AI Recommendations: Understanding Intelligent Suggestions
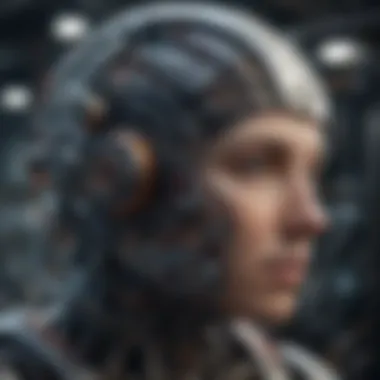
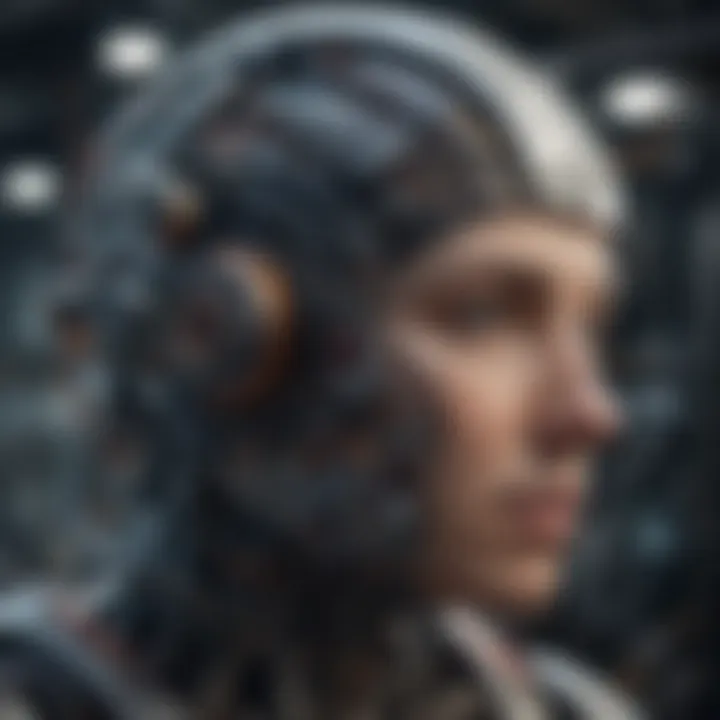
Intro
Navigating the ever-evolving world of technology, it's hard not to bump into AI recommendations. These digital nudge systems influence our choices, from what to watch on streaming platforms to products we consider purchasing online. They seem to be everywhere, quietly shaping our decisions behind the scenes.
But what really lies beneath these intelligent suggestions? How do algorithms determine our preferences? And what ethical dilemmas arise when these technologies are deployed throughout various sectors of society?
As we delve into these issues, we'll unpack the science behind these recommendation systems, exploring how they operate and their implications for user experience. This journey not only sheds light on the current landscape but also offers a glimpse into the future of AI recommendations and their potential impact on myriad industries.
Research Context
Background Information
The concept of AI recommendations has its roots deep in data analysis and machine learning. These systems rely on vast amounts of data to glean insights about user preferences. Historically, they began as simple filters, suggesting items based on explicit user behavior or input. Over time, however, they have morphed into complex algorithms capable of analyzing patterns and trends across millions of users almost instantaneously.
Consider Netflix's recommendation engine, which analyzes viewing history and then predicts what shows or movies a user might enjoy next. By learning from the audience's collective behavior, they provide a tailored experience that goes beyond mere guesswork. Yet this advanced approach also raises an eyebrow or two regarding privacy and the manipulation of choices.
Importance of the Study
Understanding AI recommendations is crucial, especially in todayβs fast-paced digital climate. With an increasing reliance on these systems, comprehending their mechanics and implications transcends mere curiosity; it becomes an essential skill for researchers and professionals alike. Decisions driven by these systems often can impact economic landscapes and even cultural trends.
The ethical concerns regarding data usage and recommendation biases must be explored thoroughly. For instance, if an algorithm suggests only one type of product due to pre-set biases, it could not only affect sales but also limit consumer experiences. This understanding helps professionals to navigate the landscape more effectively and encourages dialogue about the ethical framework in which these algorithms operate.
Discussion
Interpretation of Results
Diving deeper into these technologies, one finds that the results of AI recommendations are both promising and perplexing. They can create a personalized experience that delights users. However, there's a growing concern about the potential echo chambers they create. When users are consistently exposed to similar genres or styles, the risk of homogenized tastes arises. This leads to societal implications that extend far beyond the screen into art, culture, and even political discourse.
Comparison with Previous Research
Reflecting on traditional marketing methods, AI recommendations represent a seismic shift. Earlier approaches relied heavily on demographics and broad consumer segments. Now, we see learning algorithms that adapt in real-time, customizing interactions based on individual preferences and behaviors. This evolution prompts a reevaluation of how businesses engage with their customers. Indeed, studies have shown that businesses leveraging advanced recommendation systems often experience a higher rate of customer retention and satisfaction compared to those relying on outdated marketing tactics.
As we continue to investigate this field, it will be integral to compare findings across various studies and adapt best practices accordingly. Itβs this adaptability that will shape the future landscape of intelligent suggestions.
Understanding AI Recommendations
AI recommendations play a pivotal role in todayβs digital landscape, shaping the way we interact with technology and information. From shopping to consuming media, personalized suggestions have become almost second nature in our everyday lives. In this part of the article, we aim to unravel the significance of understanding AI recommendations. This comprehension is not just vital for tech enthusiasts but equally important for consumers, researchers, and industry stakeholders.
Definition and Scope
The concept of AI recommendations encompasses systems designed to analyze user preferences and behaviors to suggest relevant products, services, or content. At its core, AI recommendations utilize complex algorithms to process vast amounts of dataβranging from purchase history and user ratings to browsing habits. For instance, the recommendations on platforms like Netflix are not merely random selections but instead result from algorithms that scrutinize user viewing patterns and the similarities among different shows.
The scope of AI recommendations expands beyond entertainment into vital sectors such as e-commerce, healthcare, and even education.
- E-commerce: Platforms like Amazon employ sophisticated recommendation engines that not only suggest similar items but also predict what users might want next based on their shopping journey.
- Healthcare: In wellness applications, AI can suggest lifestyle changes or treatments based on user input regarding health metrics.
- Education: Online learning platforms utilize recommendations to guide learners to courses that match their previous activities and preferences.
In contrast to traditional recommendation systems, which might rely solely on prior choices or demographic information, AI recommendations harness machine learning to continuously improve. They adapt to evolving user preferences, making them increasingly relevant.
The Evolution of Recommendation Systems
Understanding the evolution of recommendation systems is crucial to comprehending their current capabilities. Historically, recommendation systems began with simple rules driven by user behavior. Early on, these systems mainly focused on collaborative filtering, where recommendations were based on what similar users liked. However, limitations arose as they struggled to suggest content for new users or items without a prior history.
The dawn of machine learning marked a turning point. As AI technologies matured, so did recommendation systems. With the introduction of neural networks and deep learning algorithms, methods became more refined.
- Past Decades: Initially limited to basic statistical techniques, the systems relied heavily on user ratings and preferences.
- Recent Advances: Todayβs systems leverage massive datasets and employ deep learning to understand complex user interactions. They can analyze text, images, and even sentiments, improving recommendation accuracy.
To illustrate:
"Imagine trying to find a needle in a haystack; modern recommendation systems serve up that needle on a silver platter while also filtering out the unnecessary hay."
As a result, the evolution of recommendation systems not only highlights technical improvements but demonstrates a growing understanding of consumer psychology.
In summary, the journey from simple algorithms to advanced AI-powered solutions underscores the necessity of understanding AI recommendations. This knowledge not only empowers users to navigate their choices more effectively but also arms businesses and developers with insights into enhancing user experience. Understanding AI recommendations is a key to unlocking the full potential of an increasingly digital world.
Types of AI Recommendation Methods
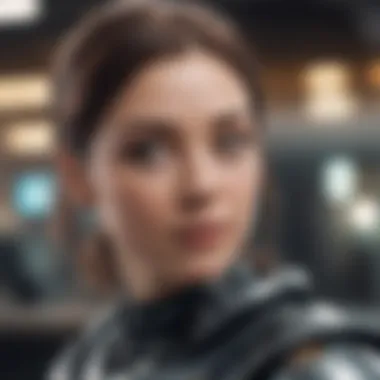
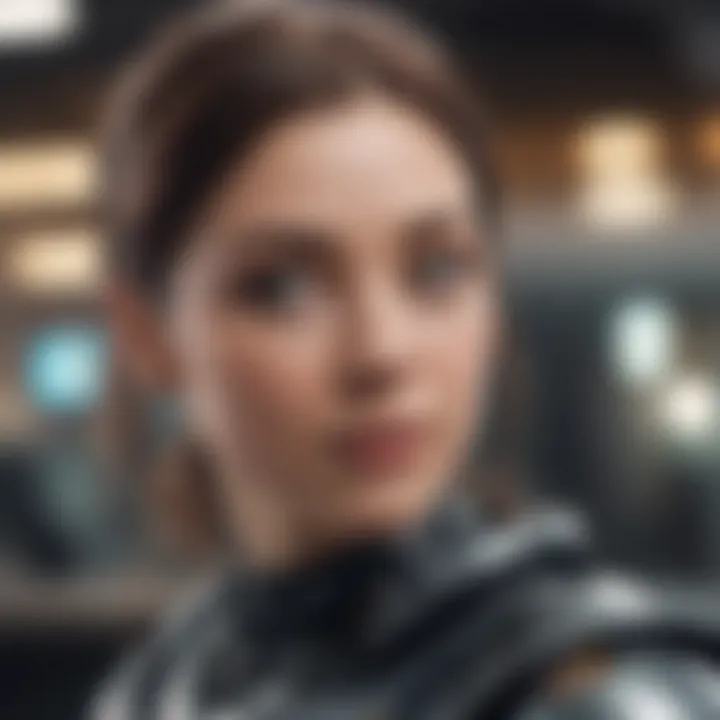
When it comes to AI recommendations, understanding the methods behind them is key for anyone engaged in this field. Each method has its unique benefits and considerations, impacting not only how recommendations are made but also how users interact and respond to them. As we plow through the dynamics of content presentation, user engagement becomes paramount. People want suggestions that resonate with their tastes, preferences, and previous interactions. Thus, the ways AI systems approach recommendations can define user satisfaction and engagement.
Content-Based Filtering
Content-based filtering is a method that suggests items similar to ones a user has liked in the past. The foundation here revolves around understanding the features of items, whether that's a movie's genre, an article's keywords, or a product's attributes. By analyzing these characteristics and the user's previous interactions, the system can offer suggestions that align closely with the user's tastes.
For instance, if someone enjoys mystery novels, the algorithm will examine the attributes of those novelsβsuch as the author's writing style, themes, and characters. The system then identifies similar works that share these features. The beauty of content-based filtering is that it thrives on personal preference while offering tailored suggestions without needing a vast base of user data. However, a potential pitfall here is the so-called "filter bubble," where users might miss out on diverse recommendations outside of their established preferences.
Collaborative Filtering Techniques
Collaborative filtering takes a different approach. Instead of focusing solely on the content of items, it taps into the actions of a broader user base. This technique posits that if two users have a similar history of liking certain products or media, they might also appreciate similar new items. The concept is grounded in the notion of communityβif your tastes align with another user, chances are youβll enjoy what they like.
This can manifest in two ways:
- User-User Collaborative Filtering: This involves finding users with shared interests and suggesting items based on what similar peers liked.
- Item-Item Collaborative Filtering: Here, the recommendation engine determines a similarity score between items based on user interactions, suggesting items that other users with similar tastes have enjoyed.
While effective, this method does face challenges, such as the cold start problem. When a new item enters the system, it may struggle to get recommendations initially due to the lack of user interest data. Nevertheless, collaborative filtering remains a cornerstone of recommendation strategies in platforms like Netflix and Amazon.
Hybrid Approaches
Hybrid approaches seek to mitigate the shortcomings of both content-based and collaborative filtering. By merging the two, these systems can offer more accurate and satisfying recommendations. For example, combining historical user data alongside item content can enhance the relevance of suggestions.
Consider a scenario where a user enjoys action movies and is also shown highly-rated action films that may not have matched their past tastes but have received glowing reviews from similar users. This offers an enriched experience, striking a balance between personalized recommendations and broader choices.
Hybrid systems can implement various strategies, such as:
- Weighted Hybridization: Combining recommendations from different systems with varying weights to curate a final suggestion.
- Switching Hybridization: Using different methods based on available data.
"The beauty of a hybrid recommendation approach lies in its flexibility and adaptability, catering to user needs and broadening their horizons."
In summary, each AI recommendation method provides a unique lens into understanding user preferences. Content-based filtering excels in personalization, collaborative filtering harnesses community insight, and hybrid approaches create a comprehensive toolkit to bridge gaps in traditional methods. As these techniques evolve, they will shape how people engage with technology and content in meaningful ways.
Key Algorithms Behind AI Recommendations
Understanding the algorithms that power AI recommendations is crucial for grasping the nuances of how these systems operate. At their core, these algorithms analyze user data to make suggestions that feel personal and relevant. They enhance user experience by ensuring that presented choices align with individual preferences, bolstered by vast amounts of data. The importance lies not only in the accuracy of predictions but also in building trust with users who rely on these systems to guide their choices in various fields, from shopping to healthcare. That's where comprehension of these algorithms becomes indispensable for educators, students, and professionals alike.
Machine Learning Fundamentals
Machine learning serves as the backbone of AI recommendations, enabling systems to learn from data rather than being explicitly programmed. It generally includes supervised, unsupervised, and reinforcement learning techniques. In supervised learning, the algorithm is trained on a labeled dataset, which means it is fed examples of input-output pairs. This allows the model to predict outcomes for new, unseen data based on learned patterns.
For instance, if you frequent an online bookstore like Amazon, the recommender system utilizes your browsing and purchasing history. It applies machine learning to find correlations between what you've bought and what other users with similar tastes have purchased. This algorithm is not only efficient but learns and adapts over time, constantly refining its predictions as it gathers more data.
Deep Learning Applications
Deep learning comes into play when tackling larger datasets with complex patterns. It uses neural networks that parallel the way human brains process information. This approach is particularly powerful for tasks such as image and voice recognition.
In the realm of recommendations, deep learning can find hidden relationships that traditional methods might overlook. For example, streaming services, like Netflix, leverage deep learning algorithms to analyze viewership behavior. They can identify not just what you watched, but also the nuanced preferences for themes, actors, or genres, allowing for richer recommendations.
"Deep learning not only forecasts user preferences but can also discover entirely new genre-blending categories that users might not even realize they enjoy."
Natural Language Processing in Recommendations
Natural Language Processing (NLP) plays a pivotal role in contextualizing recommendations. It allows systems to understand and interpret human language, enabling nuanced interactions that incorporate user reviews, preferences, and queries.
With NLP, AI can analyze massive sets of user-generated content, such as customer feedback and product descriptions. This content fuels algorithms to identify trends and sentiments within specific products or services. For instance, e-commerce platforms can employ NLP to sift through product reviews, emphasizing features that users frequently praise or criticize. This analysis can lead to enhanced recommendations based on what real customers appreciate the most.
Moreover, conversational agents, like those found in customer service on websites such as Facebook, make use of NLP to provide personalized suggestions based on user inquiries in natural language, effectively bridging the gap between human-language simplicity and mathematical complexity.
In essence, a robust understanding of these algorithms shines a light on how AI recommendations can profoundly alter the landscape of decision-making and personalization across various sectors.
The Impact of AI Recommendations on User Behavior
Understanding how AI recommendations influence user behavior is crucial in today's data-driven world. These systems play a pivotal role in how users make decisions, guiding them towards products, services, and content that they might not have discovered otherwise. As businesses leverage these tools more and more, comprehension of their impact is not just academic; itβs practical.
The mechanisms behind AI recommendations hinge on a delicate balance between personalization and user engagement, creating a dynamic interaction between the user and the machine. As we peel back the layers of this interaction, it becomes clear that at the heart of effective AI recommender systems lies deeply entrenched algorithms that adapt and respond to individual preferences.
"The ability for an AI system to tailor suggestions based on user behavior is a game changer in enhancing user experience."
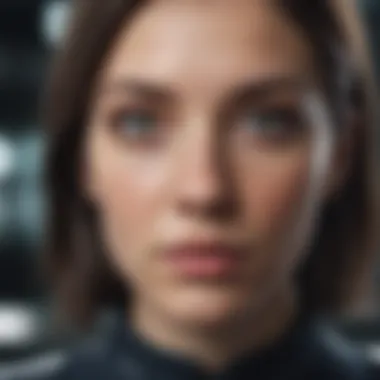
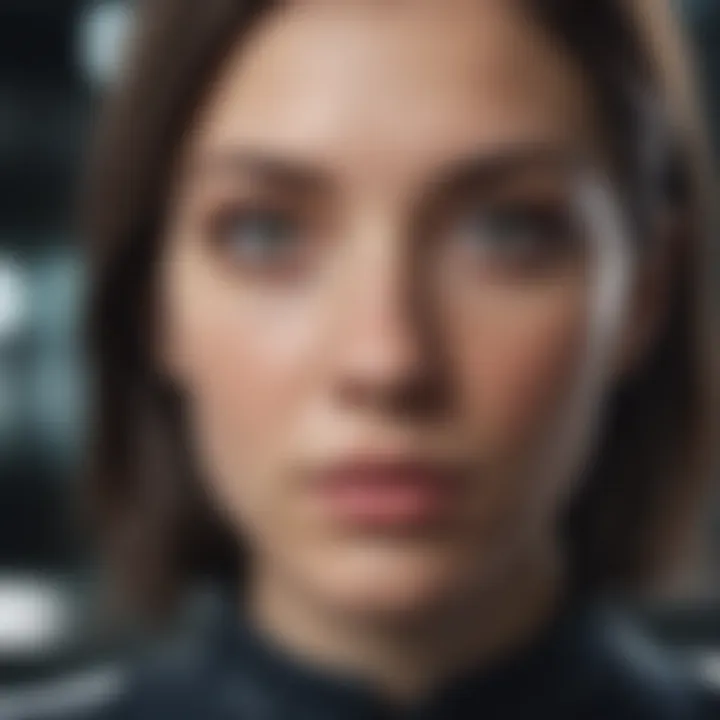
Personalization and User Engagement
Personalization in AI recommendations goes beyond just delivering content; it is about transforming user experience to make it relevant and enjoyable. The beauty of effective personalization rests in its subtleties. Think about how Netflix remembers your viewing history and suggests new shows you might like based on that. This meaningful tailoring encourages users to engage with the content deeply because it feels handpicked for them.
When a user sees a recommendation that resonates with their interests, it sparks a sense of connection. This can lead to increased time spent on platforms, as users feel more inclined to explore whatβs presented to them. However, this does raise important questions about reliance on algorithms. Are users losing their autonomy in decision-making? Will they only consume what is suggested?
Evaluating User Satisfaction
Assessing user satisfaction in relation to AI recommendations often involves analyzing two key factors: the accuracy of recommendations and the overall user experience. When users receive suggestions that align with their preferences, it tends to heighten feelings of satisfaction and loyalty. This aspect is vital for platforms such as eBay or Amazon where the consumer's path to purchase is meticulously tracked and refined through experience.
A dissatisfied user may result from irrelevant recommendations or an overwhelming number of options, leading to decision fatigue. On the other hand, if the recommendations feel spot on, users are likely to return not only to seek solutions but also to share their findings with others. Thus, a cycle of positive reinforcement is established, benefitting both users and businesses alike.
- Key elements to consider for evaluating user satisfaction include:
- Relevance of recommendations
- Usability of the platform
- Feedback mechanisms for continuous improvement
Influence on Consumer Choices
AI recommendations have a notable impact on consumer choices, often subtly steering users towards particular products or services. This influence is particularly evident in e-commerce, where consumers may purchase items they had no initial intent of buying. Think about the βCustomers who bought this item also boughtβ section on Amazon.
This type of recommendation can create a cascading effect, leading users to explore niches they may not have considered. While this boosts sales, it also necessitates ethical considerations. Companies must not exploit consumer tendencies negatively; instead, they should enhance the shopping experience without overwhelming users with choices.
AI recommendations indeed shape user behavior in profound ways. From personalizing experiences to directly influencing consumer choices, these intelligent systems craft an intricate web of interaction that becomes more robust with each passing on-line engagement. As the landscape evolves, ongoing evaluation of these impacts remains essential to ensure ethical practices and user satisfaction.
Applications of AI Recommendations
AI recommendations are not merely a modern novelty; they are a pivotal force shaping decision-making processes across various industries. By tailoring suggestions to individual needs and preferences, these systems enhance user experience, drive engagement, and ultimately influence consumer behavior. Understanding the applications of AI in distinct domains highlights not only their importance but also the nuanced benefits and considerations that come with them.
E-Commerce and Retail
In the realm of e-commerce and retail, AI-driven recommendations are like gold dust for businesses aiming to boost sales and customer loyalty. Platforms such as Amazon leverage algorithms to analyze user behavior, purchase histories, and browsing patterns to present personalized product suggestions. The result? Increased cart sizes and improved customer satisfaction.
These systems often incorporate sophisticated collaborative filtering, which identifies users with similar tastes and recommends products based on the choices of the group. This approach not only enhances the shopping experience but helps retailers move inventory more efficiently.
Some key benefits include:
- Increased conversion rates: Personalized recommendations can lead to a higher probability of purchases.
- Improved customer retention: When customers feel understood, they are more likely to return.
- Reduced return rates: Thoughtful recommendations can help users make more informed choices, reducing the likelihood of returns.
"In the e-commerce sphere, personalization feels less like marketing and more like a conversation between the brand and the consumer."
Media and Content Streaming
AI recommendations in media and content streaming are revolutionizing how we consume entertainment. Services like Netflix and Spotify utilize complex algorithms to curate playlists and recommend shows and movies tailored to the individual viewer's taste. This not only keeps users engaged longer but also allows companies to retain subscribers in an ever-competitive market.
The appeal lies in the ability to deliver content that resonates with each unique user. By analyzing user interactionsβlike what shows users binge-watch or skipβthese platforms can refine their suggestions continuously, creating a feedback loop that enhances the user's experience.
Considerations include:
- Enhanced user engagement: Users are more likely to spend time on platforms that offer tailored content.
- Diverse content exposure: Recommendations can push users towards genres or artists they might not have considered otherwise.
Social Media Platforms
Social media platforms are another area where AI recommendations have found a sturdy foothold, influencing everything from friendship suggestions to advertisements. Facebook, for example, employs recommendation algorithms to surface posts and content relevant to users, enriching their online experience.
These recommendations are crucial for sustaining user activity and driving interaction within the platform. By understanding user interests and social connections, platforms can create a more engaging community environment.
In this context, the advantages include:
- Fostering community engagement: Relevant content keeps users returning to the platform.
- Monetization opportunities: Stronger user engagement can lead to increased ad revenue for platforms.
Healthcare and Wellness
The application of AI recommendations in healthcare is perhaps the most heartening of all. By leveraging data from patient histories and current health trends, AI systems can suggest tailored treatment plans and wellness strategies. Apps that monitor fitness and nutrition benefit from this, offering personalized advice based on individual progress.
For instance, platforms like MyFitnessPal use AI to recommend meal plans that align with user goals. This type of personalized approach can lead to better health outcomes and increased adherence to wellness programs, making it a win-win for users and healthcare providers alike.
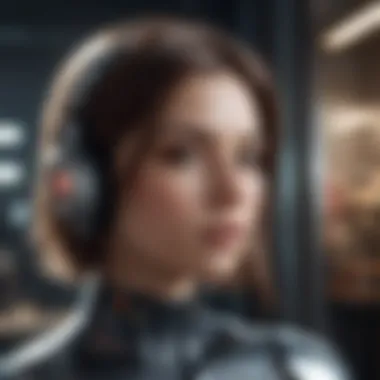
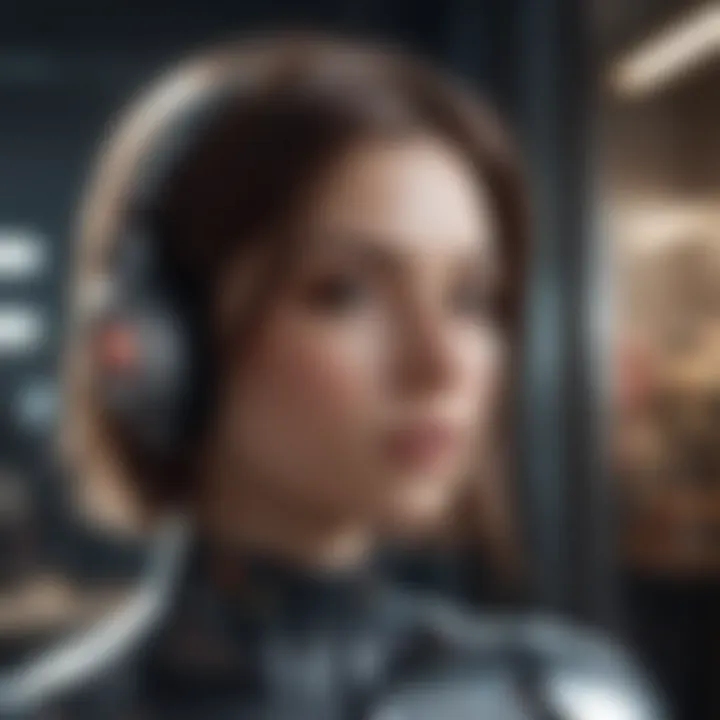
Some notable benefits include:
- Improved patient adherence: Recommendations that feel personalized can boost ongoing treatment compliance.
- Enhanced preventive care: Tailored suggestions can lead to more proactive health management, potentially lowering healthcare costs.
Challenges in Developing AI Recommendations
The landscape of AI recommendations is ripe with promise, yet it also conceals a myriad of challenges that developers and users must grapple with. Addressing these hurdles is crucial, not only for enhancing the efficacy of recommendation systems but also for fostering trust and acceptance among users. When we look at the entire ecosystem of AI-based suggestions, we must pay particular attention to data privacy, bias and fairness, and algorithm transparency. Each of these elements carries significant implications, shaping how recommendations are perceived and received across various sectors.
Data Privacy Concerns
With the rise of AI recommendations, data privacy has emerged as a cornerstone issue that cannot be brushed aside. In todayβs digital world, where data is the new gold, users are increasingly wary of how their information is collected and utilized. The operational backbone of recommendation systems heavily relies on vast amounts of personal data, from purchase history in e-commerce to viewing habits on streaming platforms. This creates a tension between improved personalization and the pervasive risk of compromising individuals' privacy.
To confront this, companies must prioritize robust data protection measures and be transparent about their data usage policies. For instance, providing users with control over their data and the option to opt-out of data collection can bolster user confidence. If users know their preferences are respected, they're more likely to engage positively with AI recommendations.
Bias and Fairness
Another significant challenge lies in the realm of bias in AI recommendations. Algorithms can unwittingly propagate existing societal biases if they're trained on skewed datasets. For example, if a recommendation system for job postings mostly uses data from a homogenous demographic, it may miss out on suggesting opportunities to candidates from diverse backgrounds. This not only perpetuates a lack of inclusivity but can also lead to dissatisfaction and disengagement from the platform.
To address these issues, developers are called upon to implement fairness-aware algorithms that actively mitigate bias. Itβs not just about creating a model that performs well statistically; itβs about ensuring that it serves all segments of society equitably. This means incorporating varied datasets that reflect diverse user profiles during the training phase, thus fostering a more inclusive recommendation process.
Algorithm Transparency
Finally, algorithm transparency is an essential piece of the puzzle. Users are rightfully curious about how algorithms arrive at specific recommendations. This curiosity can affect trust; when users feel left in the dark, they may grow suspicious of the technology.
Issues of explainability become pertinent here. Simple recommendations are one thing, yet when algorithms become more deep and complicated, explainability often falls by the wayside. Users engage more positively if they understand the reasoning behind their tailored suggestions. For instance, if a streaming service recommends a movie based on previously watched genres, explaining the rationale behind the choice can enhance the user's experience significantly.
"Transparency enables users not only to better understand the system but also to develop a sense of ownership over their data and preferences."
To foster greater transparency, developers might consider integrating tools that provide feedback on recommendations, thereby making the process more user-centered. By expanding visibility and promoting a clearer understanding of how algorithms operate, trust can blossom amidst skepticism.
The Future of AI Recommendations
The realm of AI recommendations is evolving at a breakneck pace, enveloping various sectors with the promise of more tailored experiences. Understanding the trajectory of these intelligent suggestions holds significant value, not only for developers but also for end-users who interact with these systems daily. As we navigate through the multitude of possibilities, it becomes paramount to consider the underlying trends, technological advancements, and broader societal effects that will shape how recommendations will function in the future.
Emerging Trends
The landscape of AI recommendations is not static. It is continuously shifting, influenced by numerous emerging trends that promise to redefine user experiences. One significant trend is the rise of context-aware recommendations. These suggestions take into account not just the user's past behavior but also situational data such as location, time, and even current mood. For instance, a music streaming service might suggest upbeat songs if it's morning and the user is at the gym, shifting to calming tracks when they return home after a long day.
Another trend is the growing emphasis on sustainability and ethical consumption. As consumers become more mindful of their purchases, recommendation systems will need to adapt to emphasize eco-friendly products. For example, an e-commerce platform could integrate filters that highlight sustainably sourced items tailored to a user's preferences. Companies ignoring this cue might find themselves left in the dust.
Integration with Emerging Technologies
The integration of AI recommendation systems with other emerging technologies presents a wealth of opportunities. Take augmented reality (AR), for instance. Retailers can employ AR to showcase product suggestions based on a userβs virtual try-on experience. Imagine shopping for clothes and the AR tool suggesting outfits that match your skin tone and previous preferences. This blending of AI with AR offers a seamless, immersive shopping experience that can significantly enhance user satisfaction.
Moreover, blockchain technology can bring about transparency in recommendation processes. Users could opt to view not just the recommendations but also the data and algorithms behind them. This level of transparency can build trust with users, allowing them to make informed decisions about the products and services they choose based on AI input.
Societal Implications
With the increasing pervasiveness of AI recommendations, understanding their societal implications becomes crucial. As these systems become smarter, issues of bias and equity in their algorithms must be addressed. The potential for reinforcing existing stereotypes or preferences is a concern that developers need to tackle head-on. To foster a more inclusive environment, itβs essential to ensure that the training data for AI models reflects diverse populations and perspectives.
Furthermore, the psychological impact of becoming overly reliant on AI recommendations canβt be overlooked. People habitual to relying on these suggestions might begin to feel less empowered in their decision-making. Thus, providing a balance between AI guidance and personal agency is vital. Users should feel that although they have access to insightful recommendations, they still possess autonomy over their choices.
"As we look to the future, the challenge lies in ensuring AI recommendations enhance user experience and foster a fair digital landscape."
End and Reflections
The exploration of AI recommendations has laid bare their profound influence across numerous domains. The concluding section serves as a critical juncture to unpack the insights gathered throughout the article. It centers on the intricate web of factors that have been discussed, highlighting the relevance of AI recommendations in our daily lives.
An evident takeaway is the balance AI must strike between personalization and privacy. Users today not only seek tailored suggestions but also demand assurance that their data is utilized responsibly. This becomes increasingly pivotal in fields such as healthcare, where sensitive information is at stake. The responsibility lies with developers and organizations to implement robust ethical standards, ensuring that the algorithms designed do not infringe on individual rights.
Moreover, reflections on the limitations and challenges faced while integrating these technologies are essential. For every advantage offered by AI recommendations, be it enhanced user experiences or increased efficiency, there is a counterbalance in the form of biases inherent in the algorithms. The discussion surrounding bias and fairness points to the necessity for ongoing vigilance. As technology evolves, so must our approaches toward ensuring equitable representation, especially in recommendation outcomes.
Summarizing Key Insights
- AI recommendations are ubiquitous: From e-commerce to streaming platforms, the integration of recommendation systems is evident. They shape our decisions daily, often without us even realizing it.
- Ethical implications are paramount: The shift towards an AI-driven environment leads to significant conversations about user rights and algorithmic transparency. Trust in these systems is crucial for their continued adoption.
- Technical challenges persist: Developers face a myriad of challenges including data privacy and biases within recommendation algorithms. Continuing education and adaptation in the approach to building these systems are vital.
- Future trends to anticipate: As we glance toward the future, trends such as increasingly advanced machine learning techniques and the potential for improved integration with various technologies emerge. Keeping an eye on developments in AI can prepare us to navigate this landscape more adeptly.
Encouraging Critical Thinking
Encouraging critical thinking around AI recommendations is imperative for students, researchers, and professionals alike. Here are a few considerations to ponder:
- How might widespread reliance on AI recommendations stifle originality and independent decision-making?
- What frameworks can be established to ensure ethical standards in algorithm design?
- How can community engagement in discussions about AI biases be fostered to cultivate a more informed public?
By posing these questions, stakeholders can engage in meaningful dialogues that further the understanding of AI recommendation systems. Itβs not merely about technology, but about the societal implications of how our choices are influenced and shaped by AI.