Data Collection and Analysis Tools: An In-Depth Overview
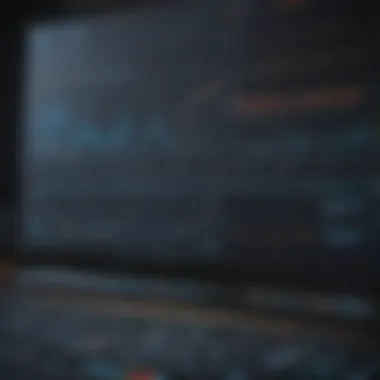
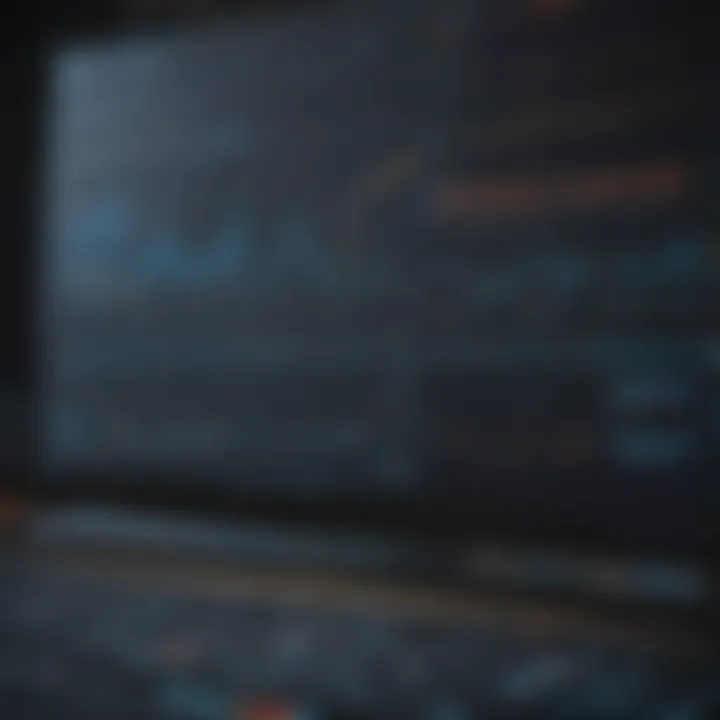
Intro
In today's fast-paced research environment, the reliance on data collection and analysis tools has reached new heights. Researchers in various fields, from social sciences to healthcare, increasingly recognize the necessity of employing robust methodologies to gather, process, and analyze data. Understanding these tools equips researchers with the ability to make informed choices that illuminate their findings and enhance the quality of their work.
Data collection and analysis are fundamental steps in the research process. They serve as the backbone for generating reliable results. The landscape of available tools continues to evolve, creating a diverse toolkit for practitioners. Traditional methods like surveys and interviews coexist with modern digital solutions, such as online analytics platforms. The choice of tool often hinges on the specific nature of the study and its objectives, making careful selection crucial for impactful outcomes.
Research Context
Background Information
The methods of data collection have undergone significant transformation over the years. Historically, researchers relied on face-to-face interviews and paper-based surveys. These traditional techniques are still relevant but are now often supplemented or replaced by digital means. Technologies such as mobile devices and cloud computing not only enable more efficient data collection but also enhance accessibility and reach.
Furthermore, the rise of big data has led to new approaches in analysis. The sheer volume and complexity of data can be overwhelming. Hence, researchers must utilize sophisticated tools that can manage large datasets effectively. The convergence of qualitative and quantitative analysis tools has also broadened the horizons, offering a more holistic understanding of research subjects.
Importance of the Study
The study of data collection and analysis tools is of paramount importance for several reasons. First, these tools influence the validity and reliability of the research. Choosing an inappropriate tool can lead to misleading results. Second, understanding the functionalities and limitations of these tools empowers researchers to adapt them to their unique requirements.
Moreover, as data becomes more integral in research, staying updated on tool advancements is vital. This comprehensive overview aims to guide researchers in navigating this ever-changing landscape. By providing insights into both traditional and modern tools, the study emphasizes practical frameworks that enhance research quality.
"The ability to choose the right data tools can be as essential as the methodologies employed in research."
As we proceed, discussions will focus on specific tools, their methodologies, and comparative effectiveness across various disciplines. This critical perspective will enable researchers to make astute decisions tailored to their research needs.
Prolusion to Data Collection and Analysis
Data collection and analysis stands as a critical component in research across disciplines. Understanding these processes equips students, researchers, educators, and professionals to make informed decisions based on empirical evidence. The capacity to gather and analyze data can dictate the relevance and success of research outcomes. In the rapidly evolving landscape of information technology, recognizing the appropriate tools becomes increasingly important.
Definition and Importance
Data collection refers to the systematic gathering of information, often with the goal of answering specific research questions. It can include a variety of methods, both traditional and digital. Analysis, on the other hand, involves interpreting the collected data to extract meaningful insights.
Understanding these concepts is paramount, as they influence the integrity and reliability of research findings. The quality of data directly affects conclusions and subsequent decisions derived from research. Therefore, researchers need to be adept in choosing suitable methodologies and tools that fit their specific needs.
Historical Context
The evolution of data collection and analysis can be traced back to ancient civilizations. Early societal structures relied on basic forms of counting and record-keeping for trade and resource management. These methods gradually transformed into more sophisticated techniques, supported by advances in mathematics and statistics. With the advent of computers in the 20th century, the realm of data collection and analysis underwent a significant transformation.
Today, a multitude of tools and methodologies exists, reflecting a blend of historical practices and modern technological advancements. This historical perspective emphasizes the ongoing development of practices which influences current approaches in research.
Types of Data Collection Methods
Data collection methods are essential for gathering the necessary information to drive research and analysis. Understanding these methods allows researchers to select the most suitable techniques for their specific needs. This section breaks down the primary types of data collection methods into three categories: qualitative, quantitative, and mixed methods. Each approach has unique features, advantages, and considerations that researchers should be aware of when designing their studies.
Qualitative Methods
Qualitative methods focus on collecting non-numerical data. They aim to understand the why and how behind behaviors, opinions, and experiences. These methods often involve open-ended questions, allowing participants to express their thoughts freely.
Common techniques in qualitative data collection include:
- Interviews: One-on-one discussions that provide in-depth insights into individual perspectives.
- Focus Groups: Group discussions that explore shared views and social dynamics.
- Observations: Directly watching participants in their natural environment can yield valuable context about their behaviors.
The benefit of qualitative methods is their ability to offer rich, detailed data that numerical methods may overlook. However, these methods can be time-consuming and often require careful analysis to identify key themes and patterns.
Quantitative Methods
Quantitative methods, in contrast, rely on numerical data that can be quantified and analyzed statistically. This approach is effective for identifying trends, testing hypotheses, and making predictions based on collected data.
Some common quantitative data collection techniques include:
- Surveys and Questionnaires: Structured tools that gather standardized information from a larger sample size.
- Experiments: Controlled studies that measure how specific variables influence outcomes.
- Existing Data Analysis: Utilizing pre-existing datasets to draw conclusions without direct data collection.
Quantitative methods are vital for yielding statistically significant results. They can help demonstrate relationships between variables and provide a foundation for generalization. Nevertheless, simply having numerical data does not guarantee that meaningful insights will emerge. Researchers must ensure proper design and implementation to avoid misleading conclusions.
Mixed Methods
Mixed methods combine both qualitative and quantitative approaches, allowing researchers to leverage the strengths of each. This technique can enhance the richness of data and provide a more comprehensive understanding of research questions.
Key attributes of mixed methods include:
- Triangulation: Using multiple methods to cross-validate data and findings.
- Complementarity: Qualitative data can enrich quantitative results by adding context, while quantitative data can test and validate qualitative insights.
- Flexibility: Researchers can adapt methods as needed, depending on the evolving nature of their study.
Utilizing mixed methods may require more resources and time, but it often leads to more nuanced findings. This approach aids in tackling complex research questions that cannot be fully understood through a single method alone.
In summary, selecting the appropriate data collection method is crucial for the success of any research project. Each type has its significance and applications, making it essential for researchers to be clear about their objectives and constraints before choosing a methodology.
Traditional Data Collection Tools
Traditional data collection tools are fundamental in the landscape of research methodologies. They form the backbone of systematic inquiry across various disciplines. This section details why these tools remain relevant, despite the emergence of digital alternatives. Furthermore, it elucidates the specific strengths and limitations of these tools, thereby providing insights for researchers aiming to select appropriate methodologies.
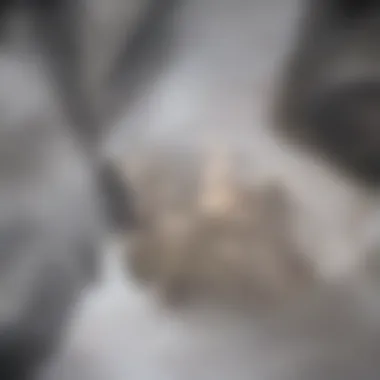
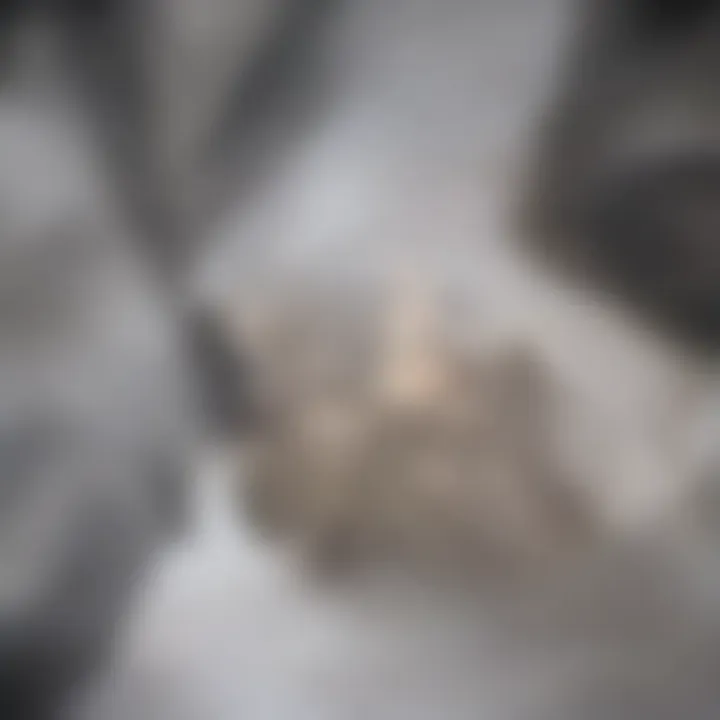
Surveys and Questionnaires
Surveys and questionnaires are perhaps the most widely used traditional data collection tools. They allow researchers to gather information directly from their target audience regarding opinions, behaviors, or characteristics. These tools have several benefits:
- Standardization: Surveys can be standardized, which allows for comparability across different responses.
- Scalability: They can reach a large and diverse sample quickly, making them efficient for quantitative research.
- Anonymity: Participants often feel more comfortable providing honest responses privately.
However, there are considerations to keep in mind. The design of the survey is crucial. Questions must be clear to avoid confusion, as poorly worded questions can lead to misinterpretation and biased results. Additionally, the mode of administration, whether it be online or paper-based, can impact the response rate and quality.
Interviews
Interviews offer a different approach to data collection, focusing on in-depth discussions rather than broad quantitative data. They can be structured, semi-structured, or unstructured, which gives flexibility based on research needs. The main benefits include:
- Rich Data: Interviews often provide more nuanced data, capturing a respondent's feelings and thoughts in detail.
- Adaptability: Researchers can explore topics further based on responses, allowing for a deeper understanding.
- Personal Interaction: The direct interaction can build rapport, leading to more genuine responses.
Nonetheless, interviews also come with challenges. They can be time-consuming to conduct and analyze. The interviewer's skills play a significant role in the quality of the data collected. Bias can unintentionally creep into the process, affecting neutrality and objectivity.
Focus Groups
Focus groups consist of guided discussions among a small group of participants. They provide qualitative insights that are particularly valuable when exploring new ideas or concepts. Focus groups have several distinct advantages:
- Interactive Dynamics: The group setting encourages participants to engage with each other, sparking ideas that may not emerge in individual interviews.
- Diverse Perspectives: They allow multiple viewpoints to surface, enriching the data collected.
- Immediate Feedback: Researchers can observe group dynamics and responses in real-time, facilitating responsive adjustments in the discussion.
However, focus groups can also pose difficulties. Dominant personalities may skew the conversation, leading to biased data. Additionally, analyzing focus group discussions may be complex due to the varied nature of responses.
Traditional tools like surveys, interviews, and focus groups remain invaluable, resonating with researchers seeking reliability and depth in their data collection processes.
Digital Tools for Data Collection
Digital tools for data collection have transformed how researchers gather and analyze information. In an increasingly data-driven world, these tools offer efficiency, accuracy, and accessibility that traditional methods struggle to match. Utilizing these tools can enhance the quality of research while streamlining workflows. They allow for quicker data gathering and often provide options for instant analysis, making them integral to modern research projects across various disciplines.
The primary aim of digital tools is to simplify the data collection process. They can capture responses with speed and accuracy, reducing human error significantly. Moreover, many digital tools provide real-time data analysis capabilities, allowing researchers to assess the information as it comes in. This affords opportunities for immediate adjustments in research strategies or methodologies if required. The versatility in digital tools means they can accommodate various data types, from numeric to text data, adapting to the nature of the research being conducted.
Despite their advantages, researchers must consider several factors when selecting digital tools for data collection. These include compatibility with existing systems, ease of use, and the ability to ensure data security and privacy. A well-rounded approach in choosing these tools will ultimately enhance the quality and reliability of the data collected.
Online Surveys
Online surveys are one of the most prevalent methods for digital data collection. Platforms like SurveyMonkey and Google Forms have made it easy for researchers to design, distribute and analyze surveys. The significant advantage of online surveys is their reach; they can distribute surveys to a vast audience quickly and efficiently. Participants can complete surveys at their convenience, which often increases response rates.
Designing an effective online survey requires careful consideration. Researchers need to craft clear and engaging questions that do not lead or bias responses. Furthermore, employing skip logic can make surveys more user-friendly, allowing respondents to navigate questions relevant to their experiences.
"Online surveys are not just about gathering data; they can also offer insights into participant behavior and preferences."
Thus, they are an invaluable tool in both qualitative and quantitative research.
Mobile Data Collection Apps
Mobile data collection apps have gained popularity as smartphone usage increases worldwide. Apps can facilitate real-time data entry and ensure that data is gathered in the field efficiently. Tools such as KoBoToolbox or OpenDataKit allow researchers to collect data directly from their mobile devices without needing intermediate steps. This approach can reduce lag time and enhance the accuracy of data collected during fieldwork.
The usability of these apps is vital for effective data collection. They should be easy to navigate, ensuring that data entry personnel can complete their tasks without significant training. Furthermore, features like offline data collection capability are important for researchers working in remote areas without consistent internet access.
Data synchronization capabilities are another critical factor, enabling researchers to update and access data in real-time once an internet connection is available.
Web Scraping Tools
Web scraping tools have grown in prominence as researchers seek to extract valuable data from online resources. Programs like Beautiful Soup for Python or Octoparse can automate the process of gathering information from various websites. This automation is particularly useful for compiling large datasets from multiple sources without manual entry.
Using these tools comes with its challenges. Researchers must navigate legal and ethical considerations, ensuring they collect data responsibly and in compliance with regulations, such as GDPR in Europe. Additionally, understanding the structure of websites and how to program the tools to scrape desired information can require a steep learning curve.
Nonetheless, once mastered, web scraping can significantly enhance a researcher's ability to collect vast amounts of data that would be challenging to obtain through traditional methods.
Data Analysis Tools Overview
Data analysis tools are indispensable in the landscape of research, offering methodologies that facilitate the transformation of raw data into meaningful insights. Having knowledge about these tools aids researchers in navigating the complexities involved in data analytics. Each category of tools serves unique purposes, addressing the diverse needs of analysis across various fields.
The significance of data analysis tools comes down to their ability to enhance decision-making, reveal trends, and support hypotheses through rigorous examination of data. Tools can range from statistical software to more visual instruments, allowing users to interpret findings visually. The right analysis tools can boost the productivity of researchers while ensuring data integrity.
Statistical Analysis Software
Statistical analysis software is primarily used for quantitative research. Programs such as SPSS and R provide a robust framework for data analysis with functionalities that accommodate various statistical tests. Their strength lies in handling large datasets and performing complicated calculations efficiently.
Researchers benefit from these tools in several ways:
- Ease of Data Handling: Work with large volumes of data without losing accuracy.
- Complex Statistical Tests: Execute advanced analyses, which would be labor-intensive to perform manually.
- Reporting Features: Generate detailed reports that can be easily shared with stakeholders.
An important consideration when choosing statistical software is the learning curve associated with it. Some software may require specific proficiency in programming or statistics, adding a layer of complexity to the research process.
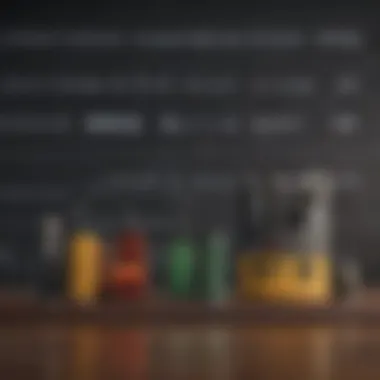
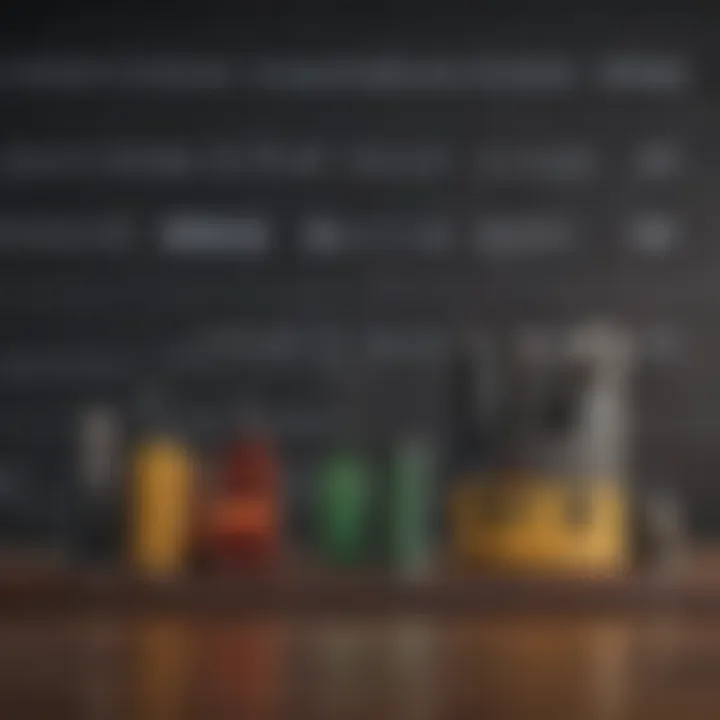
Data Visualization Tools
Data visualization tools are vital for depicting data in easily digestible formats. These tools transform raw data into charts, graphs, and other visual aids that enhance the understanding of patterns and trends. Programs like Tableau and Microsoft Power BI can significantly elevate presentations of data analysis.
Benefits of using data visualization tools include:
- Improved Communication: Visuals can convey complex data much more clearly than text.
- Identification of Trends: Easier spotting of trends, outliers, and correlations.
- User Engagement: Engaging visuals can prompt discussions and stimulate further inquiry.
While data visualization tools provide compelling representations, it is essential to maintain accuracy and avoid misleading visuals. Researchers must validate their data to ensure their visualizations accurately reflect their findings.
Qualitative Data Analysis Software
Qualitative data analysis software serves a distinct purpose, focusing on non-numeric data such as interviews and textual datasets. Software like NVivo and MAXQDA facilitate the coding and systematic analysis of qualitative data. These tools help in uncovering themes or patterns that can be instrumental in researchersβ conclusions.
Some advantages of qualitative data analysis software include:
- Streamlined Data Organization: Efficiently categorize and retrieve qualitative data.
- Enhanced Analytical Framework: Tools provide frameworks that guide thorough analysis.
- Collaboration Opportunities: Many platforms support teamwork, enabling multiple researchers to contribute to analysis simultaneously.
However, selecting the appropriate tool can be challenging. Considerations should encompass the specific research needs, the type of qualitative data being examined, and the intended outcome of the analysis.
Popular Data Analysis Software
The realm of data analysis is enriched by various software tools that serve distinct purposes and cater to diverse user needs. Popular data analysis software not only facilitates the interpretation of data but also enhances the efficiency of research processes. Understanding these essential tools allows researchers, students, educators, and professionals to choose the right applications that align with their analytical objectives. By being familiar with this software, users can optimize their research outcomes, ensuring precise and effective data insights.
SPSS
SPSS, or Statistical Package for the Social Sciences, is among the most widely recognized statistical software applications. Its engagement in social science research has made it an invaluable tool for both novices and experts. The software provides a user-friendly graphical interface that simplifies complex data analysis tasks. Researchers appreciate the various statistical tests and algorithms it offers, such as regression analysis, ANOVA, and factor analysis.
However, SPSS also has its drawbacks. Licensing can be expensive, which may not be feasible for all individuals or organizations. Moreover, those who require advanced customization or programming capabilities might find it limited compared to other solutions. Nevertheless, the ease of use of SPSS proves beneficial for quick and effective statistical analysis.
R and RStudio
R is an open-source programming language specializing in statistical computing and graphics. It is loved for its flexibility and the vast collection of packages available. When paired with RStudio, a powerful integrated development environment, the analysis process becomes even more efficient. R permits intricate data manipulation and visualization, making it highly appealing for researchers who require tailored solutions for specific projects.
While R has a steep learning curve, especially for users without a programming background, its community support mitigates this challenge. Numerous forums, including those on Reddit, provide assistance and resources. Furthermore, R is free, making it accessible for many users who might find SPSS too costly. Despite some initial hurdles, R and RStudio are undoubtedly powerful assets in the toolkit of data analysts.
Python for Data Analysis
Python is a versatile programming language that has gained traction in data analysis due to its simplicity and readability. Libraries such as Pandas and NumPy offer robust tools for data manipulation, while Matplotlib and Seaborn allow for effective data visualization. This combination makes Python appealing across various fields, especially in academia and industry.
Incorporating Machine Learning capabilities into analysis is another significant advantage of Python. With libraries like Scikit-learn and TensorFlow, researchers can not only analyze data but also extract valuable predictive insights. Despite Python's wide-ranging capabilities, newcomers may find aspects like library management somewhat daunting. Yet, as they familiarize themselves with the language, it can create pathways to more complex data analysis tasks.
"Python's versatility positions it as a top choice for data analysis in both academic and industrial settings."
Choosing the Right Data Tools
Selecting the appropriate data collection and analysis tools is pivotal for the success of any research project. The right tools can enhance the quality of data gathered, streamline the analysis process, and ultimately contribute to more reliable conclusions. With the ever-evolving landscape of technology, researchers face numerous options, each boasting unique features and capabilities. Thus, understanding these features, assessing specific research needs, and being mindful of budgetary constraints are essential steps in the decision-making process.
Assessing Research Needs
Before diving into the array of available data tools, researchers must first evaluate their own needs. This assessment can take many forms. It is important to clearly define the research objectives, the type of data required, and the method of analysis applicable to the project. For example, qualitative research might prioritize tools that excel in managing unstructured data, whereas quantitative studies may demand robust statistical capabilities.
Additionally, researchers should consider the target audience and the context in which the findings will be presented. Tools must not only facilitate data gathering but also allow for effective communication of results. Engaging with peers to understand past experiences with specific tools can provide invaluable insights.
Evaluating Tool Features
Once the research needs have been established, the next step is to evaluate tool features. Different tools serve various purposes, and understanding their functionalities enhances selection precision. Here are some critical features to consider:
- User-friendliness: The interface should allow easy navigation, minimizing the learning curve for all users.
- Integration capabilities: Tools should seamlessly integrate with existing systems and other software to ensure a smooth workflow.
- Data security: Protection of sensitive information should be a priority. Assess each toolβs data management and security protocols.
- Customizability: The ability to tailor the tool to specific project requirements can improve effectiveness.
A thorough comparison of features among potential tools is advisable, as it can illuminate the strengths and weaknesses of each option.
Budgetary Considerations
Finally, budgetary constraints cannot be overlooked. Research projects often have limited funding, and selecting tools that provide value for money is crucial. Several aspects require careful reflection:
- Initial costs: Evaluate the price of the software or tool, including any additional setup fees.
- Recurring expenses: Consider whether the tool operates on a subscription basis and any costs associated with updates or additional features.
- Hidden costs: Factor in costs that might arise from training, technical support, and potential extra installations needed for effective use.
Researchers must balance cost with functionality, ensuring they do not compromise on essential features that impact data quality and analysis.
"Thorough planning and consideration of needs, features, and costs lead to more effective tool selection and better research outcomes."
By taking these considerations into account, researchers can make informed decisions that align with their project goals and financial constraints.
Challenges in Data Collection and Analysis
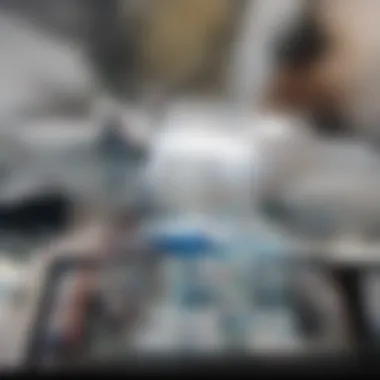
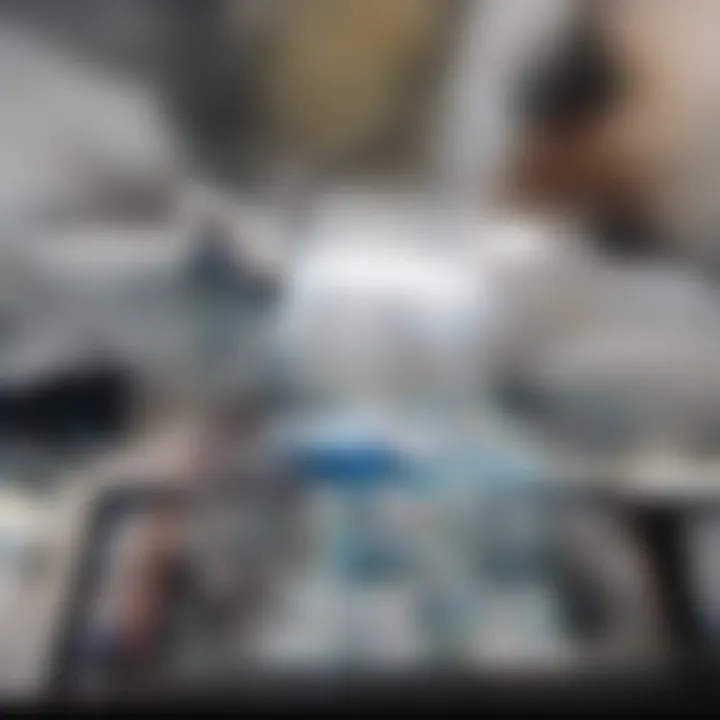
Understanding the challenges in data collection and analysis is crucial for any research endeavor. These obstacles can significantly impact the reliability and validity of the dataset, which ultimately affects the conclusions drawn from the analysis. Addressing these challenges is essential for ensuring that the data collected accurately represents the phenomena being studied. Key areas of concern include data quality issues, ethical considerations, and data security concerns. By recognizing and managing these challenges, researchers can enhance the quality of their findings and contribute to their respective fields more effectively.
Data Quality Issues
Data quality is fundamental in research. Poor quality data can lead to incorrect conclusions and misguided recommendations. Inconsistent data collection methods, missing data, and biases in data sourcing or entry are common issues that researchers face.
- Inconsistency: Different methods across studies can yield results that are not comparable. Varying definitions of variables may lead to confusion and misinterpretation.
- Missing Data: This occurs for various reasons, such as non-response in surveys or errors during data entry. Missing information can skew results and make analyses less reliable.
- Bias: Both selection and response bias can compromise data quality. For instance, if only a small group of people participate in a survey, the results may not reflect the larger population's views.
To mitigate these issues, researchers should:
- Select data collection methods carefully to ensure consistency.
- Plan for missing data, considering strategies such as imputation to fill gaps.
- Be mindful of potential biases and use techniques to minimize their impact.
Ethical Considerations
Ethics in data collection is paramount. Researchers must prioritize the integrity and rights of participants. Violations can lead to trust issues, legal penalties, and damage to reputations. Key ethical considerations include:
- Informed Consent: Participants must understand the nature of the study and consent to participate willingly.
- Anonymity and Confidentiality: Protecting personal information is critical. Researchers should implement stringent measures to safeguard participant identities.
- Data Integrity: Researchers should report findings honestly, maintaining transparency in methodology and potential conflicts of interest.
Adhering to ethical standards not only enhances the credibility of the research but also fosters a respectful relationship between researchers and participants.
Data Security Concerns
As data becomes increasingly digital, the risk of security breaches also rises. Protecting data, especially sensitive information, is essential to prevent exploitation. Key areas of concern include:
- Unauthorized Access: Cybersecurity threats can lead to data theft or manipulation. Therefore, robust security measures must be in place.
- Compliance with Regulations: Researchers must be aware of regulations like GDPR, which govern data protection. Failure to comply can lead to severe repercussions.
- Data Backup: Regularly backing up data is crucial in avoiding loss. This ensures continuity in research even in case of data corruption or loss.
For further insights, see relevant sources on Wikipedia and Britannica.
Future Trends in Data Tools
The landscape of data collection and analysis is continuously evolving. Staying abreast of future trends is essential for researchers and professionals. The integration of advanced technologies reshapes how data is processed and understood. This section articulates the significance of these trends, emphasizing Artificial Intelligence, Big Data technologies, and Cloud-based solutions.
Artificial Intelligence in Data Analysis
Artificial Intelligence (AI) plays a pivotal role in modern data analysis. The capabilities of AI allow for high-level processing of large datasets. Algorithms can identify patterns and trends that might be invisible to human analysts. This efficiency improves decision-making across various fields, including medicine, marketing, and social sciences.
Benefits of utilizing AI include:
- Automation: Reduces the time needed for manual analysis, allowing researchers to focus on strategic decisions.
- Predictive Analytics: AI tools can forecast future trends based on historical data, enhancing planning and resource allocation.
- Enhanced Accuracy: Machine learning algorithms minimize human error and bias in data interpretation.
However, some considerations must be taken into account. Data privacy issues arise, as AI often requires access to sensitive information. Ensuring robust ethical guidelines and regulations is critical for responsible AI deployment.
Integration of Big Data Technologies
Big Data technologies are indispensable in managing and analyzing vast amounts of data. These tools facilitate the extraction of valuable insights from diverse datasets. Their capacity to handle data at scale has been transformative for industries such as finance, retail, and logistics.
Key elements of Big Data integration include:
- Storage Solutions: Systems like Hadoop and Apache Spark provide efficient frameworks for data storage and processing.
- Real-time Analysis: The ability to analyze data in real-time aids in swift decision-making.
- Data Variety: Big Data technologies can gather structured and unstructured data, revealing comprehensive insights.
Nevertheless, businesses must navigate their investment in these technologies. Training staff and ensuring infrastructure support are essential steps for successful implementation.
Cloud-based Data Solutions
Cloud-based data solutions present a versatile approach to data collection and analysis. They offer researchers flexible, scalable access to resources without the need for extensive hardware investments. The cloud enables teams to collaborate seamlessly from various locations.
Core advantages include:
- Cost Efficiency: Reduces the need for physical servers and maintenance, allowing budget allocation to other research areas.
- Scalability: Easily adjusts to growing data needs, accommodating fluctuations in project scope or scale.
- Accessibility: Users can access data anytime, anywhere, provided there is an internet connection.
In contrast, organizations must also be cautious about data security. Potential vulnerabilities in cloud systems necessitate robust security measures to protect sensitive information.
In summary, understanding future trends in data tools is critical for successful research. Monitoring advancements in AI, Big Data technologies, and cloud solutions can help professionals adapt to the ever-changing data landscape.
Ending
The conclusion of this article serves as a crucial summation of the insights and knowledge gained throughout the discussion on data collection and analysis tools. Understanding the various tools available is not merely an academic exercise; it is fundamental for effective decision-making in research and business practices. Each tool has its unique strengths and weaknesses, which influence the outcomes of analyses and the reliability of findings.
Summary of Key Points
The article addressed numerous crucial elements concerning data collection and analysis tools. Here are the key points:
- Diverse Methods: Data can be collected through various methods like surveys, interviews, and digital tools, each providing different levels of detail and insight.
- Tools for Analysis: Tools such as SPSS and Python were discussed for their particular applications in statistical analysis and data processing.
- Choosing Wisely: Emphasis was placed on assessing research needs, tool features, and budget when selecting the right tools for any project.
- Challenges: Data quality, ethical considerations, and security concerns highlighted the complexities that come with data handling.
- Future Trends: Trends including artificial intelligence and cloud-based solutions indicate the evolving landscape of data tools.
These points generate an understanding of the key considerations when working with data, emphasizing a strategic approach to tool selection and application.
Final Thoughts on Data Tools
"In the era of information, understanding how to collect and analyze data effectively is not just a requirement but a necessary skill for success in research and industry."
Overall, investing time in understanding the spectrum of data tools allows one to navigate complexities efficiently and transform data into actionable insights.