Comprehensive Insights into Stock Pattern Recognition
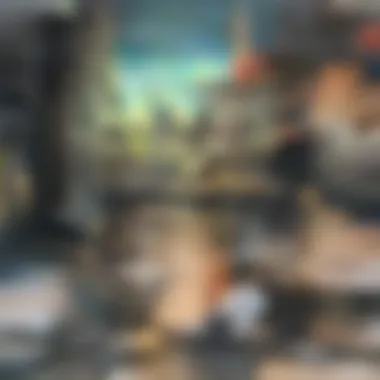
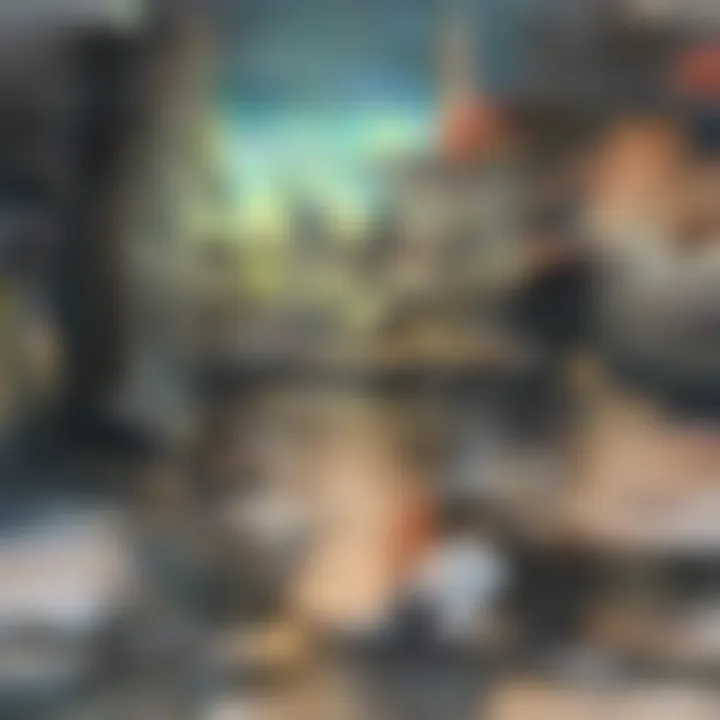
Intro
The landscape of financial markets is continuously evolving, and the role of technology has become paramount. One of the significant advancements in this field is stock pattern recognition software. This software plays a critical role in analyzing historical trends and patterns in stock prices. By utilizing complex algorithms and machine learning techniques, it helps users make informed trading decisions.
Understanding stock pattern recognition software goes beyond mere awareness. It involves an exploration of its architecture, the technology that powers it, and its applications in financial markets. This article aims to provide insights into these facets, along with evaluation metrics and future trends. The goal is to equip researchers and practitioners with knowledge that enhances trading strategies and market predictions.
The relevance of stock pattern recognition software cannot be overstated. As data becomes more extensive and complex, utilizing effective tools to analyze this data is crucial. The ability to recognize patterns can lead to improved trading outcomes and risk management.
Through this exploration, stakeholders will gain a solid basis for understanding how to leverage stock pattern recognition software to navigate the complexities of financial data analysis. With a focus on algorithms, user interfaces, and the implications of machine learning, this article serves as a valuable guide.
Foreword to Stock Pattern Recognition Software
In the ever-evolving financial landscape, stock pattern recognition software serves as a critical tool for market analysis and investment decision-making. Understanding stock patterns allows traders and investors to identify potential opportunities and make informed decisions based on historical data trends. The importance of this technology cannot be overstated; it encompasses not only the identification of patterns but also the statistical methods and algorithms that underpin these systems.
Definition and Overview
Stock pattern recognition software is designed to detect and analyze price patterns in stock market data. It uses algorithms that process historical price movements, volume, and other relevant metrics to discern recurring patterns. These patterns can indicate potential buy or sell signals, assisting traders in their strategy development.
The software typically integrates with various data sources, providing real-time data analysis and visualization tools that enhance decision-making processes. Users can customize how they view this data, making it adaptable to individual trading strategies.
Historical Context
The concept of stock pattern recognition has roots in technical analysis. Historically, traders have studied charts and patterns for centuries to predict future price movements. The rise of technology in the late 20th century transformed this process. Early systems relied on manual analysis, which was time-consuming and prone to human errors.
As computational power increased, algorithms were developed to automate the recognition of stock patterns. The introduction of machine learning and artificial intelligence has further revolutionized this field, enabling software to learn from new data inputs and improve its predictive accuracy over time.
The evolution of stock pattern recognition software reflects a broader trend towards data-driven decision-making in the finance sector. Today, both retail and institutional investors rely on these sophisticated tools to enhance their market analyses and trading strategies.
Key Features of Stock Pattern Recognition Software
Understanding the key features of stock pattern recognition software is essential for maximizing its utility in financial analysis. These features determine the softwareโs effectiveness in identifying market trends and assisting traders in decision-making. When evaluating such tools, specific elements can greatly affect performance, user experience, and overall benefits.
Algorithm Types
The core of stock pattern recognition software lies in its algorithms. Each algorithm type incorporates different methodologies to analyze stock data. For example, some software employs technical analysis algorithms which focus on historical price movements. Others might utilize machine learning algorithms that adapt based on incoming data, helping to identify more nuanced patterns. Algorithms can range from simple moving averages to complex neural networks. Understanding the strengths and weaknesses of these algorithms is crucial. It helps a user select software that aligns with their trading strategies and goals.
User Interface Considerations
An intuitive user interface is vital for effective stock pattern recognition software. The experience of analysts and traders can significantly be overall improved through clear navigation and responsiveness. Users benefit from features like customizable dashboards, which allow them to concentrate on the most relevant data. Good visualization tools can also enhance the interpretation of patterns. Additionally, accessibility on various devices, such as tablets and smartphones, can make analysis more flexible. Ensuring the interface can cater to skill levels from novice to expert can enhance user engagement and productivity.
Data Integration
Data integration is a critical feature in stock pattern recognition software. The ability to aggregate data from different sources enhances the robustness of analysis. This means integrating real-time market data, historical data, and other relevant financial indicators into a unified system. Those who wish to utilize software effectively should favor systems that support API integration for seamless data pulls. Integration with third-party platforms can broaden the analytical capabilities and provide deeper insights into market movements. Users should also consider how well the software handles various data formats to enhance accuracy in processing information.
"The effectiveness of stock pattern recognition is often enhanced by the quality and diversity of input data."
How Does Stock Pattern Recognition Work?
Understanding how stock pattern recognition works is essential for anyone involved in finance, whether they are individual investors or institutional traders. This section aims to dissect the underlying components that contribute to the functionality of stock pattern recognition software. By exploring specific elements such as data sources, processing techniques, and pattern detection algorithms, readers can gain insights into the advantages and considerations when utilizing this technology. The ability to analyze patterns in stock data can inform trading decisions, enhance market predictions, and provide a competitive edge in the financial landscape.
Data Sources
The foundation of effective stock pattern recognition lies in the quality and variety of data sources. Typically, stock pattern recognition software pulls information from multiple datasets, including historical price data, volume statistics, and market indicators. These datasets may come from various financial markets, including stocks, ETFs, and indices.
Common data sources include:
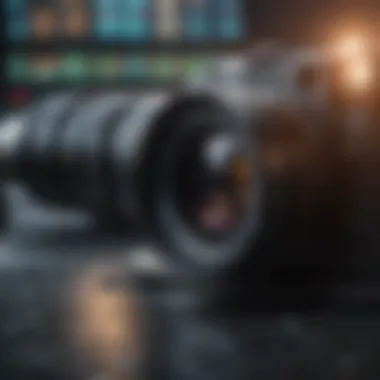
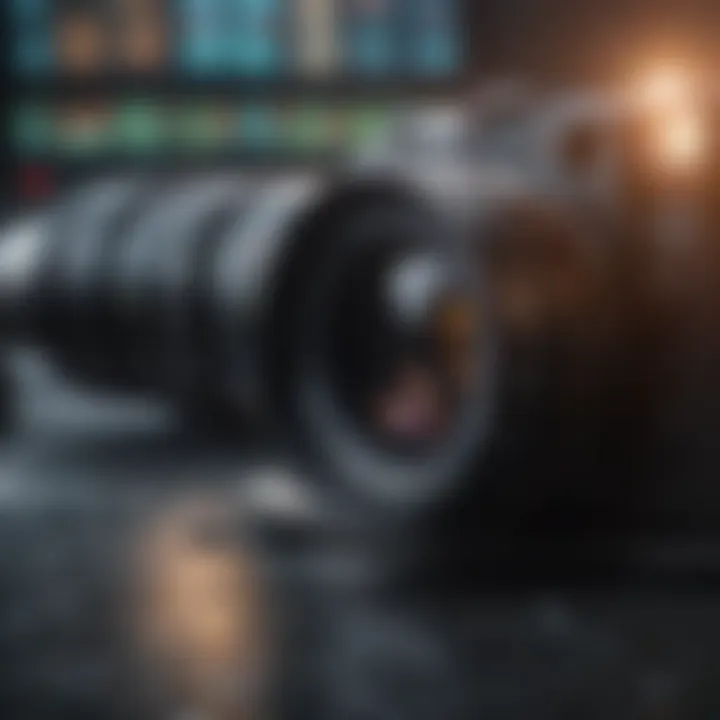
- Market Exchanges: Data from stock exchanges like NYSE and NASDAQ.
- Financial News APIs: News articles can influence stock movements, and APIs from sources such as Bloomberg or Yahoo Finance can be integrated.
- Social Media: Platforms like Twitter can provide sentiment analysis data.
Having access to diverse data sources allows for a comprehensive analysis. This is crucial as different stocks or markets may behave differently under varying conditions. It can also help to mitigate the impact of data inconsistencies. The more reliable data a trader can use, the better-informed their decisions ultimately are.
Processing Techniques
Once the relevant data is gathered, specific processing techniques must be applied to transform raw datasets into actionable insights. This stage is pivotal because it determines the accuracy and efficiency of pattern recognition. Common techniques include:
- Data Cleaning: Removing inconsistencies, null values, or outliers in data.
- Normalization: Adjusting the scales of different datasets to create a uniform benchmark for comparison.
- Time-Series Analysis: Methodologies that focus on analyzing data points collected or recorded at specific time intervals.
These processing techniques help to ensure that the pattern recognition algorithms work with precise and reliable input. A well-structured dataset can significantly improve the detection of meaningful patterns, thus enhancing the software's overall effectiveness.
Pattern Detection Algorithms
At the core of stock pattern recognition software are the algorithms designed to detect patterns in the processed data. These algorithms can range from simple moving averages to complex machine learning models. Examples include:
- Moving Average Convergence Divergence (MACD): A trend-following momentum indicator that shows the relationship between two moving averages.
- Support and Resistance Levels: Algorithms to identify price levels where stocks tend to reverse direction.
- Neural Networks: Advanced techniques that learn and improve from the data over time, becoming adept at recognizing intricate patterns.
Pattern detection algorithms play a critical role in the software's success. The right algorithm can lead to better trading signals and improved decision-making. However, users must also be wary of the limitations inherent in each algorithm. Factors such as market turbulence or sudden news events can distort patterns and lead to erroneous predictions.
"The efficacy of stock pattern recognition software depends fundamentally on the strength of its algorithms and the quality of the data inputted."
Applications in Financial Markets
The relevance of stock pattern recognition software in analyzing financial markets cannot be understated. As financial markets evolve, the complexity and volume of data require innovative solutions for effective analysis. Pattern recognition software serves as a vital tool for traders, analysts, and investors, aiding them in making informed decisions. The application of this technology spans various areas including market analysis, trading strategies, and risk management, making it indispensable in today's trading environment.
Market Analysis Methods
Market analysis methods involve evaluating market trends, price movements, and trading volumes to gain insights into potential future activities. Stock pattern recognition software enhances these methods by automating data analysis.
- Trend Analysis: By identifying prevailing market trends, this software helps traders understand market direction, whether bullish or bearish.
- Technical Indicators: The software integrates various technical indicators, such as moving averages and relative strength index, which provide additional insights into market conditions.
- Signal Generation: It generates actionable signals based on historical data patterns, which can alert traders to potential buy or sell opportunities.
Collectively, these approaches empower traders to interpret complex data, leading to more strategic decisions.
Trading Strategy Development
Developing a robust trading strategy is critical for success in financial markets. Pattern recognition software plays a significant role in crafting these strategies.
- Backtesting Strategy: New trading strategies can be tested against historical data to assess their viability before actual implementation. This reduces the risk and enhances strategy refinement.
- Real-Time Analytics: Software provides real-time analysis which allows traders to adapt strategies promptly in response to market changes.
- Customization: Users can tailor strategies specific to their investment goals and risk tolerance, making the software suitable for various trading styles.
By leveraging these capabilities, traders can improve their performance, ultimately leading to better returns.
Risk Management Techniques
Effective risk management is crucial in trading. Stock pattern recognition software offers several features to identify and mitigate risks.
- Volatility Assessment: The software can analyze market volatility and help investors understand the potential risks involved in their trades. This is essential for making well-informed decisions while investing.
- Stop-Loss and Take-Profit Levels: It assists in setting appropriate stop-loss and take-profit levels based on market patterns, thereby protecting capital.
- Diversification Strategies: The software can analyze correlation among various securities, guiding users on how to best diversify their portfolios to manage risk effectively.
Such techniques play a fundamental role in safeguarding investments and enhancing overall market performance.
"Understanding and applying stock pattern recognition effectively will fundamentally shift the way one engages with the financial markets."
Evaluating Stock Pattern Recognition Software
Evaluating stock pattern recognition software is crucial for investors and traders who aim to navigate the complexities of financial markets. The evaluation process helps identify which software solutions provide the best tools for analyzing market patterns and developing effective trading strategies. By understanding the key evaluation metrics, backtesting processes, and user feedback, stakeholders can make informed decisions that enhance their trading performance and reduce risks.
Performance Metrics
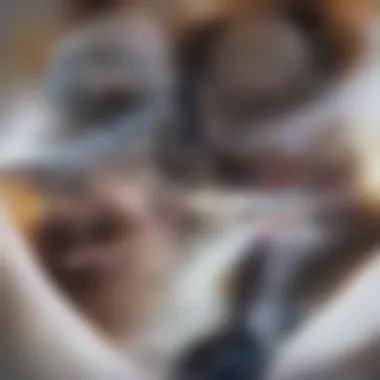
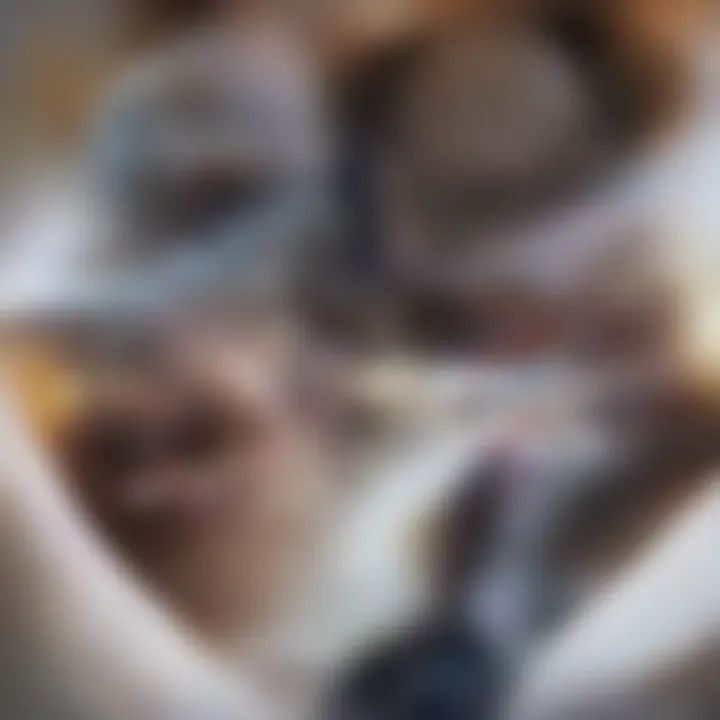
Performance metrics serve as benchmarks to assess the effectiveness of stock pattern recognition software. Key metrics to consider include:
- Accuracy Rate: This indicates how often the software correctly identifies patterns in historical data. A higher accuracy rate suggests more reliable predictions.
- Speed: Speed of data processing and pattern recognition is critical, especially in fast-paced markets. Efficient software should provide real-time analysis.
- Usability: The software should be intuitive and user-friendly. A steep learning curve can hinder users from fully leveraging its capabilities.
- Integration Capabilities: The ability to integrate with other trading platforms and data sources enhances the software's functionality.
"Understanding performance metrics is essential in evaluating the reliability and efficiency of stock pattern recognition software, allowing investors to trust their chosen tool."
The right combination of these performance metrics will directly impact trading success and strategy development.
Backtesting Methodologies
Backtesting is a critical component in the evaluation of stock pattern recognition software. It involves testing the softwareโs algorithms against historical data to determine how well they would have performed in real trading conditions. Several methodologies exist in this space:
- Historical Simulation: This method uses historical price data to identify how the softwareโs predictions would have fared in the past. It provides a solid foundation for evaluating potential outcomes.
- Walk-Forward Analysis: This technique divides historical data into segments, using earlier segments for backtesting and the later segments for validating results. It allows for adaptive model evaluation by emulating pseudo future trading conditions.
- Monte Carlo Simulation: This is a stochastic technique that assesses risk and uncertainty by simulating a wide range of possible market scenarios. The results can highlight how various factors may affect performance over time.
Effectively utilizing backtesting methodologies permits a clearer understanding of how reliable and robust a softwareโs prediction algorithms truly are.
User Feedback and Reviews
Genuine user feedback and reviews provide invaluable insights into the functionality and practicality of stock pattern recognition software. Assessing reviews highlights:
- Real-World Usability: Users share their experiences, showcasing strengths and weaknesses that may not be evident through formal documentation.
- Support and Community: Active user communities often offer advice, support, and share best practices, which can enhance the overall experience with the software.
- Updates and Maintenance: Regular updates based on user feedback can indicate a companyโs commitment to improving its software.
Overall, examining user feedback helps potential users gauge the quality of the software before making any investment. Positive reviews can reinforce confidence while negative reviews may signal potential pitfalls.
This systematic approach allows users to critically evaluate stock pattern recognition software, ensuring they select a solution that aligns with their trading goals and methodologies.
Challenges in Stock Pattern Recognition
Understanding the challenges associated with stock pattern recognition is crucial for both developers and users of this software. Recognizing these challenges allows for better implementation and utilization of the software in real-world trading. Acknowledging data quality issues, market volatility effects, and algorithm limitations will enhance the overall efficacy and reliability of the software. This deeper understanding can lead to improved predictive capabilities and informed decision-making in the financial markets.
Data Quality Issues
Data quality stands out as one of the primary hurdles in stock pattern recognition. The effectiveness of pattern recognition software heavily depends on the quality and accuracy of the data fed into its algorithms. Poor data can lead to misleading signals and inaccurate predictions. Issues such as missing data, incorrect price points, and outdated information can distort the outputs from these systems.
Investors must ensure that they use data from reputable sources. Some common sources include financial market databases, stock exchanges, and financial news platforms. It's essential to perform regular checks and clean the data set to maintain its integrity. Additionally, software that lacks robust data handling capabilities may exacerbate these issues.
Market Volatility Impact
Market volatility also poses significant challenges for stock pattern recognition. Markets can experience rapid fluctuations due to various factors like economic announcements, geopolitical events, or changes in market sentiment. Such volatility can lead to abrupt changes in stock price movements, making it challenging for algorithms to accurately identify patterns.
For software users, this means that a strategy based solely on historical patterns might not perform well in highly volatile conditions. Thus, it is important to incorporate market volatility considerations into trading strategies. Recognizing the dynamic nature of the markets is vital.
Algorithm Limitations
Lastly, it is essential to consider the limitations of the algorithms used in pattern recognition. While many algorithms are designed to detect trends and patterns, they often rely on historical data and may struggle with novel situations. This limitation can result in a failure to adapt to new market dynamics or unique events, which can lead to erroneous conclusions.
Furthermore, the complexity of certain algorithms can lead to issues such as overfitting, where the model performs well on training data but poorly on unseen data. This can diminish the model's utility in real-time trading scenarios. Investors should be aware of these algorithmic constraints and take them into account when evaluating the softwareโs performance.
"A critical examination of these challenges is necessary for any investor aiming to use stock pattern recognition software effectively."
By understanding these challenges, users of stock pattern recognition software can approach their trading strategies with more awareness. They can incorporate better data practices, be mindful of market conditions, and choose algorithms that are more aligned with their investment strategies.
Future Trends in Stock Pattern Recognition Technology
The future of stock pattern recognition software is shaping up to be make a significant impact in trading and investment strategies. As technology continues to advance, several key trends are emerging that professionals in the field should closely observe. The integration of artificial intelligence, the rise of big data analytics, and the incorporation of blockchain technology for data security are all important elements that can enhance the capabilities and reliability of stock pattern recognition software.
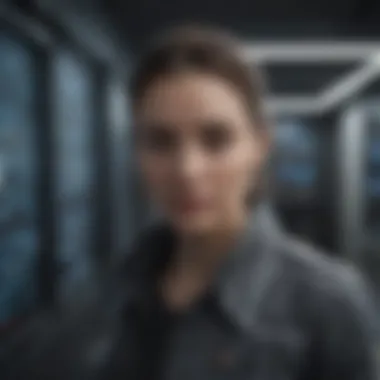
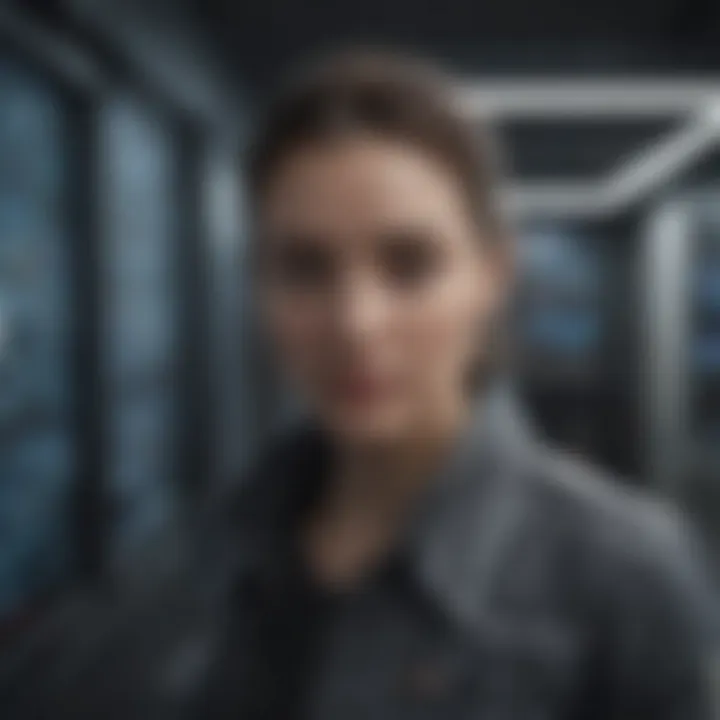
Integration of AI and Machine Learning
AI and machine learning are transforming stock pattern recognition by providing more precise and adaptable algorithms for predictive analysis. These technologies enable software to learn from historical data, improving its ability to identify patterns over time. As algorithms evolve, they become adept at not just identifying past trends but also predicting future market movements with greater accuracy.
- Personalization: AI can tailor trading strategies based on an individualโs investment preferences and risk tolerance.
- Automation: Many tasks can be automated, saving time and reducing human error in analyses.
The growing reliance on AI means that traders need to be familiar with how these systems work.
Advent of Big Data Analytics
Big data is now a cornerstone of financial analysis, offering vast amounts of information for recognition and forecasting. Stock pattern recognition software can harness this data to extract actionable insights which can influence trading decisions.
- Enhanced Analysis: Access to larger datasets allows for sophisticated analyses that may have been unfeasible in the past.
- Contextual Understanding: Evaluating stocks based on extensive market data lets software understand context better, leading to improved decision-making.
The capacity to analyze diverse datasets from various sources continues to grow, leading to more informed trading tactics.
Role of Blockchain in Data Security
As stock pattern recognition software becomes more embedded in financial systems, data security must be prioritized. Blockchain technology presents a promising solution by offering a decentralized method for securing data. It ensures that the information remains transparent and tamper-proof.
- Immutable Records: Data integrity is crucial, especially when dealing with sensitive financial information. Blockchain creates unchangeable records, enhancing trust.
- Streamlined Transactions: By utilizing blockchain, transactions can be processed more efficiently while maintaining security, which is vital in high-speed trading environments.
"The evolution of stock pattern recognition software is a testament to the accelerating pace of technology in finance."
Understanding these trends is essential not just for keeping competitive but also for leveraging the full potential of financial tools in an ever-changing market.
Finale
Understanding stock pattern recognition software is essential for both investors and researchers in finance. This technology provides tools that enable users to analyze vast amounts of market data to identify patterns and trends. The implications of this analysis are far-reaching in the context of trading strategies and risk management. By utilizing advanced algorithms and machine learning techniques, investors can gain an edge in the highly competitive financial markets.
Summary of Key Points
Throughout this article, we have explored several critical areas of stock pattern recognition software:
- Definition and Overview: Established the foundational understanding of what stock pattern recognition software is and its evolution.
- Key Features: Discussed the various algorithms, user interface comprehensibility, and the importance of data integration.
- Functionality: Clarified how inputs and processing techniques contribute to effective pattern detection.
- Applications: Highlighted how market analysis, strategy development, and risk management utilize this technology.
- Evaluation Metrics: Focused on performance metrics, backtesting methodologies, and the role of user feedback.
- Challenges and Trends: Addressed common problems and examined future trends including AI integration, big data analytics, and blockchain's role.
Implications for Investors and Researchers
For investors, stock pattern recognition software can significantly enhance decision-making processes, leading to better-informed trading practices. Investors can process complex data more efficiently, utilizing insights to navigate volatility and optimize portfolios. For researchers, understanding the technical and practical aspects of this software fosters innovation in financial modeling and data analysis methodologies. It opens avenues for further studies into algorithm performance and real-time data processing capabilities.
Final Thoughts
The landscape of stock trading is continuously changing. As technology evolves, the capabilities of stock pattern recognition software will undoubtedly expand. Investors and researchers alike must stay informed. By keeping abreast of trends and emerging technologies, stakeholders can capitalize on new opportunities and mitigate risks effectively. In an age where rapid data analysis can make a difference, embracing these tools will likely shape the future of financial markets.
Further Reading and Resources
In the realm of stock pattern recognition software, further reading and resources play a vital role. They offer insights that deepen understanding and application of concepts discussed in this article. For students, researchers, and professionals alike, additional materials can illuminate advanced techniques and foster critical thinking about software utility in the financial sector.
Academic Journals
Engaging with academic journals is essential for thorough comprehension of stock pattern recognition software. Journals often publish peer-reviewed articles that analyze the latest findings and methodologies. This body of work presents cutting-edge research that can enhance your knowledge and application of software tools. Notable journals in this domain include the "Journal of Financial Markets" and the "Journal of Trading." These journals can provide rigorously conducted studies and case analyses. Consequently, by referencing these materials, one can better appreciate how algorithms function in real-world contexts and their implications for trading strategies.
Online Courses and Tutorials
Online courses and tutorials represent another beneficial avenue for enhancing expertise in stock pattern recognition software. Many platforms, such as Coursera and Udemy, offer focused courses that delve into specific aspects of stock analysis, algorithm development, and software applications. These resources allow learners to acquire skills at their own pace and revisit complex subjects. Engaging in well-structured tutorials can also foster practical understanding, making it easier to implement learned concepts in trading environments.
Research Studies and White Papers
Research studies and white papers add depth to oneโs understanding of stock pattern recognition. They often encompass detailed examinations of software efficacy, performance metrics, and the actionable insights they provide. White papers from reputable sources detail advancements in technology and provide empirical data concerning software performance. Moreover, platforms like arXiv or SSRN are excellent for finding cutting-edge research that informs ongoing trends in the finance field. This information is crucial for investors and researchers aiming to stay ahead of technological developments and improve their analytical approaches.
"Knowledge is power, and in finance, the more informed you are, the better your trading decisions can be."
Accessing these resources enables a comprehensive grasp of stock pattern recognition software, empowering users to make informed decisions while navigating financial markets.