Understanding Automated Intelligence: Concepts & Applications
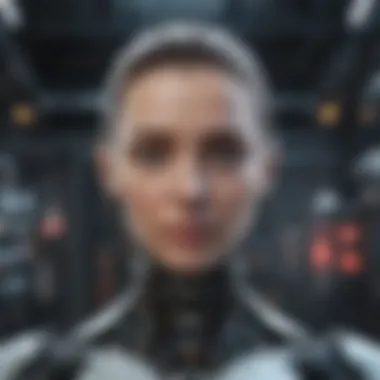
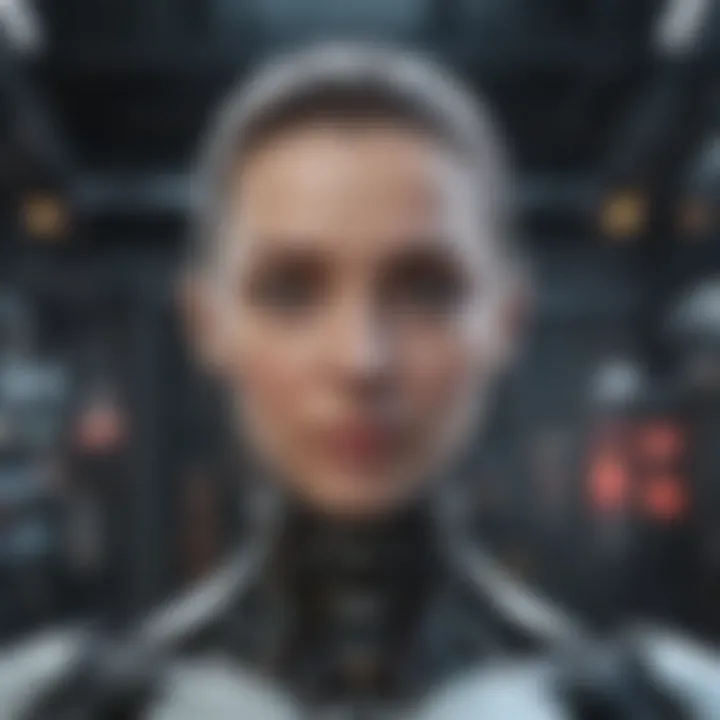
Intro
Automated intelligence is a rapidly evolving field that integrates concepts from automation and artificial intelligence. This fusion creates systems that can perform complex tasks with minimal human intervention. The significance of understanding automated intelligence lies in its potential to improve efficiency and decision-making across various sectors. In this article, we will explore its key components, methodologies, and applications.
The rise of automated intelligence is primarily driven by advances in technology. Organizations use automated systems to analyze data and uncover insights that aid in strategic planning and operations. As industries evolve, the relevance of automated intelligence becomes increasingly apparent. This study aims to clarify these concepts, offering a richer understanding of their impact on the present and future.
Research Context
Background Information
Automated intelligence encompasses machine learning, data analytics, and robotic process automation. These elements work together to enable systems to learn from data. For instance, machine learning algorithms can identify patterns within large datasets, while robotic process automation can execute predefined tasks efficiently. This synergy facilitates a more streamlined approach to problem-solving.
The concept also connects to broader themes in technology, such as big data and cloud computing. As data generation surges, the need for systems that can parse and interpret this information grows. This context provides a backdrop for the study of automated intelligence.
Importance of the Study
Understanding automated intelligence is pivotal for several reasons:
- Informs Stakeholders: Educates business leaders and technologists on current trends and potential impacts.
- Drives Innovation: Encourages the development of new solutions that leverage automated intelligence.
- Facilitates Ethical Discourse: Provides a foundation for discussions surrounding the ethical implications of automation and AI.
By shedding light on these areas, this article will contribute to the growing discourse on automated intelligence.
Discussion
Interpretation of Results
The findings of this study highlight how automated intelligence transforms various sectors. In healthcare, for example, automated systems can analyze patient data for trends that inform treatment plans. In finance, algorithms help in fraud detection by spotting irregular transaction patterns. The practical implications of these applications suggest a future where automated intelligence becomes a cornerstone of operational excellence.
Comparison with Previous Research
While earlier research focused primarily on discrete aspects of automation or AI, contemporary studies emphasize the convergence of these fields. This study extends previous analyses by illustrating how automated intelligence fuses different methodologies into cohesive solutions. Unlike traditional studies, which tended to compartmentalize topics, this article interprets findings as part of an integrated framework, underscoring the importance of holistic understanding in technology's evolution.
Automated intelligence represents more than just a technological advance; it signals a transformation in how industries operate and make decisions.
Defining Automated Intelligence
The concept of automated intelligence is critical in today's technological landscape. As industries increasingly rely on intelligent systems, understanding what constitutes automated intelligence becomes vital. The term encapsulates the ability of machines to perform tasks that typically require human intellect. This includes problem-solving, learning, and adapting to new information. Recognizing automated intelligence helps in assessing its impact on various sectors, ranging from healthcare to finance.
The Origin of the Term
Automated intelligence emerged as a term to represent the intersection of automation and artificial intelligence. Initially, automation referred to mechanized processes that did not require cognitive abilities. However, as computers became more capable, the need for intelligenceβspecifically, the capacity to analyze data and make decisionsβgrew. This shift made the combination of automation and AI essential. Understanding the term's roots provides clarity on its evolution and relevance in recent technological developments.
Key Components of Automated Intelligence
Automated intelligence comprises several vital components that work together to optimize processes. Three key elements deserve special attention:
Data Processing
Data processing is fundamental to automated intelligence. It involves collecting, organizing, and analyzing vast amounts of information. In this context, data processing contributes significantly to the overall efficiency of automated systems. One key characteristic is its ability to handle large datasets quickly. This is a beneficial choice because, without effective data processing, the subsequent phases of automation cannot function optimally. A unique feature of this aspect is its scalability, allowing systems to grow in capacity as data volumes increase. However, challenges persist, particularly around data quality and relevance, impacting the effectiveness of processing systems.
Machine Learning
Machine learning represents a major facet of automated intelligence. It enables systems to learn from data patterns and improve their predictions or decisions over time. The key characteristic of machine learning is its adaptability, making it a popular choice in various applications. By continually learning, these systems can refine their operations. A unique feature of machine learning is its various techniques, such as supervised and unsupervised learning, which cater to different data scenarios. Nonetheless, challenges remain, including the need for substantial labeled datasets and concerns surrounding overfitting.
Decision-Making Algorithms
Decision-making algorithms play a crucial role in automated intelligence. They facilitate the selection of actions based on processed data and learned insights. The key characteristic of these algorithms is their ability to optimize choices under uncertainty. This ability makes them essential for dynamic environments where quick adaptations are needed. A unique aspect is the variety of algorithms available, from simple rule-based systems to complex neural networks. While they offer significant advantages, such as improved decision speed and consistency, there are also risks, including reliance on biased data, which can lead to erroneous conclusions.
Historical Context and Evolution
Understanding the historical context and evolution of automated intelligence is essential for grasping its current capabilities and future potential. Automation has roots that span back decades. It started with the aim to reduce human effort and enhance productivity across various sectors. Over the years, the landscape transformed remarkably, leading into the domain of automated intelligence, which now significantly improves decision-making processes.
Early Developments in Automation
The early days of automation focused primarily on mechanical processes. Simple machines facilitated repetitive tasks, boosting efficiency in manufacturing. For example, assembly lines revolutionized industries like automotive production. These foundational steps marked the beginning of automation, paving the way for more sophisticated systems. The advent of the first programmable logic controllers in the late 1960s enhanced this trajectory, allowing for more complex operations without human intervention. Such systems could execute pre-defined instructions, laying the groundwork for future innovations in automated technology.
Transition to Artificial Intelligence
As the 20th century progressed, automation began to incorporate forms of intelligence. This transition was driven by the rise of computers and advancements in algorithms. Artificial Intelligence emerged in the 1950s, focusing on mimicking human cognitive functions. By merging automation with AI, systems could now learn from data patterns. The transition opened channels for more versatility in applications, particularly in areas requiring judgment and adaptation, like healthcare and finance. It signified a pronounced shift, from merely performing tasks to making informed decisions.
Notable Milestones in Automated Intelligence
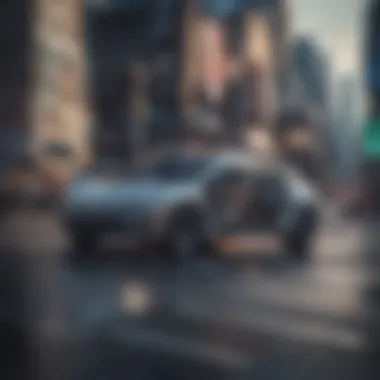
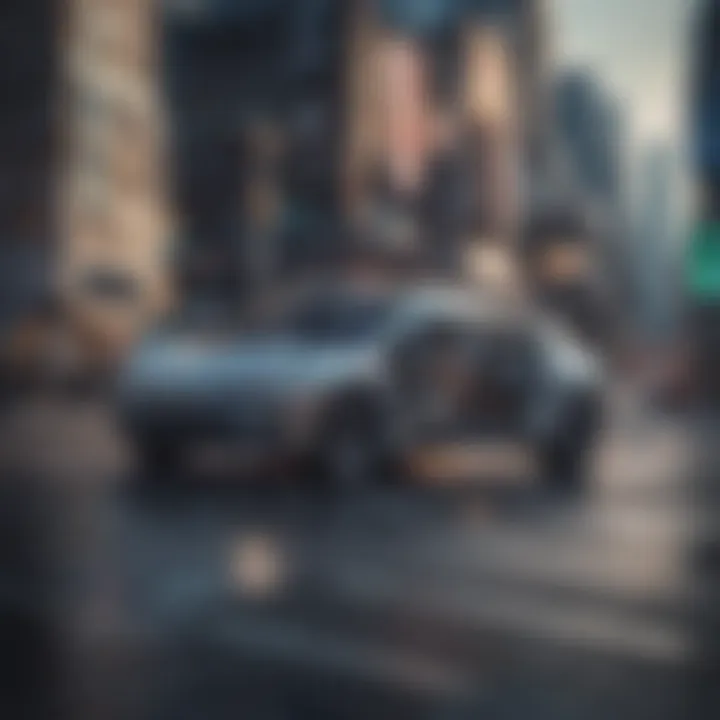
Several pivotal milestones underline the progress of automated intelligence. The introduction of backpropagation in neural networks during the 1980s gave impetus to machine learning, which propelled systems to enhance their performance iteratively. In the late 20th century, breakthroughs in natural language processing debuted, allowing machines to understand and generate human language with more accuracy.
Moreover, the launch of self-driving cars and sophisticated recommendation systems exemplify milestones in practical applications of automated intelligence. These achievements not only reflect the growing capabilities of technology but also pose new questions regarding ethical implications and the alignment of such systems with human values.
"Understanding the evolution of automated intelligence helps in recognizing not just where we are, but where we might be headed."
As we progress into a future increasingly shaped by automated systems, understanding these historical elements will aid both scholars and practitioners in navigating the complex landscape of automated intelligence.
Core Technologies in Automated Intelligence
Core technologies in automated intelligence are crucial as they provide the foundation for the systems that enhance efficiency and decision-making across various applications. These technologies include machine learning techniques, natural language processing, and robotic process automation. Each plays an essential role in analyzing data, interpreting human language, and automating tasks, respectively. Understanding these components is vital for grasping how automated intelligence can be effectively utilized in different sectors, including healthcare, finance, and manufacturing. The growing importance of these technologies stems from their ability to process large volumes of data and produce actionable insights with minimal human intervention.
Machine Learning Techniques
Machine learning is a subset of artificial intelligence that allows systems to learn from data to make decisions without explicit programming. It enhances the learning process, enabling automated intelligence systems to adapt and improve over time.
Supervised Learning
Supervised learning is a machine learning technique that relies on labeled data to train algorithms. This approach is designed to make predictions or classify data based on prior examples. The key characteristic of supervised learning is its reliance on a clear input-output mapping, which allows for effective model training. It is a widely used option because of its applicability across numerous domains, such as image recognition and spam detection.
The unique feature of supervised learning is its capacity to perform well with sufficient amounts of quality labeled data. One advantage is that it typically yields high accuracy given enough relevant training data. However, it does have its disadvantages; collecting and labeling data can be resource-intensive and time-consuming.
Unsupervised Learning
Unsupervised learning refers to a type of machine learning that works with unlabeled data. Its objective is to uncover patterns or structures within the data without prior knowledge of the outputs. The key characteristic of unsupervised learning is its ability to segment or cluster data based on intrinsic properties, making it useful for exploratory data analysis.
This technique is beneficial when the outcomes are unknown or when data is abundant but lacks labels. Its unique feature is the capability to generate insights without requiring human intervention in the labeling process. The potential disadvantage is that interpreting the results can be challenging, as the insights produced might not always be immediately actionable or clear.
Reinforcement Learning
Reinforcement learning is a type of machine learning focused on training algorithms through a system of rewards and penalties. An agent learns to make decisions by interacting with an environment, receiving feedback from actions taken. The key characteristic of reinforcement learning is its focus on finding a balance between exploration and exploitation of available knowledge.
This method is increasingly popular, especially in applications such as robotics and game development, due to its ability to address complex problems with dynamic environments. A distinctive feature is that it learns from its experiences and continually refines its approach. The disadvantage lies in its requirement for significant computational resources and time to achieve optimal performance.
Natural Language Processing
Natural language processing, or NLP, is a technology that enables machines to understand, interpret, and respond to human language in a way that is both meaningful and useful. This capability is essential for driving automated communication and analysis processes across numerous applications. NLP embodies the intersection of linguistics and artificial intelligence, making it invaluable for tasks such as sentiment analysis, chatbots, and virtual assistants.
Robotic Process Automation
Robotic process automation, or RPA, involves the application of software robots or "bots" to automate repetitive tasks that were previously performed by humans. RPA is particularly significant in business environments, as it improves efficiency and reduces operational costs. By automating mundane tasks, RPA frees up human employees to focus on more strategic and value-adding activities.
Conversely, implementing RPA can present challenges. Organizations must ensure the bots are correctly configured and maintained to avoid errors in automated processes. This technology represents a powerful tool for enhancing efficiency, but careful management and oversight are necessary to achieve the desired outcomes.
Applications of Automated Intelligence
Automated intelligence has found significant applications across various industries. The ability of these systems to process large amounts of data with remarkable speed enhances efficiency and accuracy in operations. Examining the distinct applications helps us to appreciate their real-world impact. This section highlights crucial sectors where automated intelligence plays a vital role and how they contribute toward achieving operational goals.
Healthcare Innovations
Diagnostic Systems
Diagnostic systems represent a pivotal application of automated intelligence in healthcare. These systems analyze medical imaging and patient data to assist in diagnosing diseases. A key characteristic of diagnostic systems is their reliance on algorithms that can identify patterns in data. This makes them a popular choice, as they can quickly and accurately pinpoint potential health conditions. One unique feature of diagnostic systems in healthcare is the use of machine learning to continuously improve accuracy through exposure to large datasets. Nevertheless, the over-reliance on technology can pose risks, including misdiagnosis when systems fail or data quality is compromised.
Patient Monitoring
Patient monitoring systems utilize automated intelligence to track patient vitals continuously. This contributes significantly to improving healthcare outcomes. The key characteristic of these systems is their real-time data collection capabilities. They enable healthcare professionals to respond promptly to any changes in a patientβs condition, making it a beneficial application in critical care environments. A unique feature is the integration of alerts for abnormal readings, enhancing rapid intervention. However, while effective, patient monitoring systems can generate false alarms, which may lead to unnecessary anxiety among patients and staff.
Personalized Medicine
Personalized medicine emphasizes patient-centric approaches through tailored treatment plans based on individual genetic profiles. This innovative application of automated intelligence can significantly enhance therapeutic effectiveness. Its key characteristic lies in analyzing complex data to identify the most suitable treatments for specific patient demographics. This use of data analytics allows for a more efficient allocation of healthcare resources. An important aspect of personalized medicine is its potential to reduce adverse drug reactions by customizing therapies. However, challenges remain, such as ensuring data privacy and the ethical implications of genetic testing.
Manufacturing and Supply Chain
Predictive Maintenance
Predictive maintenance applies automated intelligence to monitor equipment conditions and predict failures before they occur. This approach extends the lifespan of machinery and minimizes downtime. The main characteristic of predictive maintenance is its proactive nature, allowing companies to address issues before they escalate. It is a widely adopted choice because of its ability to save costs associated with unexpected equipment failures. A standout feature of this method is the integration of sensors that monitor performance metrics. However, to maximize benefits, organizations must invest in appropriate technologies and training.
Inventory Management
Automated intelligence streamlines inventory management by analyzing demand patterns and optimizing stock levels. The importance of this application lies in reducing overhead costs and ensuring product availability. Its key characteristic is the automatic adjustments in inventory based on predictive analytics. This makes inventory management more efficient and less prone to human error. An essential feature is the real-time tracking of inventory levels, which helps businesses respond swiftly to fluctuations in demand. However, insufficient data integration can lead to inaccurate forecasts and stockouts.
Quality Control
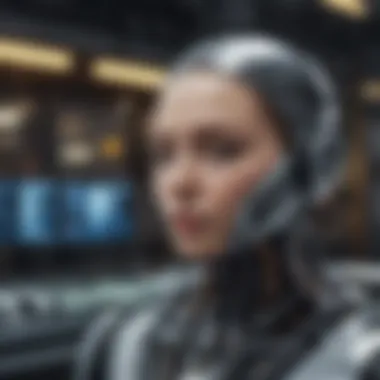
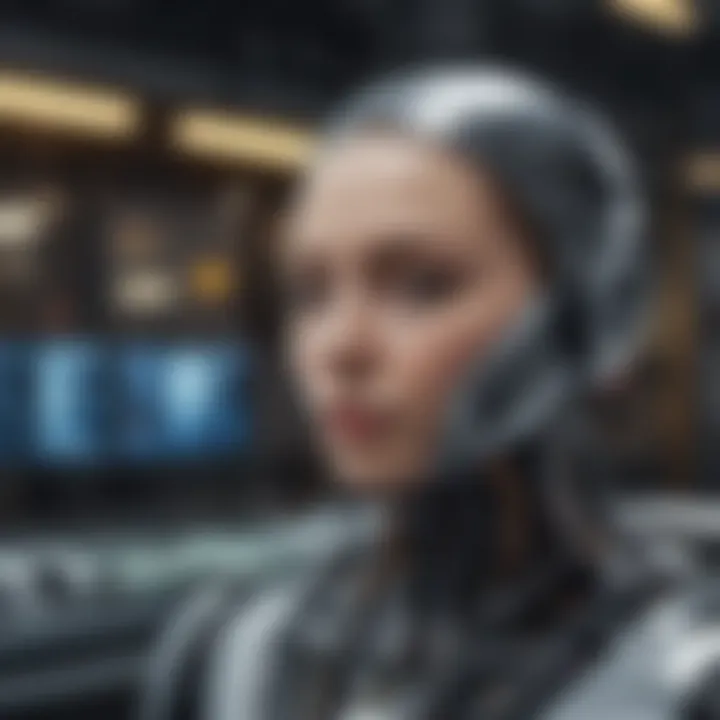
Quality control applications utilize automated intelligence to ensure products meet specified standards. This process significantly contributes to maintaining high-quality outputs. The primary characteristic of these systems is the ability to conduct thorough inspections at high speeds. This efficiency makes quality control a crucial component of manufacturing. A unique feature is the use of vision systems that can detect defects during production in real-time. Nevertheless, relying heavily on automation may lead to oversights if systems do not adapt to unexpected variations in product designs.
Financial Services
Fraud Detection
Fraud detection systems employ automated intelligence to identify suspicious activities across financial transactions. They play a crucial role in safeguarding against losses. The main characteristic of these systems is their capacity to analyze transaction patterns for anomalies. This makes them a highly effective choice for financial institutions. A distinct feature is the use of machine learning algorithms that learn from previous fraud cases to improve detection capabilities continuously. However, false positives can be a drawback, causing inconvenience to legitimate users.
Risk Assessment
In financial services, risk assessment is fundamental for evaluating potential losses in investments or loans. Automated intelligence applications facilitate better analysis of risk factors. The key characteristic of risk assessment is its predictive capability, allowing institutions to foresee potential issues. It is fundamental to making informed decisions. A unique feature is the integration of data from multiple sources, which provides a comprehensive view of potential risks. However, relying solely on automated assessments without human analysis can lead to incomplete evaluations.
Algorithmic Trading
Algorithmic trading leverages automated intelligence to execute trades at high speeds based on pre-defined criteria. This application greatly enhances market efficiency. Its main characteristic is the ability to process market data and execute trades far faster than any human trader. This makes algorithmic trading very popular among institutional investors. A unique feature is the use of algorithms that can adapt to changing market conditions. Nonetheless, market volatility can pose risks, and automated strategies must be carefully monitored to avoid significant losses.
Understanding the applications of automated intelligence allows us to see its transformative potential in modern industries. From improving patient care to enhancing financial security, its impact is profound.
Advantages of Utilizing Automated Intelligence
Automated intelligence offers several advantages that are reshaping industries and improving processes. Understanding these benefits is crucial. They include increased efficiency, enhanced accuracy, and cost reduction. Each of these elements contributes significantly to productivity and operational success.
Increased Efficiency
One of the most notable benefits of automated intelligence is the boost in efficiency. Organizations can automate repetitive tasks, thereby liberating employees to focus on more strategic activities. For instance, in data processing, automated tools can handle vast amounts of information much faster than a human can. This rapid processing speed not only saves time but also accelerates decision-making cycles.
When efficiency is improved, companies can respond to market changes more swiftly. They are better equipped to meet customer demands without unnecessary delays. This agility can lead to a competitive advantage, setting a business apart in its industry.
Enhanced Accuracy
Another significant advantage of utilizing automated intelligence is the enhancement of accuracy. Automated systems operate with precision. They minimize errors commonly associated with manual processes. In fields like healthcare, where incorrect data entry can have serious consequences, automated diagnostic tools ensure a higher level of reliability. In finance, algorithms can analyze data with greater consistency, identifying fraudulent transactions more effectively.
Moreover, the use of automation in data analysis allows for continuous monitoring. It ensures that data is processed consistently, maintaining a high level of quality. As a result, organizations can trust the insights generated by their automated systems.
Cost Reduction
Cost reduction is a compelling advantage of automated intelligence. By streamlining processes, companies can decrease the number of hours required to complete tasks. This reduction in labor time translates directly to savings in operational costs. For instance, robotic process automation can handle back-office functions, such as invoicing or payroll, at a fraction of the cost of hiring additional staff members.
Furthermore, the long-term savings are significant. Even though there may be initial investments in technology, the ongoing cost of ownership is usually lower than traditional methods. For businesses aiming to improve their bottom line, utilizing automated intelligence can be a key driver of financial performance.
Automating intelligence not only enhances productivity but also plays a significant role in achieving long-term cost efficiency.
Challenges and Limitations
Understanding the challenges and limitations in automated intelligence is crucial for realizing its full potential. This section delves into various aspects like data privacy concerns, technical limitations, and the necessity of human oversight. While automated intelligence presents substantial benefits, it also brings considerable risks and ethical dilemmas that must not be overlooked. Addressing these challenges is essential to ensure a responsible and effective implementation in various sectors.
Data Privacy Concerns
Data privacy is a significant issue within the domain of automated intelligence. The use of large datasets is essential for training algorithms effectively. However, this often involves collecting and processing personal information, which raises several concerns. With GDPR and other regulations, organizations must navigate strict laws governing data collection, usage, and retention.
A key point is that a lack of transparency in data handling practices could lead to a breach of trust between users and companies. If users do not understand how their data is used, it can result in hesitance or refusal to engage with automated systems. Thus, organizations must establish rigorous policies and transparently communicate practices to mitigate such concerns.
Moreover, breaches can lead to legal consequences and reputational damage, making data privacy not just a compliance issue but also a strategic element in the deployment of automated intelligence.
Technical Limitations
Automated intelligence is not without its limitations. Although tremendous strides have been made, various technical barriers still hinder its widespread adoption. For instance, many algorithms require significant computational power and resources, which can be a major hurdle for smaller organizations. Furthermore, the quality of output is heavily dependent on the quality of input data. Poor data can lead to inaccurate predictions which undermine trust in automated systems.
Some systems also struggle with understanding context. Natural language processing, while improving, is still prone to misinterpretation or failure to capture intent accurately. These shortcomings can limit the usefulness of automated intelligence in critical applications, particularly those requiring nuanced understanding.
Additionally, reliance on vendor-specific technology can create challenges in integration across different platforms. Interoperability remains a key hurdle if organizations wish to harness diverse automated intelligence tools.
Human Oversight Requirements
While automated intelligence can significantly enhance efficiency, it still relies on human oversight for optimal performance. This is important in ensuring accountability, especially in areas where the consequences of erroneous decisions can be severe, like healthcare or finance. Algorithms may make decisions based on patterns, but they lack the ability to fully understand human contexts and ethical implications.
Incorporating a human element can help mitigate risks associated with biases in algorithms. An algorithm trained on biased data can perpetuate and even amplify those biases in its decisions. Human oversight serves as a necessary check, allowing for a more balanced approach when deploying automated intelligence systems.
Moreover, continuous monitoring and assessment are essential. Regular evaluations can ensure that the systems adapt as new information becomes available and changes in the environment occur. Without this, there is a risk that automated solutions become outdated or misaligned with user needs.
"In the realm of automated intelligence, human oversight is not a hindrance but a fundamental necessity for ethical decision-making."
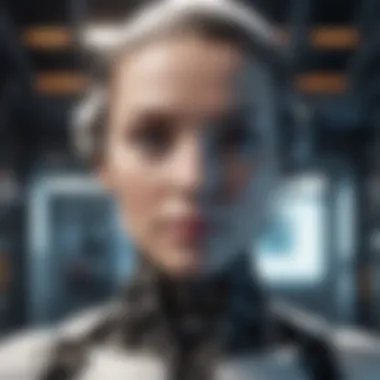
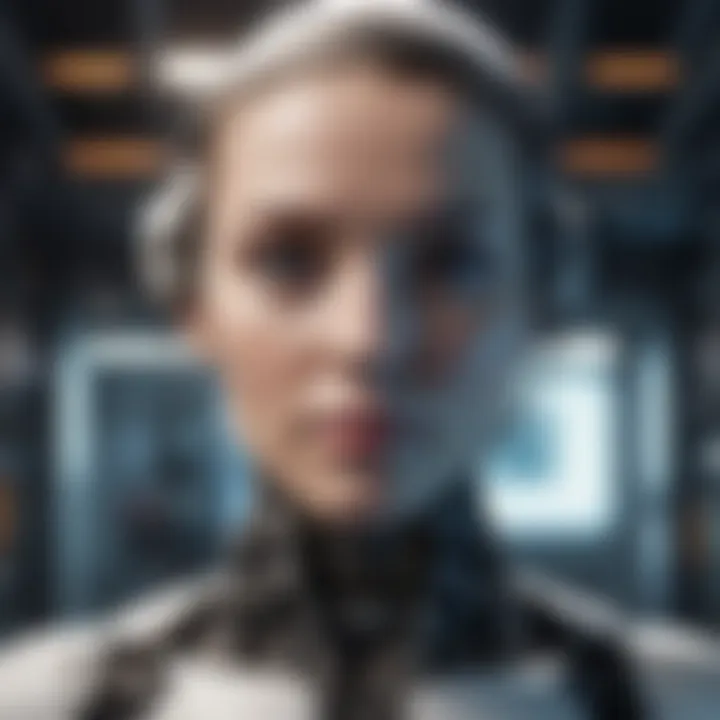
Overall, understanding these challenges and limitations provides valuable insights into how to deploy automated intelligence responsibly and effectively.
Ethical Considerations in Automated Intelligence
The role of ethics in automated intelligence cannot be overstated. As systems increasingly take on decision-making roles across various sectors, understanding ethical considerations becomes crucial. This helps to both shape technology development and safeguard society.
Bias and Fairness
Bias in automated systems arises for various reasons. Inadequate data, whether due to underrepresentation of certain groups or inherent biases in collected data, can lead to skewed results. An example can be seen in facial recognition algorithms. Studies show that these systems often misidentify individuals from minority groups at higher rates than others.
Fairness requires that automated intelligence systems are designed to be inclusive. Developers must prioritize diverse datasets, testing algorithms rigorously to identify biases before deployment. Long-term monitoring is equally important to adapt to social changes. Mitigating bias is not merely a technical challenge; it reflects deeper societal values. Fair decisions can lead to improved trust in automated systems and their applications in real-world scenarios.
Accountability in AI Decisions
Accountability in automated intelligence involves answering for the outcomes generated by algorithms. When an AI system makes a decision, understanding who is responsible for that action is imperative. This concern drives discussions about liability in various domains including healthcare, finance, and law enforcement.
The complexity of AI systems poses a challenge. Transparent processes for decision-making must be in place. Developers and organizations need to establish clear guidelines for accountability. This ensures that any flaws in the system can be addressed properly. Using explainable AI models can help elucidate how outcomes were reached, thereby increasing trust and making it easier to assign accountability in case of adverse effects.
Impact on Employment
The rise of automated intelligence is transforming the job landscape. Increased automation leads to enhanced efficiency but it can also result in job displacement. Routine tasks in sectors like manufacturing, retail, and even customer service are increasingly managed by automated systems. However, this change is not purely negative.
The adaptation to automated systems may create new roles that require different skill sets. Workers may need to transition into more analytical positions, focusing on managing and optimizing AI tools. Addressing this shift proactively through education and retraining programs is essential.
In summary, discussing the ethical dimensions of automated intelligence needs urgency. Bias and fairness, accountability for decisions, and the impact on employment are not just technical concerns but elements that define the future of society and technology. As automation continues to grow, ethical considerations must guide its implementation.
Future Trends in Automated Intelligence
Automated intelligence represents a rapidly evolving field, and understanding its future trends is crucial for staying ahead in technology. These trends illustrate the transformative potential this technology holds across industries. They reveal how integrated systems can optimize processes, enhance decision-making, and create innovative solutions to complex problems.
Integrating AI with the Internet of Things
The integration of artificial intelligence with the Internet of Things (IoT) will reshape how devices communicate and operate. IoT involves connecting physical devices to the internet, allowing them to collect and exchange data. However, adding AI enhances this capability significantly.
AI analyzes data gathered from IoT devices for patterns and insights. For instance, in smart homes, AI can adjust heating, lighting, and security settings based on user behavior or environmental conditions. In industrial settings, AI can predict equipment failures before they happen, enabling timely maintenance. This predictive capability minimizes downtime and extends the lifespan of machines, resulting in operational efficiency.
Moreover, the synergy between AI and IoT can facilitate smarter urban management. It can optimize traffic flow, reduce energy consumption, and enhance public safety through better data utilization. Real-time analytics is key in such applications, making cities more responsive to residents' needs.
Advancements in Cognitive Computing
Cognitive computing refers to systems that simulate human thought processes. These systems use AI and machine learning algorithms to process vast amounts of unstructured data, providing insights that can inform strategic decisions. The advancements in this area are paving the way for more intuitive human-computer interactions.
One significant development is improving natural language processing. Enhanced algorithms allow machines to understand and respond to human language more effectively. This can lead to more sophisticated virtual assistants and customer service applications, which can handle complex inquiries and tasks without human intervention.
Furthermore, cognitive computing applications extend to fields such as healthcare, where systems can analyze patient data for better diagnosis and treatment plans. They can also inform financial services by providing insights into market trends and consumer behavior. Therefore, cognitive computing is critical for unlocking the full potential of automated intelligence.
The Role of Quantum Computing
Quantum computing holds promise for revolutionizing automated intelligence through unparalleled processing power. Unlike classical computers, which process information in binary form, quantum computers utilize quantum bits, or qubits, enabling them to perform complex calculations at unprecedented speeds.
This advancement could significantly enhance areas such as machine learning and optimization. For instance, quantum algorithms can improve the efficiency of data analysis, making it possible to process vast datasets rapidly. It could lead to breakthroughs in complex problem-solving across industries, such as logistics, finance, and drug discovery.
However, the practical implementation of quantum computing in automated intelligence is still in early stages. Much research and development is necessary before it can be widely applied. Yet, its potential should not be underestimated, as it could define the future capabilities of automated systems.
"Quantum computing not only enhances speed but also enables the solution of problems previously deemed unsolvable."
In summary, the future trends in automated intelligence indicate a pathway toward more interconnected, intelligent, and efficient systems. The combination of AI with IoT, advancements in cognitive computing, and the emergence of quantum computing underscore a shift that could redefine how we live and work.
End: The Path Ahead
The topic of automated intelligence plays a significant role in shaping future technological landscapes. It is essential to understand how it integrates into various industries, from healthcare to finance. As we summarize the core components and applications of automated intelligence, we also recognize the profound influence it will have on our societal frameworks.
Summarizing Automated Intelligence
Automated intelligence essentially revolves around enhancing efficiency in decision-making processes. It encompasses the blend of data processing, machine learning, and decision-making algorithms. Each part contributes to creating advanced systems that learn from data and improve over time.
The evolution of automated intelligence shows a clear trajectory towards more integrated and intelligent solutions. The advancements in areas such as machine learning, natural language processing, and robotic process automation underscore this progress. These technologies, collectively, are reshaping how organizations operate and compete in the modern era.
Additionally, applications of automated intelligence are profound. For instance, in healthcare, it aids in diagnostic systems and personalized medicine, impacting patient outcomes. In finance, it enhances fraud detection and risk assessment, ensuring better security and trust in financial transactions. The reach of automated intelligence extends across numerous sectors, reflecting its versatile nature and productivity enhancements.
"The effective application of automated intelligence is crucial in improving operational efficiency across various businesses."
Emphasizing the Need for Responsible AI
With the increasing reliance on automated intelligence, responsible AI becomes paramount. This concept focuses on ethical guidelines, transparency, and accountability in AI decisions. As these systems start to impact lives, public trust hinges on their operations being fair and unbiased. Ensuring equitable algorithms and preventing discrimination must take precedence.
Moreover, accountability in AI decisions is vital. Organizations must be ready to explain the reasoning behind automated decisions, especially in sensitive areas like healthcare and law enforcement. This transparency fosters understanding and reinforces trust. Individuals should also understand how automated systems gather and process their data.
The impact on employment is another critical consideration that needs attention. As automated systems become more prevalent, they could replace certain job categories while creating new roles. Balancing the advantages of efficiency with the inherent challenges for the workforce highlights the need for responsible AI implementation.