To interpret statistical significance effectively, it is crucial to:
- Understand the context of the study.
- Use correct statistical tests suitable for the data type and research question.
- Recognize that statistical significance does not imply practical significance or relevance.
This understanding aids in formulating valid conclusions and enhances the credibility of the research.
Discussing Implications and Limitations of Findings
Every research study, no matter how rigorous, carries implications and limitations. Discussing these aspects provides transparency and depth to the research work. Recognizing the implications can illuminate how findings might influence the field, potentially guiding future research or practical applications. Conversely, comprehending the limitations surrounding the study can help curb overgeneralization.
Considerations for discussing implications and limitations include:
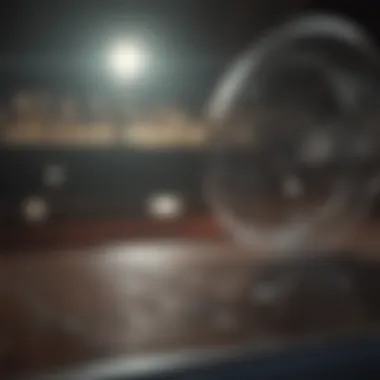
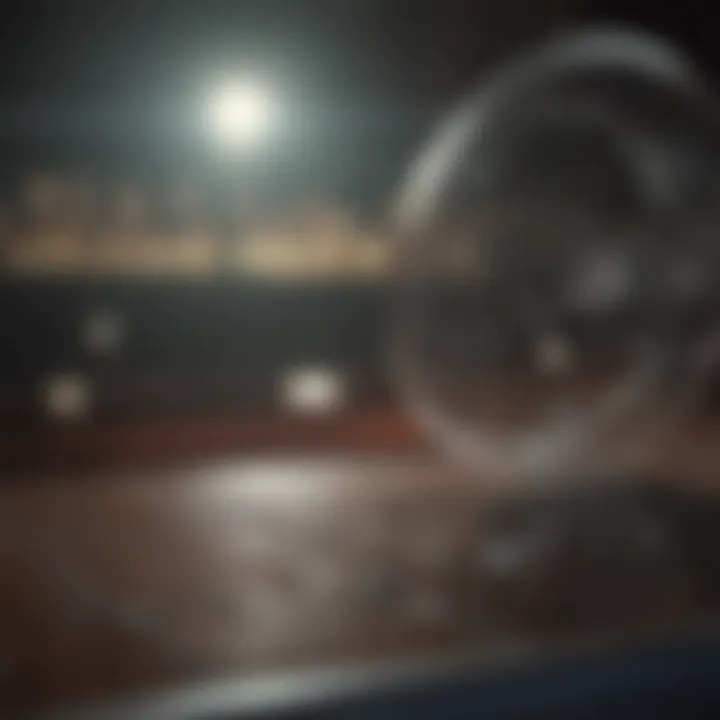
- Sample Size: A smaller sample may limit the generalizability of results.
- Methodological Constraints: Certain methods might introduce biases.
- External Factors: Variables outside the control of the study can impact outcomes.
Clearly articulating these elements fosters trust among readers and peers within the scientific community.
Reporting and Presenting Research Findings
Reporting and presenting research findings is a critical aspect of scientific inquiry. It serves as the bridge between data analysis and knowledge dissemination, ensuring that insights gained from research are communicated effectively to the intended audience. The key elements involved in this process include clarity, structure, and engagement. Proper structuring of reports helps readers, be they peers, funding bodies or the general public, to grasp the research's significance and implications.
Effective communication of results elevates the quality of scientific discourse. By reporting findings in a transparent manner, researchers uphold the integrity of their work, facilitating reproducibility and fostering trust among stakeholders. Considerations such as accuracy, accessibility, and the right choice of presentation format play vital roles in how findings are perceived and understood.
"Clear communication is essential for scientific progress. It ensures that knowledge is not just generated but also shared in a meaningful way."
Overall, well-structured reports and effective communication can enhance the impact of research, making it more likely to influence future studies and real-world applications.
Structuring Research Reports
The structure of research reports must follow a logical framework that guides readers through the research process. A typical research report includes several key sections:
- Abstract: A concise summary of the research, providing a quick overview of the objectives, methods, results, and conclusions.
- Introduction: This section contextualizes the research, outlining the problem, research questions, and objectives.
- Methods: Here, the methodology used for data collection and analysis is detailed. It should allow other researchers to replicate the study.
- Results: This section presents the data findings without interpretation, typically accompanied by tables, graphs, or charts.
- Discussion: The interpretation of results takes place here, exploring implications, limitations, and areas for future research.
- Conclusion: A brief recap of the main findings and their relevance.
- References: Proper citation of sources used in the research.
Maintaining consistency across these sections enhances readability and facilitates comprehension. This structured approach helps in communicating intricate research insights effectively.
Effective Communication of Results
To communicate results effectively, researchers must translate complex data and findings into clear, relatable language. This requires an understanding of the audience's background and knowledge level. Key practices include:
- Simplicity: Use straightforward language and avoid jargon where possible.
- Visual Aids: Incorporate graphs and charts to illustrate data trends, making them easier to understand.
- Interactive Formats: Consider using online platforms, webinars, or visual storytelling to engage the audience more dynamically.
Taking into account feedback and questions from the audience helps refine presentations and reports further. Engaging with different audiences through tailored communication can result in more impactful dissemination of research findings. Doing so not only contributes to greater academic collaboration but also aids in translating findings into real-world applications.
The Ethical Considerations in Data Analysis
Understanding ethical considerations in data analysis is crucial for ensuring the trustworthiness and integrity of scientific research. Researchers who analyze data carry the responsibility not only for their findings but also for the methods and processes they employ to acquire those results. Conducting research ethically contributes to the credibility of science itself.
One primary element of ethical data analysis is maintaining transparency in research. This involves openly sharing methodologies, data sources, and how conclusions were drawn. Transparency allows other researchers to evaluate the validity of findings and to replicate studies where necessary. When researchers are honest about their processes, it fosters collaboration and trust within the scientific community. Moreover, transparency helps in eliminating biases and ensuring that results are not misleading.
Maintaining transparency leads to several benefits, including:
- Promoting trust between researchers and the public
- Encouraging accountability in research practices
- Enabling independent verification of results
Maintaining Transparency in Research
Transparency in research goes beyond merely reporting results. It encompasses a comprehensive presentation of study design, data collection methods, analysis procedures, and potential conflicts of interest. Researchers should provide a clear account of their methodologies. They need to state whether they followed established protocols or if any deviations occurred.
Furthermore, itโs essential to document and share research data wherever possible. Open data initiatives allow others to access datasets, which can help in validating findings and applying the same data to different contexts. It is important, however, to weigh the need for transparency against the potential risks of sharing sensitive information.
"Transparency in research is not just a best practice; it is a fundamental ethical obligation to ensure scientific integrity."
Protecting Data Privacy and Confidentiality
In tandem with transparency, protecting data privacy and confidentiality is another critical ethical consideration in data analysis. Researchers often handle sensitive information, such as personal data about individuals or proprietary data from businesses. It is vital to safeguard this information from unauthorized access or misuse.
To manage this sensitive data responsibly, researchers must implement robust data protection strategies. This includes anonymizing data, which involves removing personally identifiable information or using aggregation methods to ensure that individuals cannot be identified from the results. In some cases, obtaining informed consent from participants is necessary, ensuring they understand how their data will be used and safeguarded.
The following practices can help ensure that data privacy remains intact:
- Conducting regular audits of data usage and storage
- Establishing strict access controls for data handling
- Implementing strong encryption methods for data transmission and storage
Balancing transparency with privacy can be challenging. However, it is essential to recognize that both are critical for ethical research practices. By prioritizing ethical considerations, researchers uphold the integrity of their work and maintain the trust of their audience.
Future Trends in Data Analysis
The analysis of data is continually evolving, driven by rapid advancements in technology and the changing nature of scientific inquiry. Understanding future trends in data analysis is crucial for researchers, students, and professionals. This section explores the implications of new technologies and methodologies, highlighting their potential benefits and considerations.
Emerging Technologies and Their Impact
Technology is reshaping how researchers collect, analyze, and interpret data. Several key technologies are becoming increasingly significant:
- Artificial Intelligence and Machine Learning: These technologies automate the analysis process, enabling complex data sets to be processed more efficiently. Machine learning algorithms can identify patterns that might not be obvious to human analysts, offering deeper insights into the data.
- Big Data Tools: As the volume of data generated continues to increase, tools designed to process and analyze large datasets become essential. Platforms like Apache Hadoop and Spark allow researchers to handle vast amounts of information and extract meaningful insights without extensive delays.
- Cloud Computing: This technology enables researchers to access data storage and processing power remotely. It facilitates collaboration among scientists across different locations, enhancing data sharing and analysis. With cloud computing, the limitations of local computing resources diminish, allowing for larger and more diverse data collections.
"The future of data analysis is not just about tools; it's about how we integrate those tools to innovate research methods and enhance findings."
These emerging technologies have significant impacts: they allow for faster processing times, reduce human error, and enable more sophisticated statistical models. However, they also pose challenges. For instance, reliance on algorithms without sufficient understanding of their operation can lead to misinterpretations of results. Researchers must remain critical of the analysis produced by these technologies.
The Evolving Role of Data Analysts in Research
With advancements in technology, the role of data analysts is evolving. Analysts are transitioning from mere data processors to becoming strategic partners in the research process. Their skills are in high demand as they provide essential insights that guide decision-making. Key aspects of this evolution include:
- Enhanced Collaboration: Data analysts are increasingly collaborating with domain experts to shape research questions and methodologies. Their contributions ensure data analyses align with specific research objectives and incorporate the appropriate knowledge from various fields.
- Focus on Data Interpretation: Beyond just analyzing data, analysts must also interpret results and communicate findings effectively to stakeholders. This demand necessitates a blend of technical and communication skills, making analysts more multifaceted professionals.
- Proactive Problem Solving: The complexity of data now requires analysts to proactively identify potential research issues and suggest methodologies and analyses. Their ability to foresee analytical challenges can significantly impact the research outcome.
In summary, the future of data analysis is characterized by emerging technologies and an evolving role for analysts. Researchers must adapt to these changes, taking advantage of new tools while cultivating collaborative relationships with data analysts. By doing so, they can ensure that their studies remain rigorous and relevant in a rapidly changing scientific landscape.